- Home
- Intro
- Login
- Contact formular
Encrypted communication
mail@christopher-germann.de
PGP key download (4096-bit RSA algorithm): https://christopher-germann.de/0xBB5784B99E839AB1.asc-----BEGIN PGP PUBLIC KEY BLOCK----- mQINBFyXJ/EBEACh+pm/3HaoC+5BtImkkr2QTamKi2C/8yvy2Hlfr9hX6yB3FZMM EsIMpPZXwYdcZkkY5A5QFAKYBALPjlBdf0jnXEOq8DzNZWUa9ftezIpkMiec0WzV Rzobp4JraAtMqXSfVFmKetySLv+XeqBhd6zK3dWMj5GKSDVrnCz7H9hWok6s+2fw wlZ6A/ngKw+5GCjI+eOgWl9kxrYGqohGv3D+JvkzBA6G8OvEEf5Qg5Cb4qq9Sos2 /u1kUBa1DyiF3KnsWSxDe2yRMtbL8ADh9+xVMVxxc70fibKl22Jz5ZJHGjEZgv7O rDRfLA+SzfzG8yNoxutZC8sGnkDsry+LrDQqs4M8iEnTC/edUr9q/ExCwVJyHiQb AtJmTNf5scEEJ3CGKuV4KJqwV/rojWFokagZaWaUfDxxj22GBKoNV/c/N2VLW21c 5aozYX7YmEClnu2PxDLg47x2ldM57MiWfpBeEKBFb1wpRFFzTzd9OF4LoIpaeDkw icJO96AU6whFPQfAYGCRnLMcSS2vE8yvt551Ab9+8zC0ElMqhpLKg+tclHHdfvd8 PwFbRSKYRx/537abAcF6dxwNKoN6gTiGFK1KM/GdY8o6IL+0g1gug5l2hALT4sO3 MNT8BF5y24hvG4JeK0SLUKMES2WWk6A++r/nmSZu0GjfoU2ATP4tAVijcQARAQAB tClDaHJpc3RvcGhlciA8bWFpbEBjaHJpc3RvcGhlci1nZXJtYW5uLmRlPokCVAQT AQgAPhYhBBZ7mXia8KAzG20MJbtXhLmeg5qxBQJclyfxAhsjBQkJZgGABQsJCAcC BhUKCQgLAgQWAgMBAh4BAheAAAoJELtXhLmeg5qxazkQAInZiLk1BkQ9n6bs/bkR BdeM9pdXt7KEnRoqjjnY0VYRwZGoV3Ht1KNQZto990PFbNSEkb/McGeE2PpaJKgn jmxHYKv6/nDZVhHsLFPXo1ORXtyyNBt9JTdr1IX8y/v26dR69PVPipKQZhIHpVfD VVNthvmh4igN1O5l5freH74E8QBTorFncHnoHtcJGn8faYHw/Unrxqyz6ziCPsr8 ivcYr2HF5ZSQHn+UWkM2zs3CTP5kmaIrfwoLBmYxef9RPDbG6+YJaujN+6Rp4QOy SKKZHK2aZwheH2ccU558M/0++oqeYZk/r2BT3A31Qof0cVmE3vI3LkByhNwTCz4V /DhgwSF6LTda6gbTZ3VDHb/du3Y1hycpT+viwOpVAyVWb9zpWPFRqxvPVMLDjWnH 9gMafpK2/6kb26zqH4R7GKllfah9/6CNquVPO98rpbjxRaARDXVG1v2FibWBwc3S +sUqQOvEbDDw+l+3cFMvWT8Ulzt5LTJfapKaSnKg2GSjrkRVLEpfxP4x/RrxEj8k AYgmdEmfAB53V8YHbYTwZSrQ7QsHUoUOv/PpEl8b9hkg1zUeDx1PR0/ZVc0Gm+oO Hwy5/t84j5ppPakRgmIDZr1u2xSsajgVEDOKsjpIvrBBkIRGaHK62+NLHF2X6l8k 1hutXB8g4WMBmEFKRm3QP+dpuQINBFyXJ/EBEADD3izFV9NhZnzBVS9b588Twr/w WMOcGF6p2pBF513bQvg4SgVvSrf54hqKJ3MqYEiYHUsZmoM/tpgU9IO+1d/pVBoP sdG92HzDrpv6/Lj/Em6c00zv5f3iWT6kqSaB1Q49Khw+MbKc/OsBciIZPU+8J06n WGrdUGtCxt+x0DAk/zYJyGo/C7Jwkra4vSCxdP70F0oepXSZZCRPMA9j1kObmJKA M68vtHRzZJ4o9Z4CC+1Symxcmq+QaDK3n1/2mjBx5WHP0iTm3Puj9hJMCjjW/vtd LXl8A2lNUnvsgVGq2EPP1RMU2EN8tSrNnKCbCcGw4EvVDuovOSdaF0ozd37PmwJ/ x9x08/5vdm2PRBM2PUutjHWKeqL99CA0alHjmw0Fmc8W99ynjTltzlGf24lLKr9f 4R7TV4vQ9YbVvSxpwaD1Nas0Ch4CQPS63XRHcxvbPQBBYU7B+gYhdfJQVWMWNExR ytFr80G7/ngTGL0SQDzN9ZYrFQlXqTtlhjoMydM7tTATgQswQ3fdSs8dbyCadmka 0mMwqFDPLcX2/AADkvxnY3wN7RcXK/CcBfAZR7Rjq6Gktys9ZmSktWcjcPuq7p/3 l5A6pkrOdJNc5pYJimTeObM0f2tn4kXysV85jLXsvPIn0hT6O9yUh6Uyaun4p5Ta yueEbEzZ2AOv0UeA3wARAQABiQI8BBgBCAAmFiEEFnuZeJrwoDMbbQwlu1eEuZ6D mrEFAlyXJ/ECGwwFCQlmAYAACgkQu1eEuZ6DmrGodRAAjfO2Bh+sqp4B/PyouwZ2 T5LrFPcQb1XbiYiN0gvkMmXHMwEfFr05nI1XZvVKNZp8KEAmu1SbGtm6uDCYmuV1 YzcvICzeW9kgCu7gDdYcDt/2WsHcz0hZQT4ii2i979kbUkd+8QtG/katQ3aetaXK aGYP8MAuVqxlLUHvO37nFfd8ecWlyKsuPR3IW4mVPFjy4mgYdzr0Pmq3GgRJs13R CLuqTEwiQYjlwMwrgBmLzTf7blm/60e7kjFOkgIC6ZzG33bsqXQpR1rSy+WlndgU 2l8H627gv93CKqDDOaZD3V8pFptBArS+eukyhLV5lpPwYBBNlDctC2zACct4ZIJ2 /D1XBaYK++yOGiZgvaG6h5e6Fi3hTY1+1zthzCM5mbNma5vqztllk+HV5gtHK0Q0 ZowhqI7ejTvfsmMT6kP5JdOV9ZNfdTaPrME181OTDVl+WSEwMGGJEPwAGYQSc3np Gc5ShuOURvNPnBl13hGGTdMrDiBuwqJasj0u3FgmTsUSN/JLbIum0s/VHxhbkVfS CAl5yyqvmrhdBWs83NgwOSxNKrhfajZ0r+A0te6rdPk0EMns1/rnLOCsem4WyCZ4 v5/RNw8i/b4VGdzSPItUx9LC5l5zksXbYkZjgaWD9RRIzPzoMlEkEM/uagi8RXDg E+/seqODYu5vr6n0k2ETgNM= =roWh -----END PGP PUBLIC KEY BLOCK-----
- Intelligent Search Function
-
-
Intelligent Search Function
Note:
You can use Boolean operators/regular expressions to refine your search.
-
-
-
Site-search with DuckGo
(avoid Google like the devil! I know it's difficult.
It's omnipressence is part of the problem...)
-
-
- PostsSelect
-
-
Archives
-
-
- AI Translation
- Curriculum Vitæ / CV
- Marie Curie Alumni
- Presentation @ Oxford Saïd Business School (19.04.2024)Conference on value-based education
- PhD dissertation: A psychophysical investigation of quantum cognition - An interdisciplinary synthesisFull-text in HTML5
- Visualising the brain with R
- 5-Methoxy-N,N-dimethyltryptamine: An ego-dissolving endogenous neurochemical catalyst of creativityPublication
- N,N-Dimethyltryptamine: An endogenous neurotransmitter with extraordinary effectsConference Proceedings
- Bayesian a posteriori parameter estimation via Markov chain Monte Carlo simulations
- Cognitive Neuroscience Podcast on Quantum Cognition and DMTInterview
- International conferences
- The paralogisms of null hypothesis significance testing in science
- Lecture on Quantum Cognition @ CogNovo (University of Plymouth, United Kingdom)Video-lecture
- Presentation @ “Science and Nonduality” conference (California, USA)Audio-lecture
- Quantum cognition: An epistemological challenge for naïve and local realismConference abstract
- Dimensions of epistemology and ontology: A multidisciplinary dual-aspect monism perspective on psychophysics
- A manifesto for systematic interdisciplinary Psilocybin research
- CogNovo.eu
- Statistical Power & Sample Size – An interactive spatial metaphor
- Psychometric scales
- Qbism - The psychophysics of Bistable Perception
- Transpersonal neurochemistry: A novel treatment for addiction
- Interdisciplinary visions: Desert toads, shamans, and entheogens
- Meta-ethics
- Transcending academic and epistemic boundaries: Psychoactive tryptamines and the frontiers of human exploration
- Podcast with Prof. Roger Malina @ CreativeDisturbance.orgInterview
- Book reviews
- The “Brain in a vat” Gedankenexperiment
- Neuro-Juggling & Embodied Cognition
- The toxic effects of Glyphosate on the brain (neurotoxicity)
- The ‘Weapons Priming Effect’ and aggressive behaviour in children
- Aerial yoga
- Yoga āsanas
- Travels
- Onion website (only accessible via TOR browser)
- BitTorrents
- Academia
- Psychology
- Interview @ RadioCognovia
- Prāṇāyāma
- Bose-Einstein Statistics (Quantum dice)
- Web-based auditory experiment
- Secondment at Manipal University Jaipur in India
- Social/public engagement and humanistic concerns
- Interactive 3D scatterplot in OpenGL
- Misc
- Dŗg-Dŗśya-VivekaA systematic introspective psychophysical inquiry into the ultimate non-dual nature of the seers and the seen
- Yoga Sutras of Patanjali
- Post slider
- Post portfolio
- Contact
- Sitemap
- External Domains
- Data-protection (GDPR)
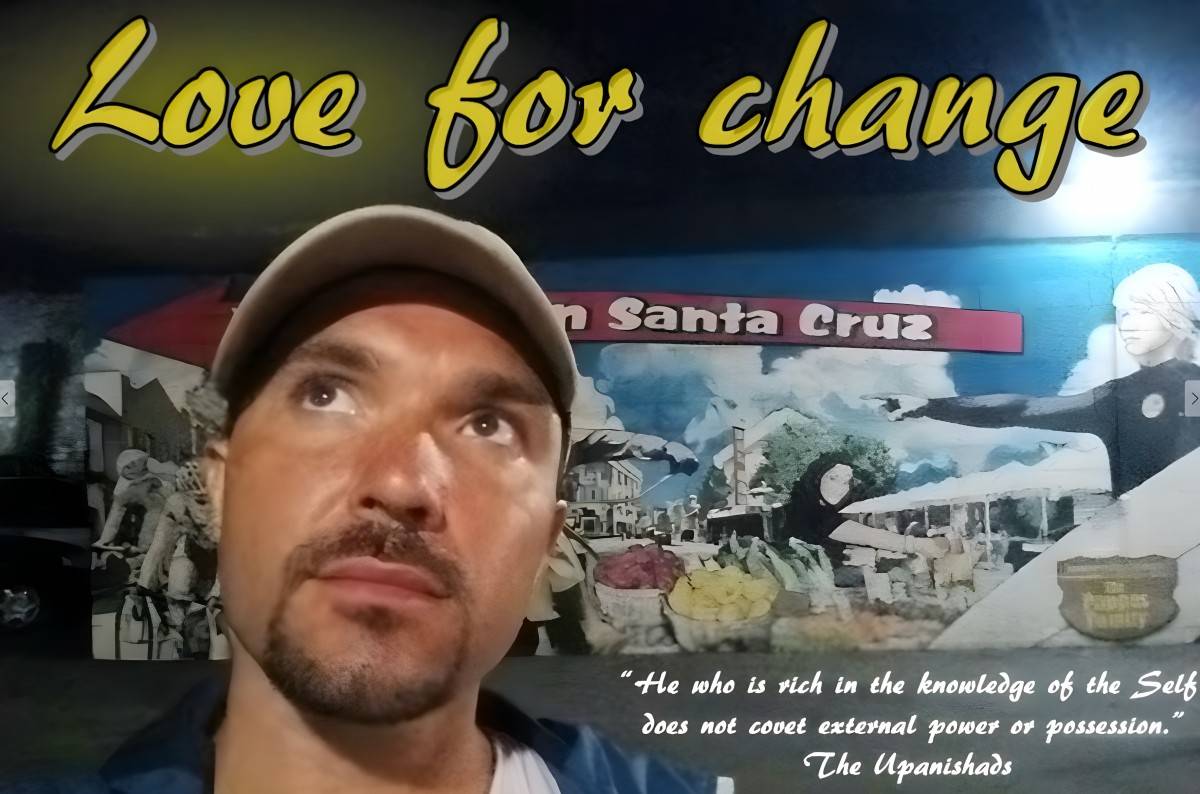
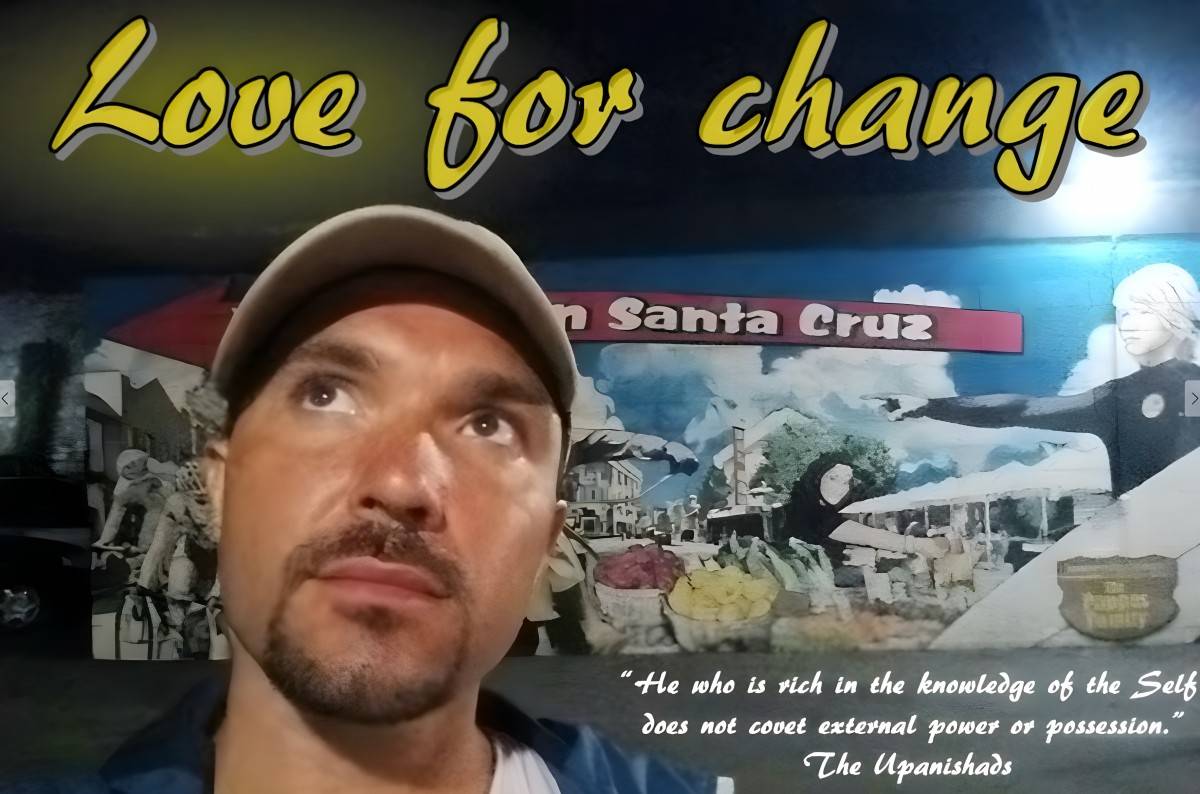
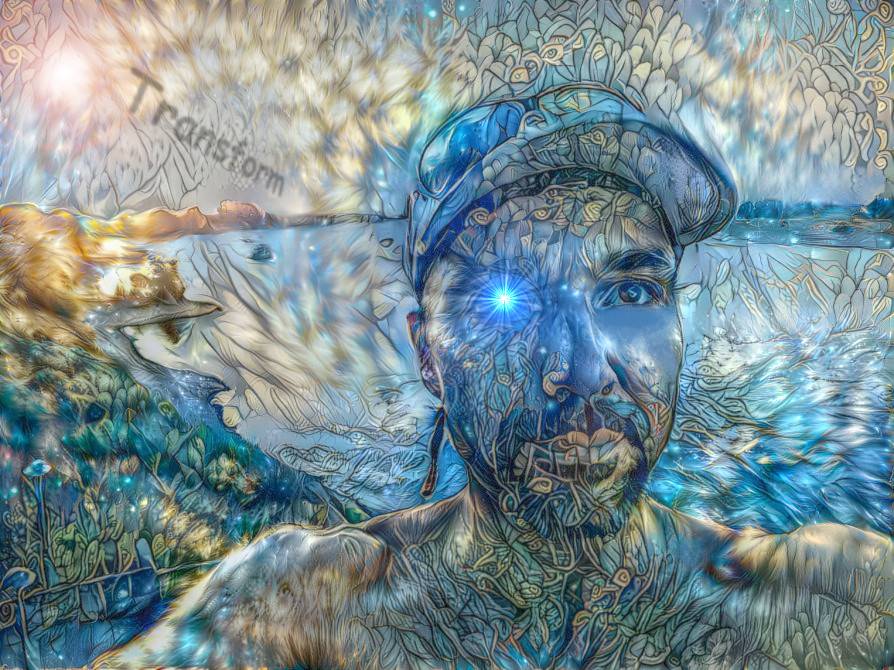
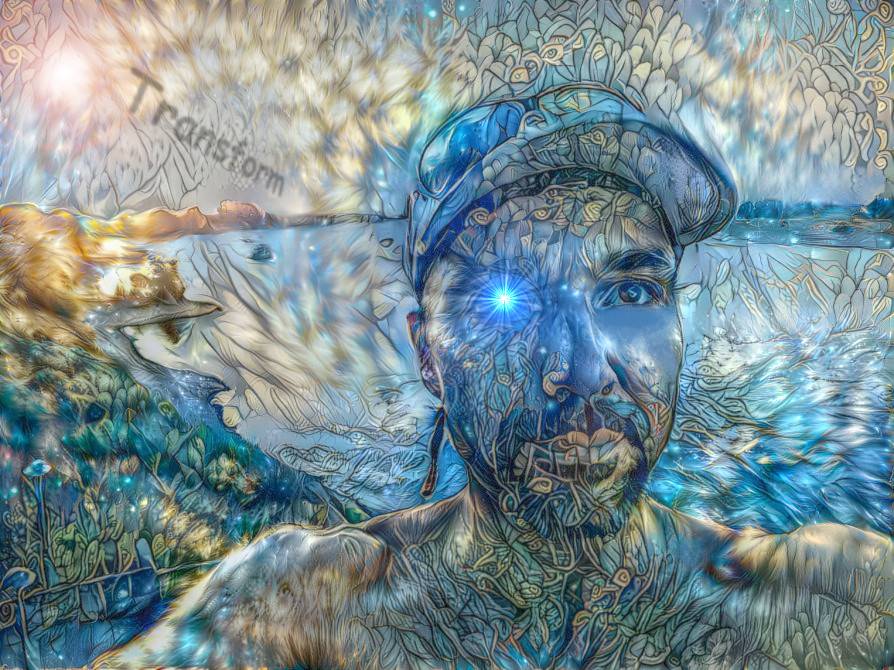
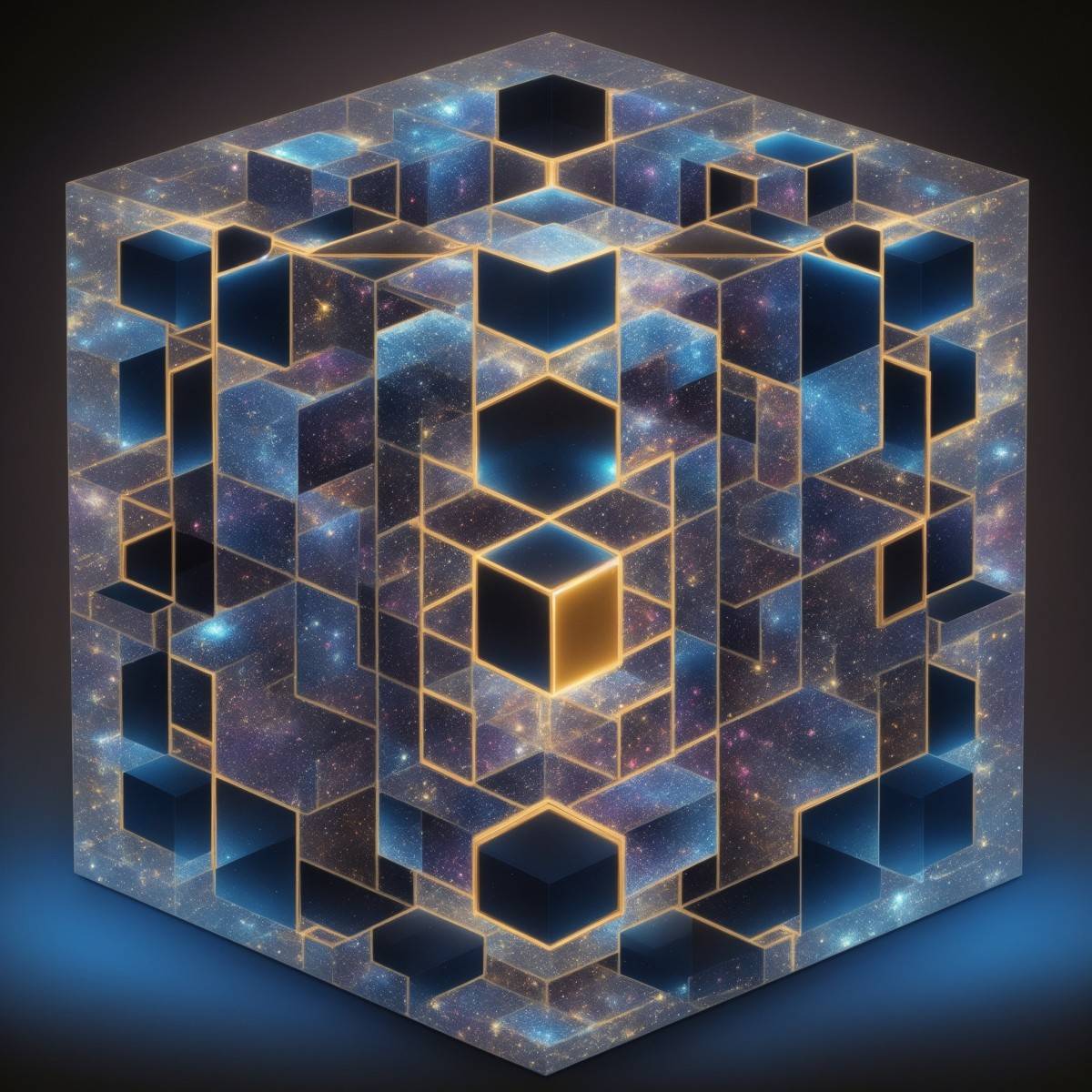
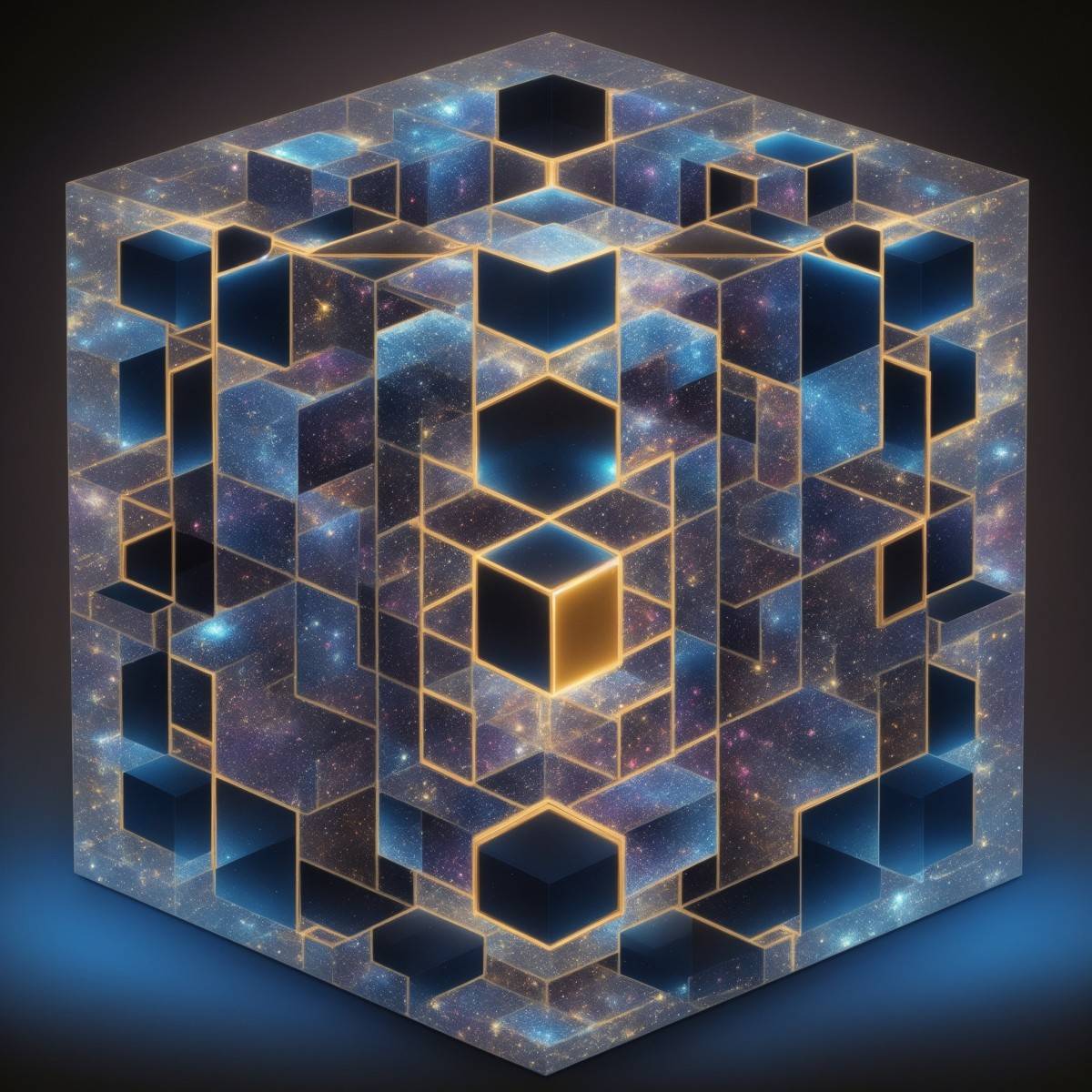
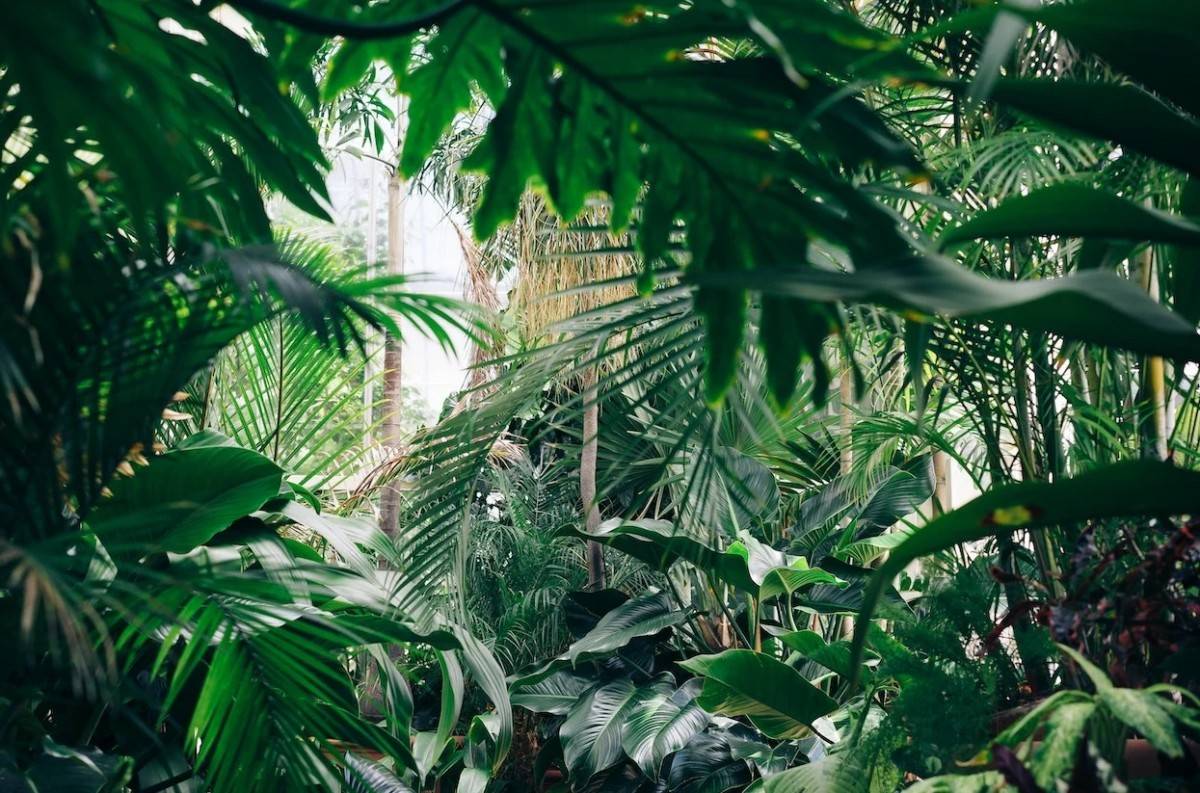
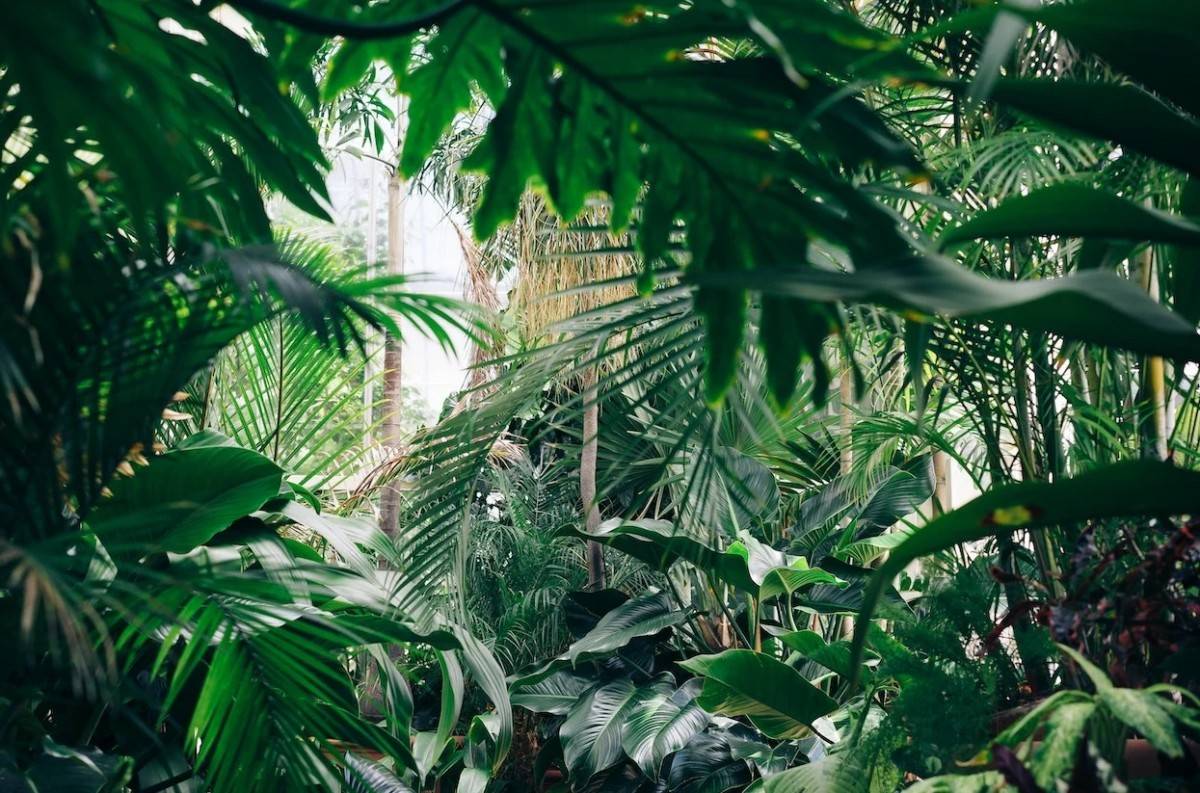
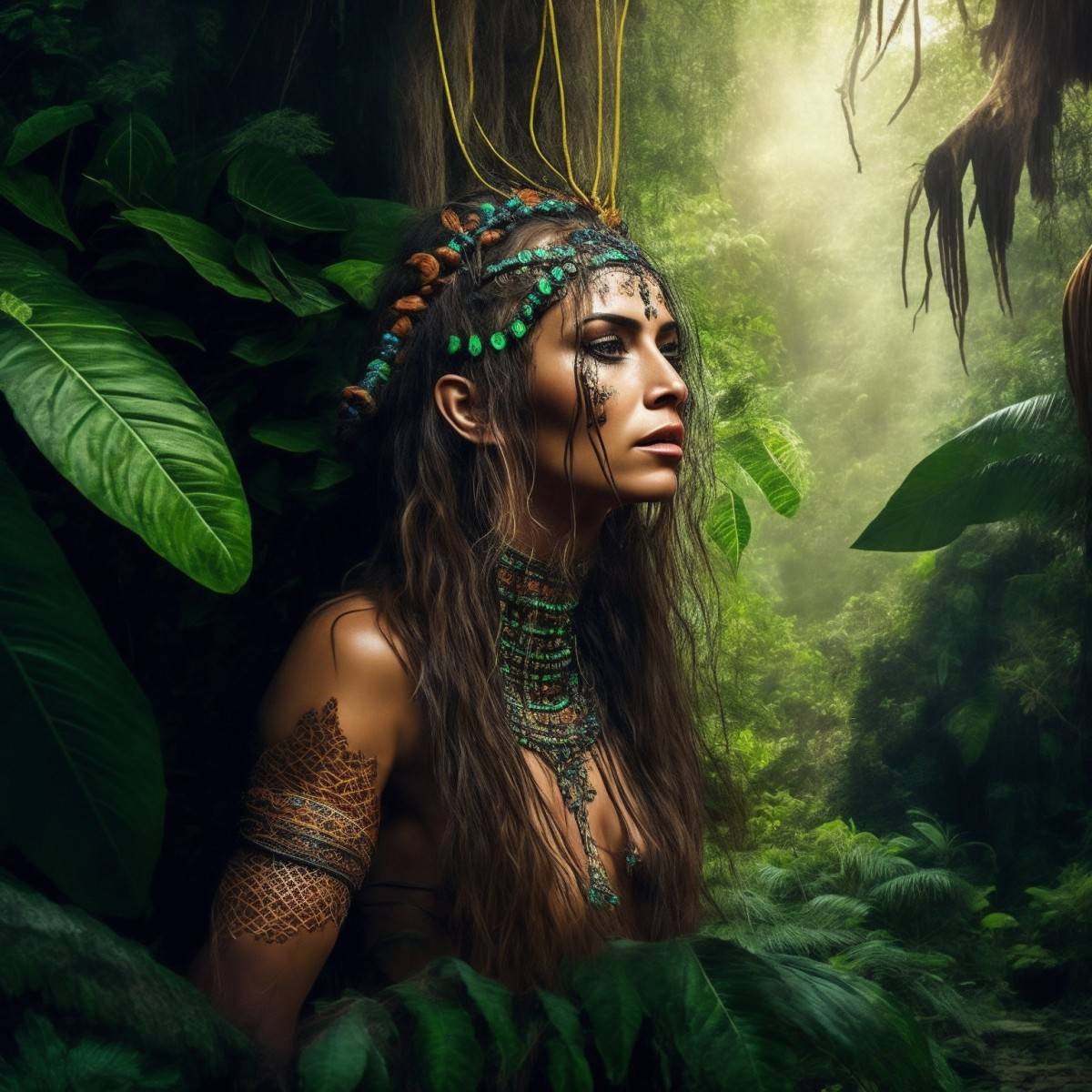
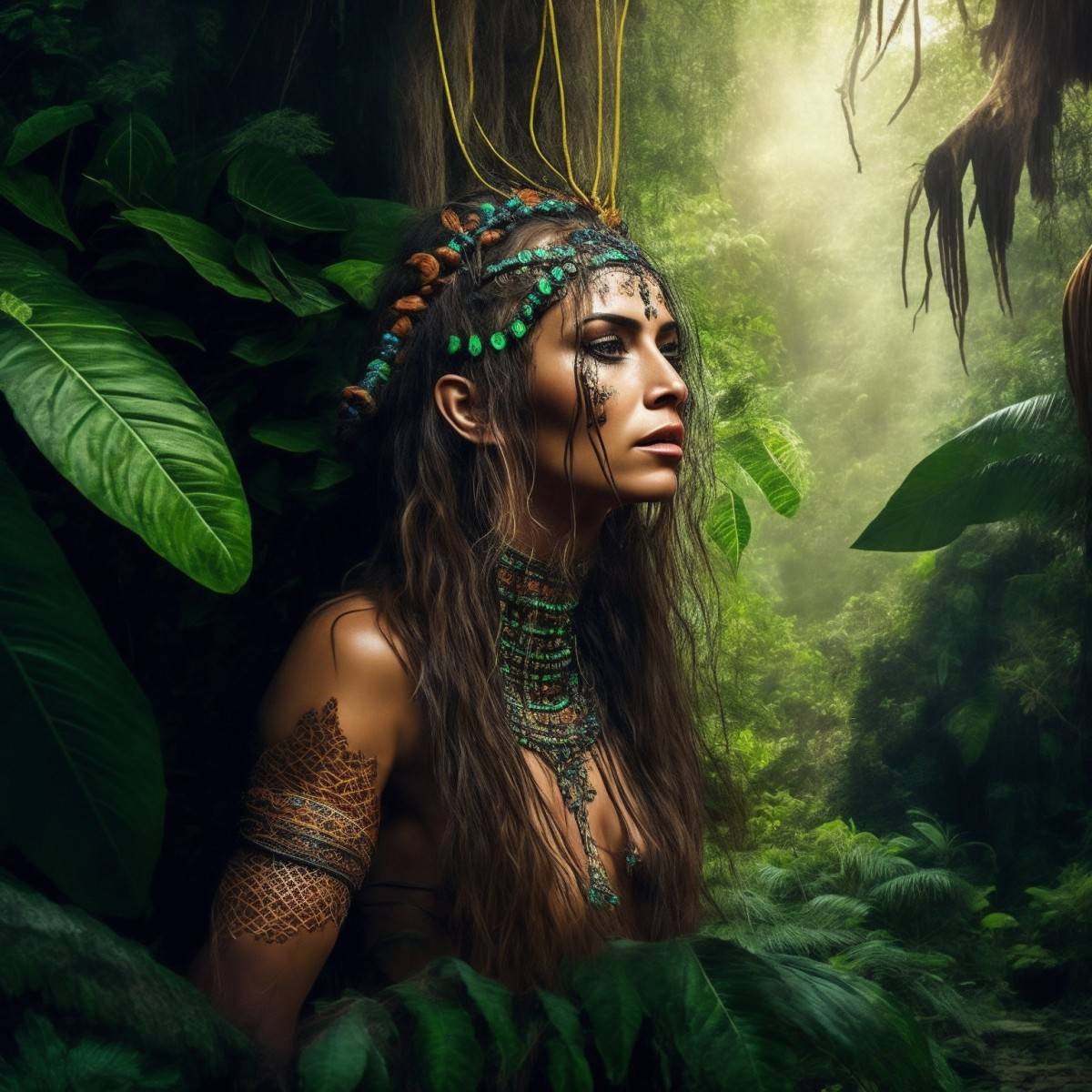
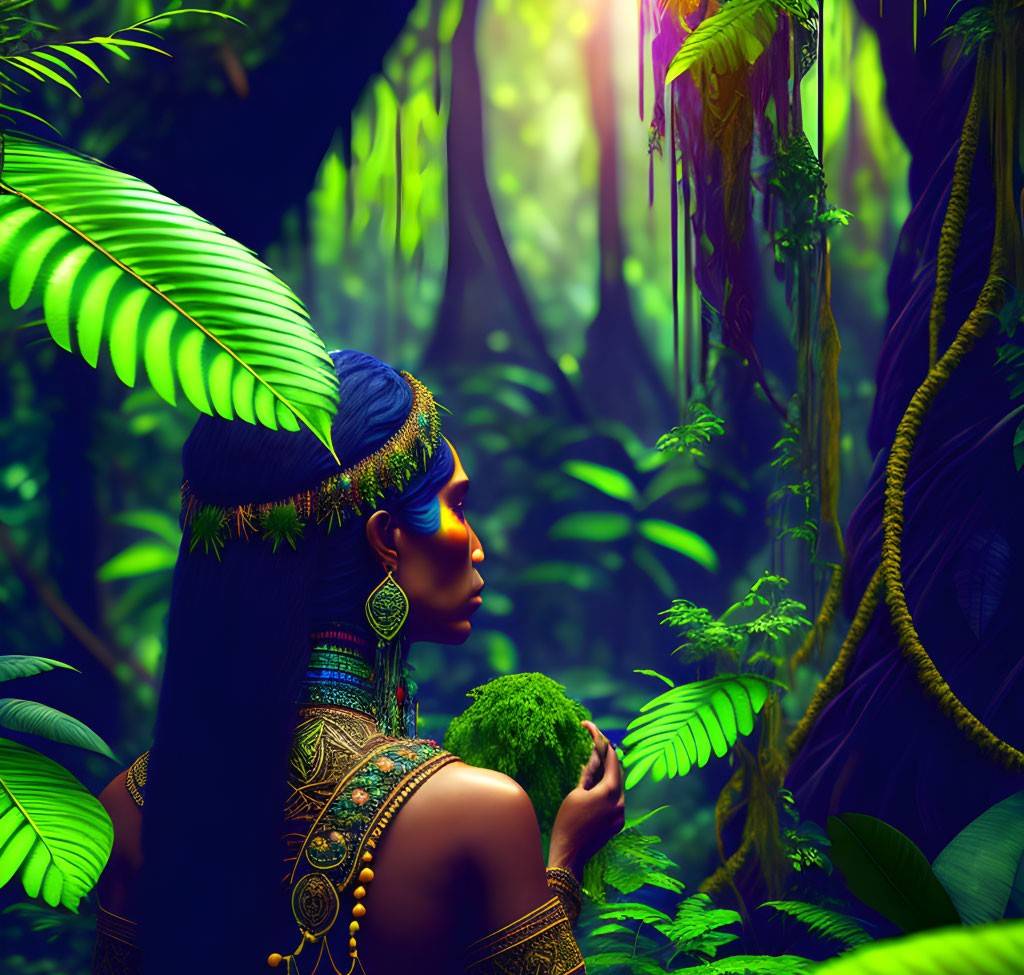
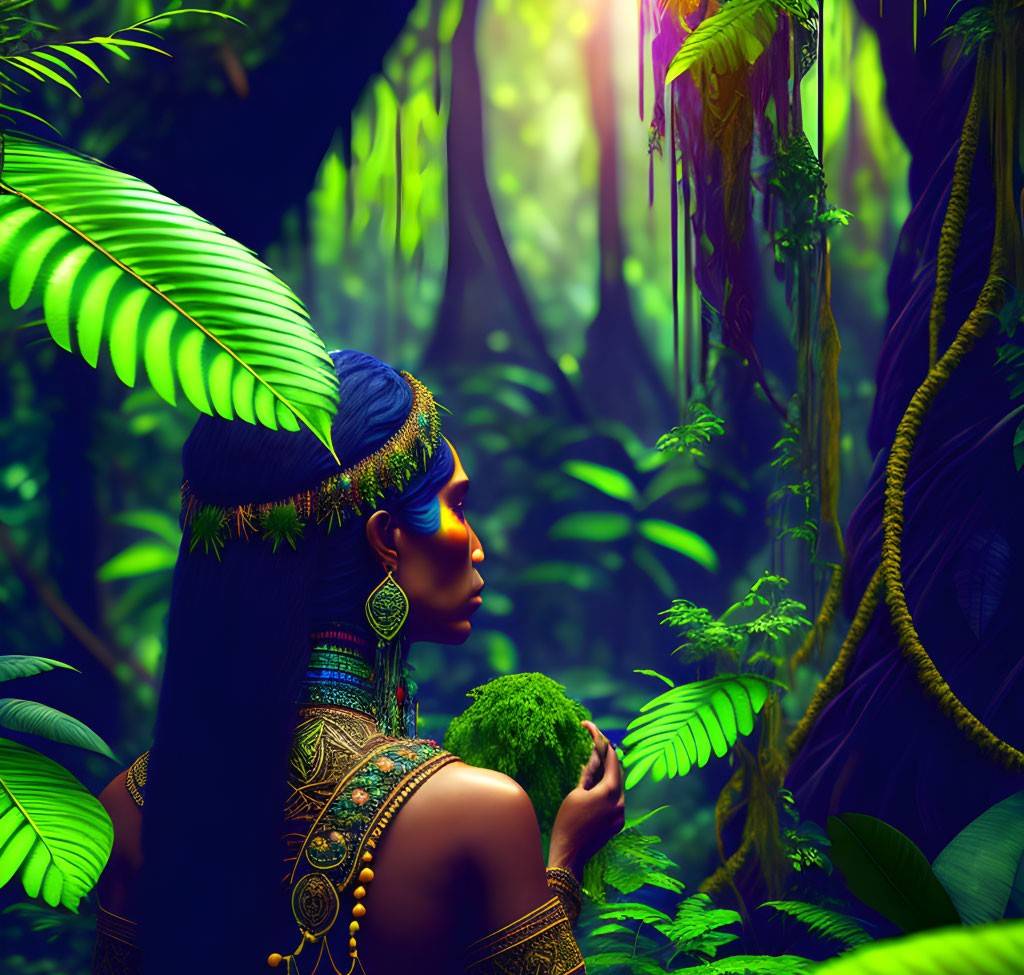
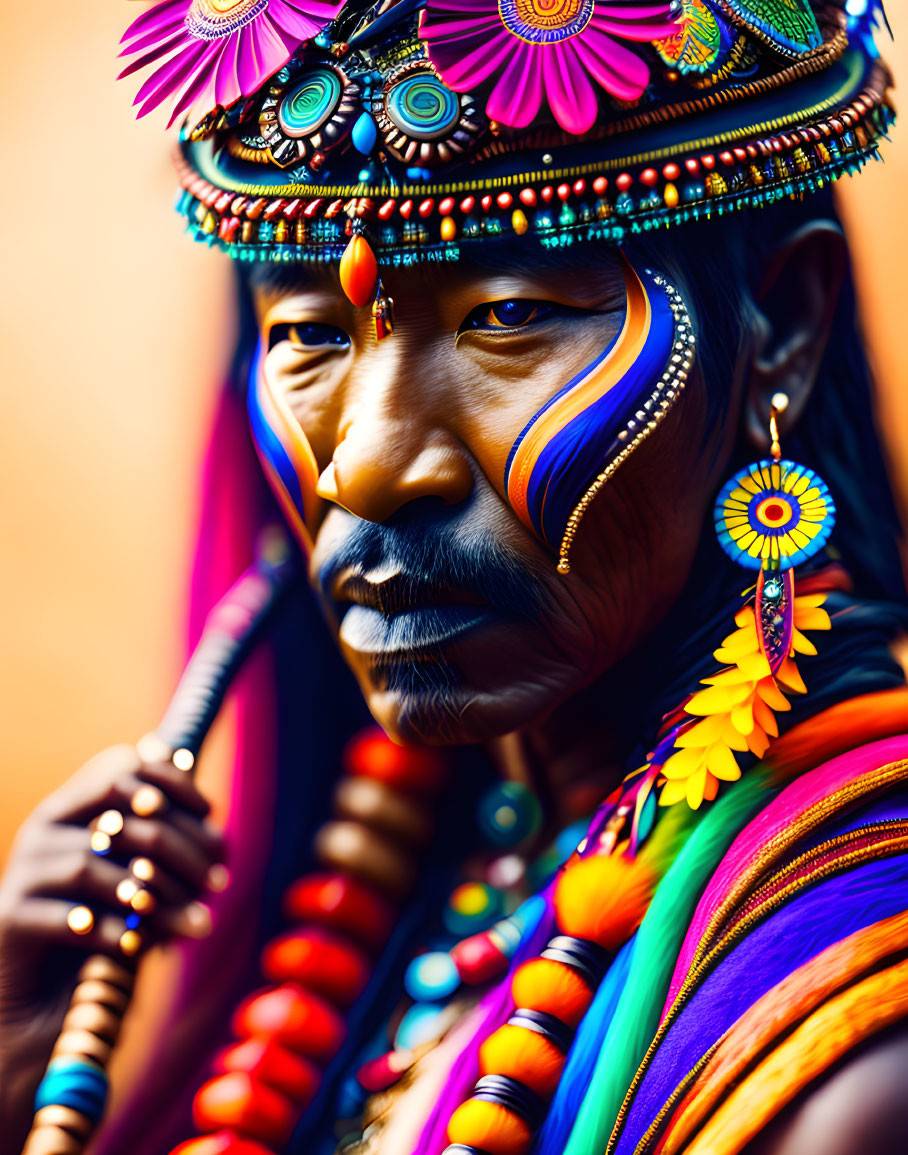
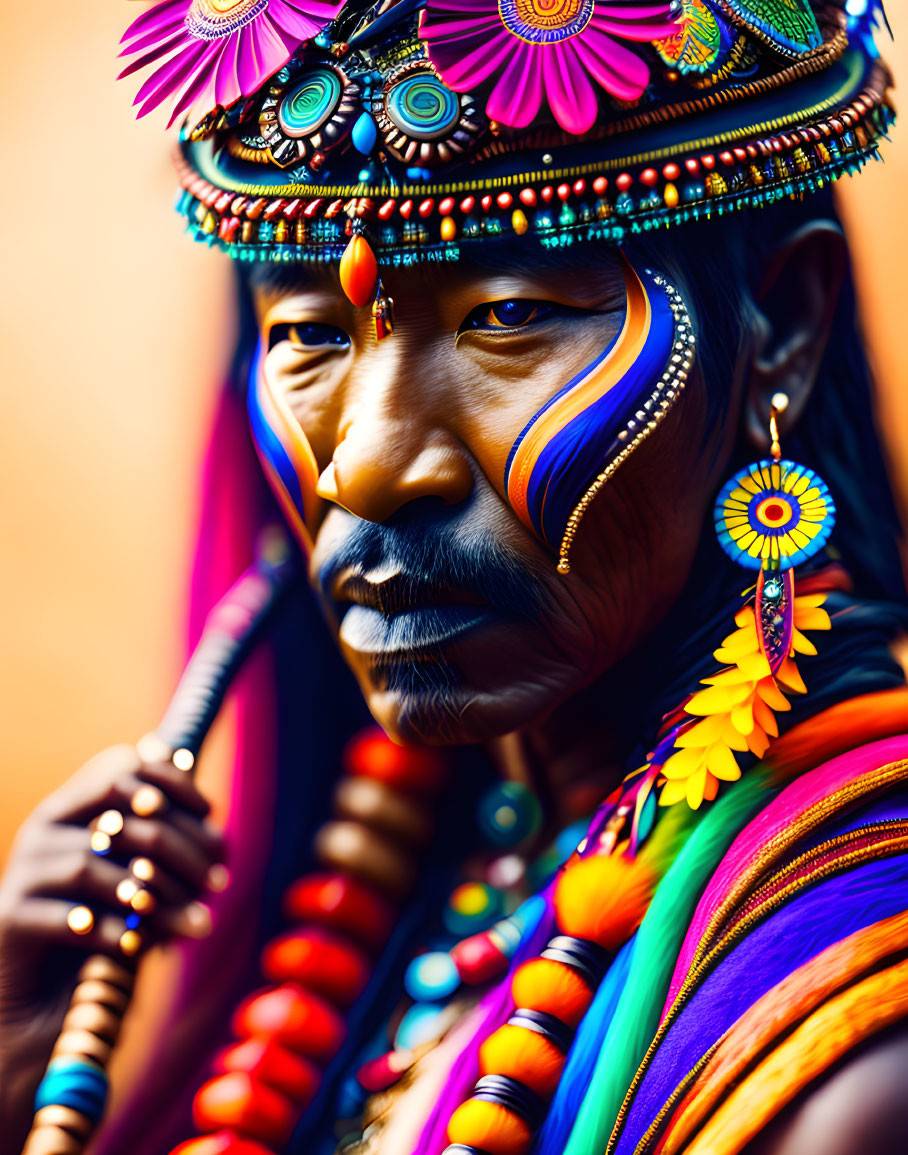
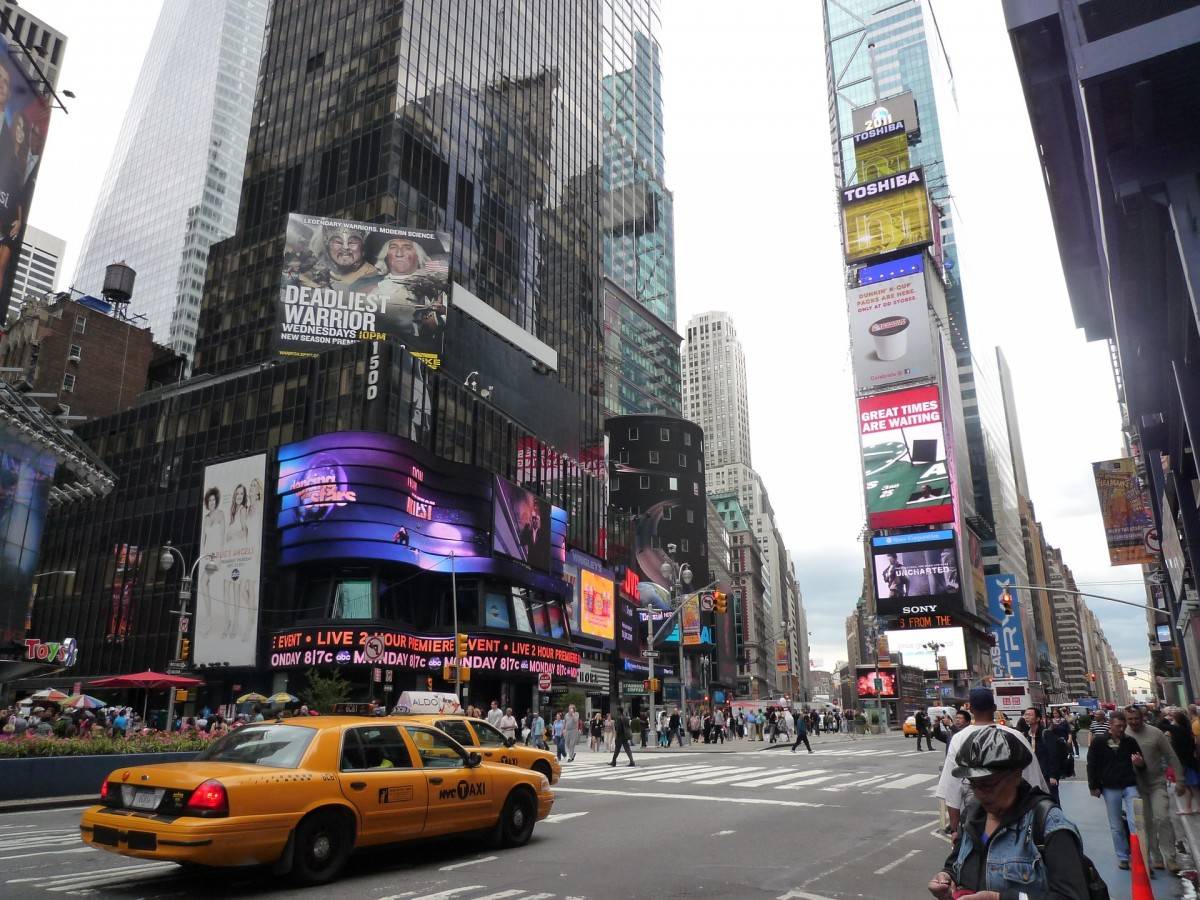
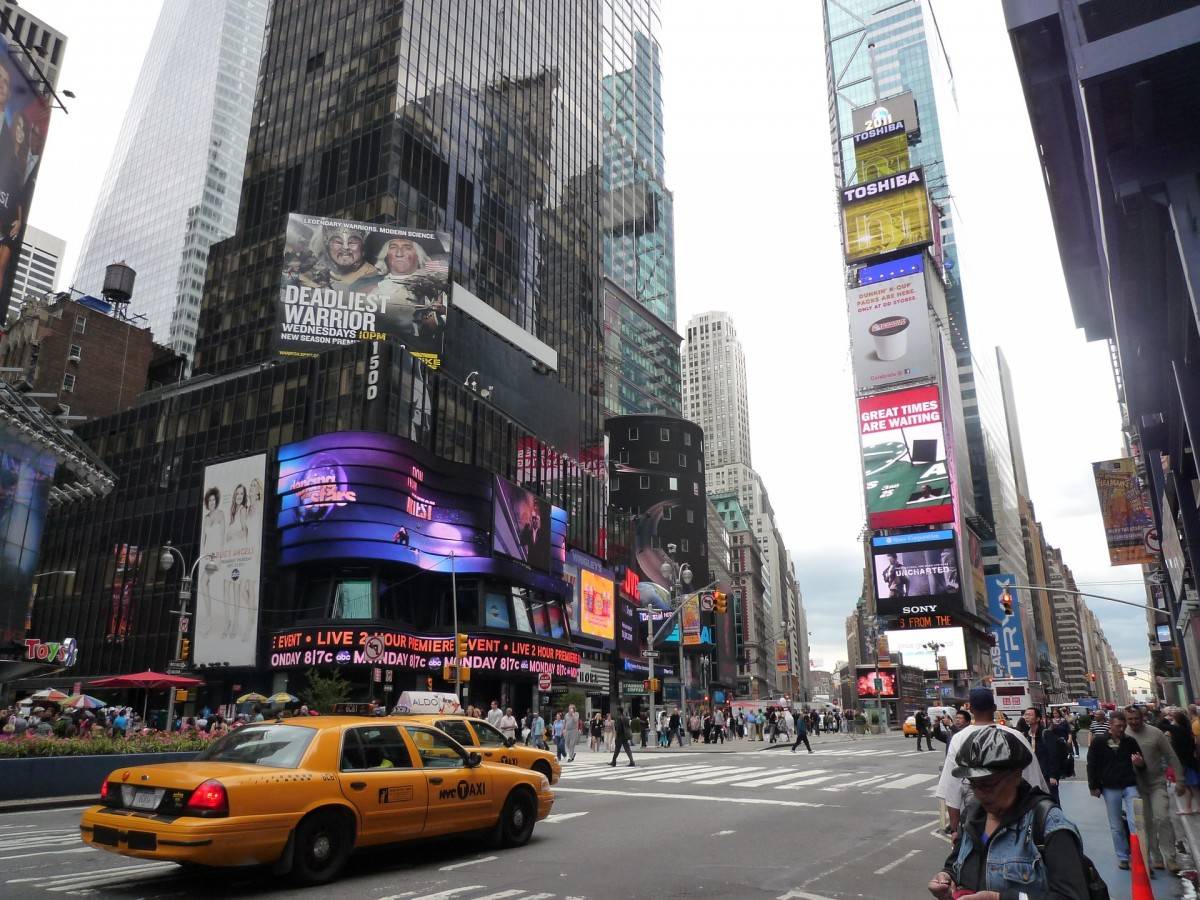
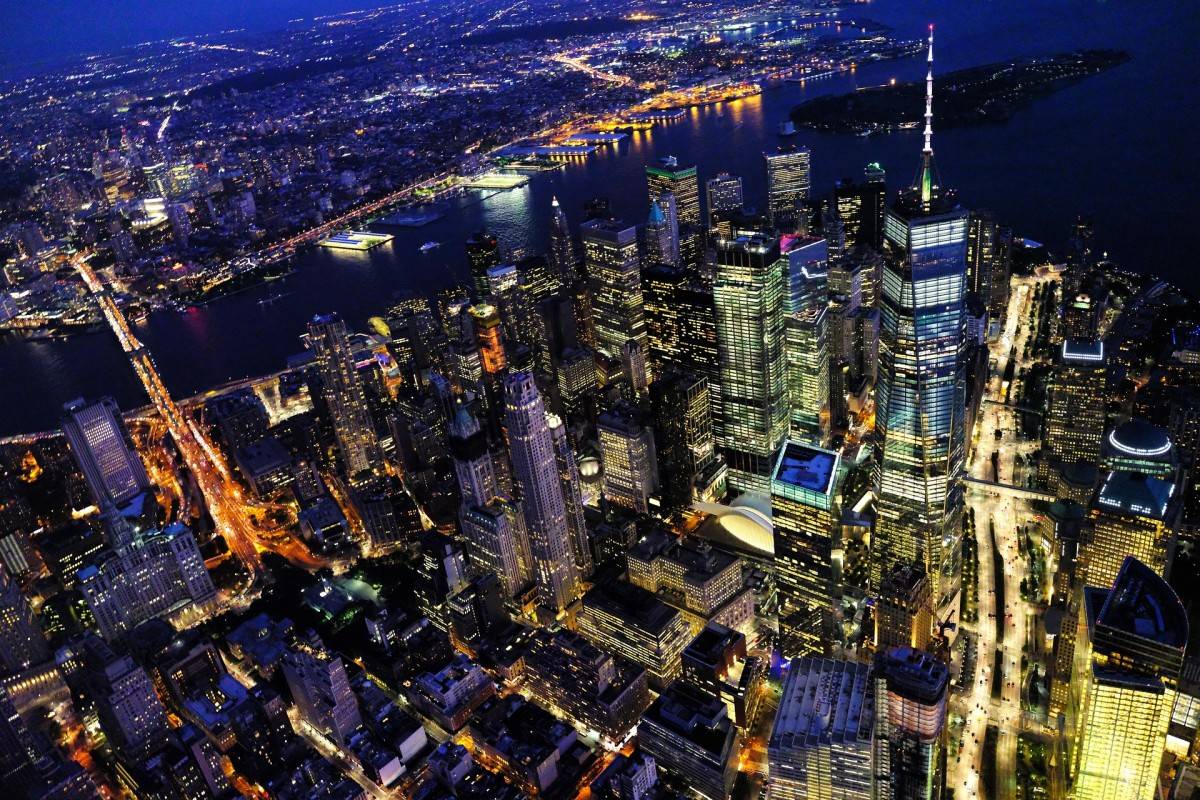
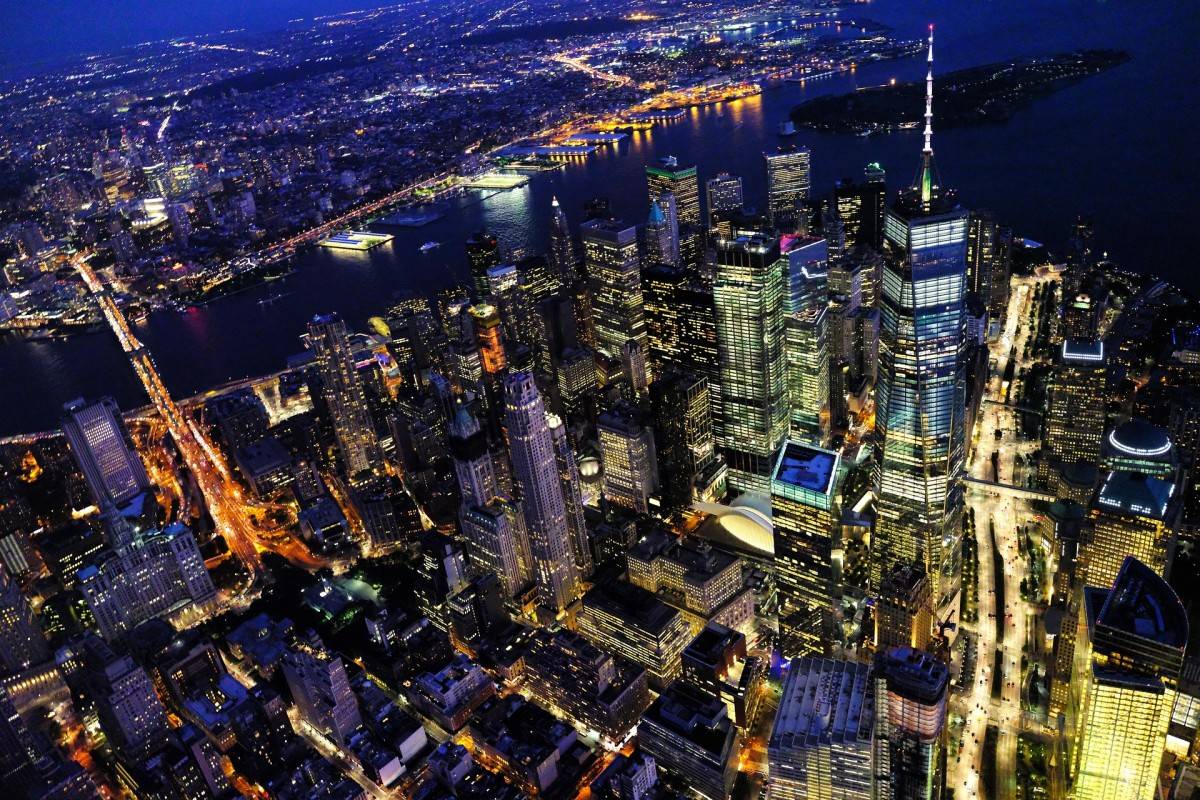
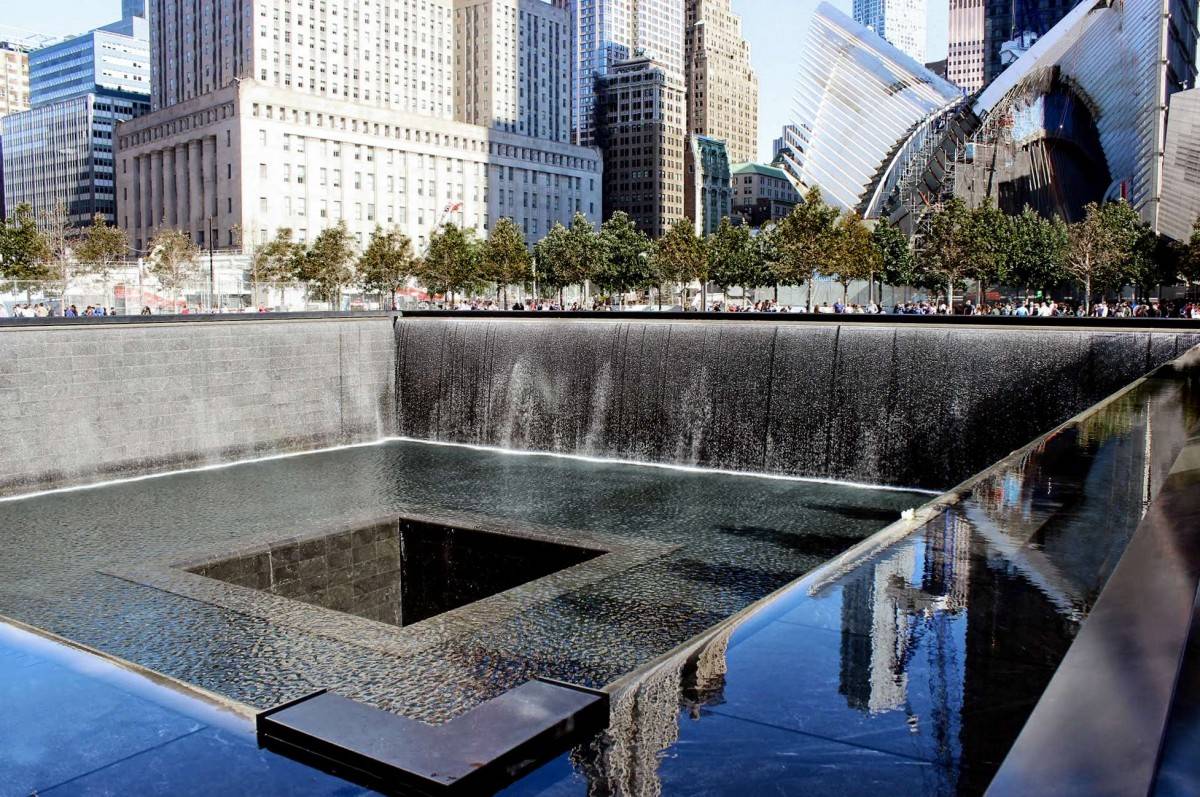
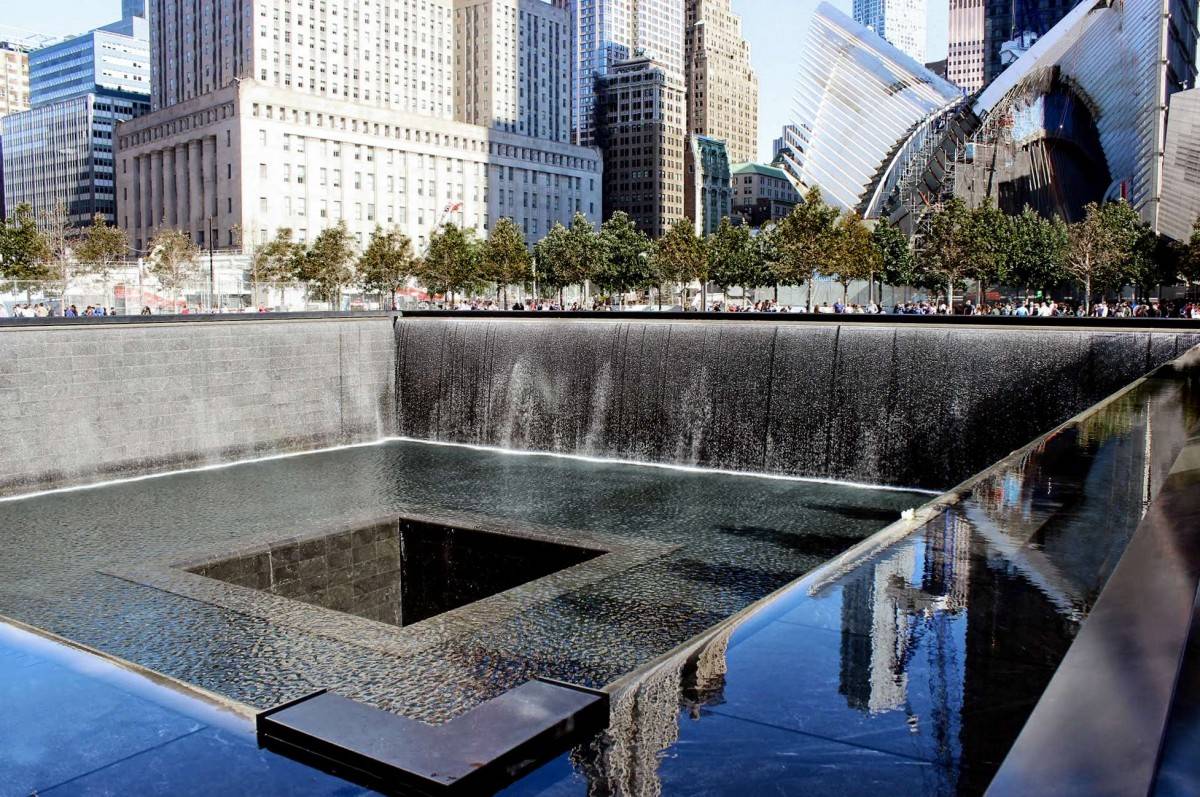
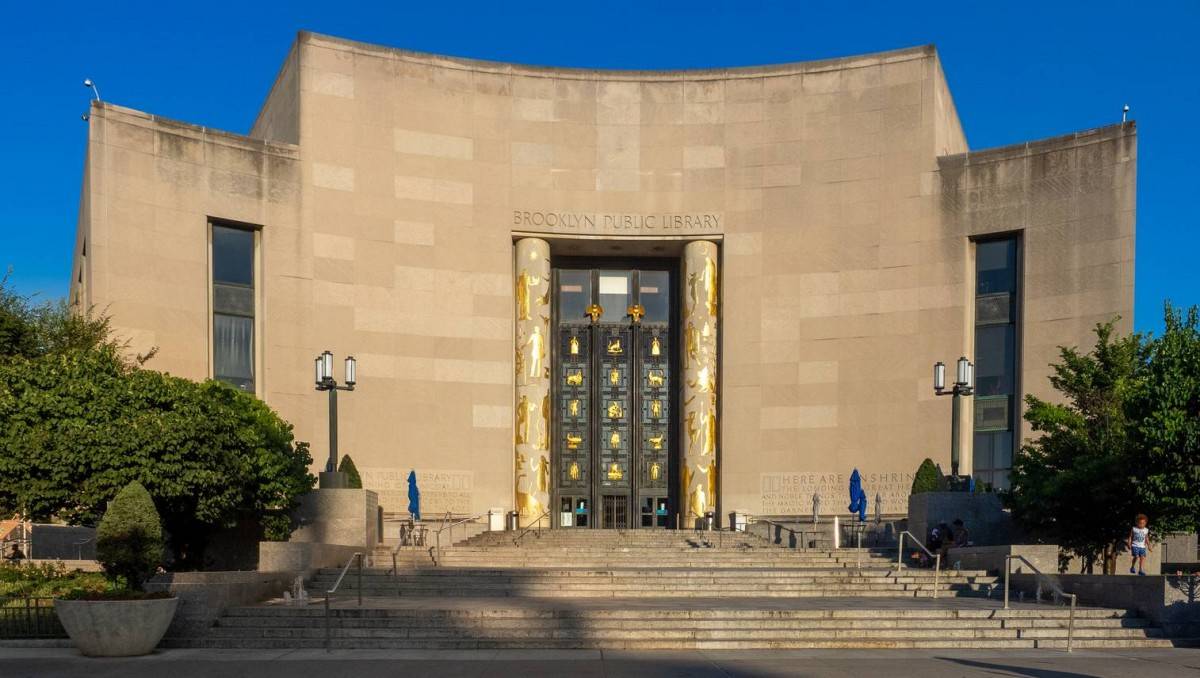
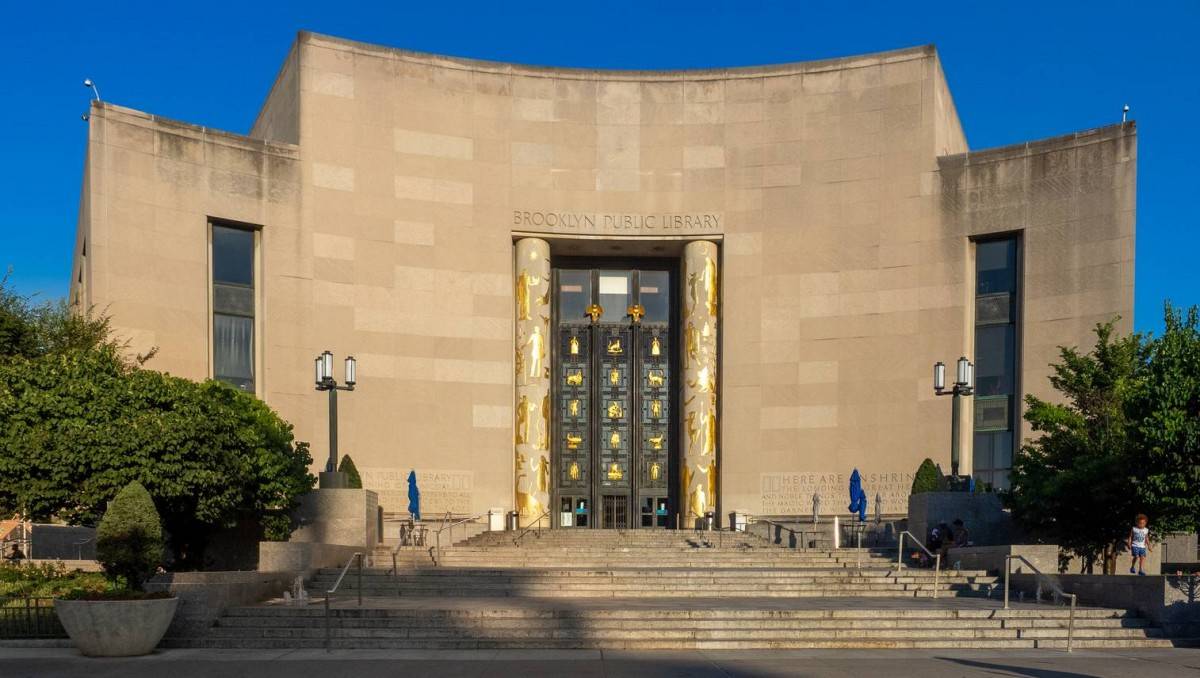
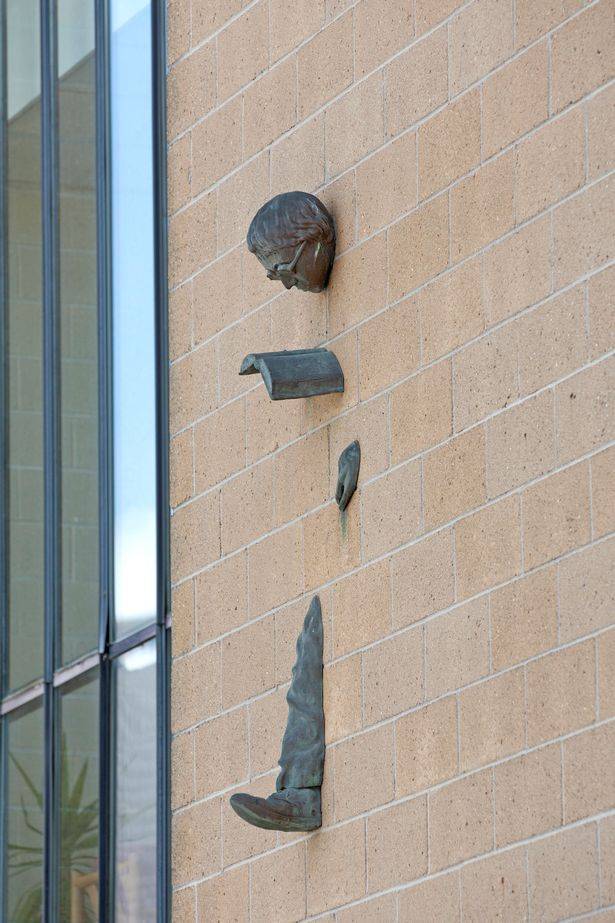
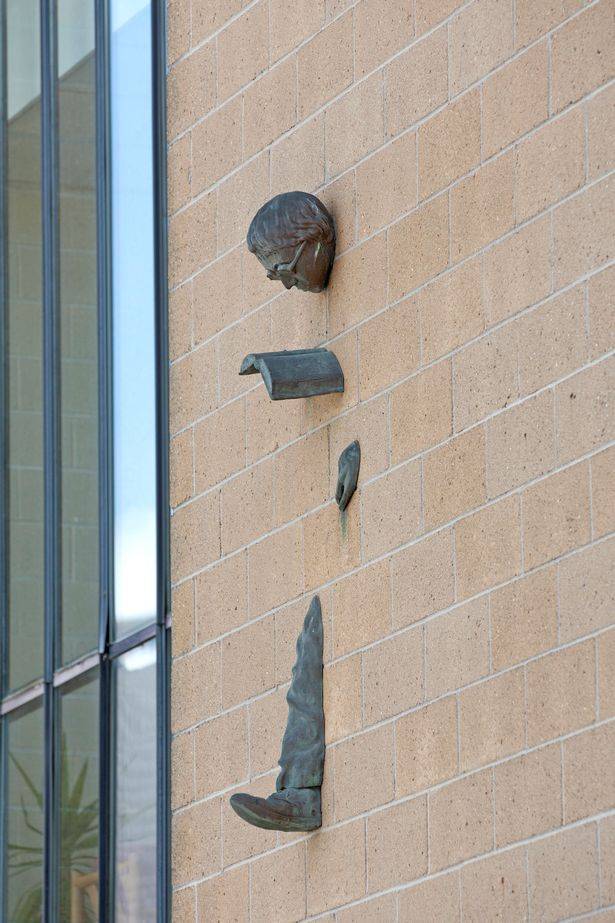
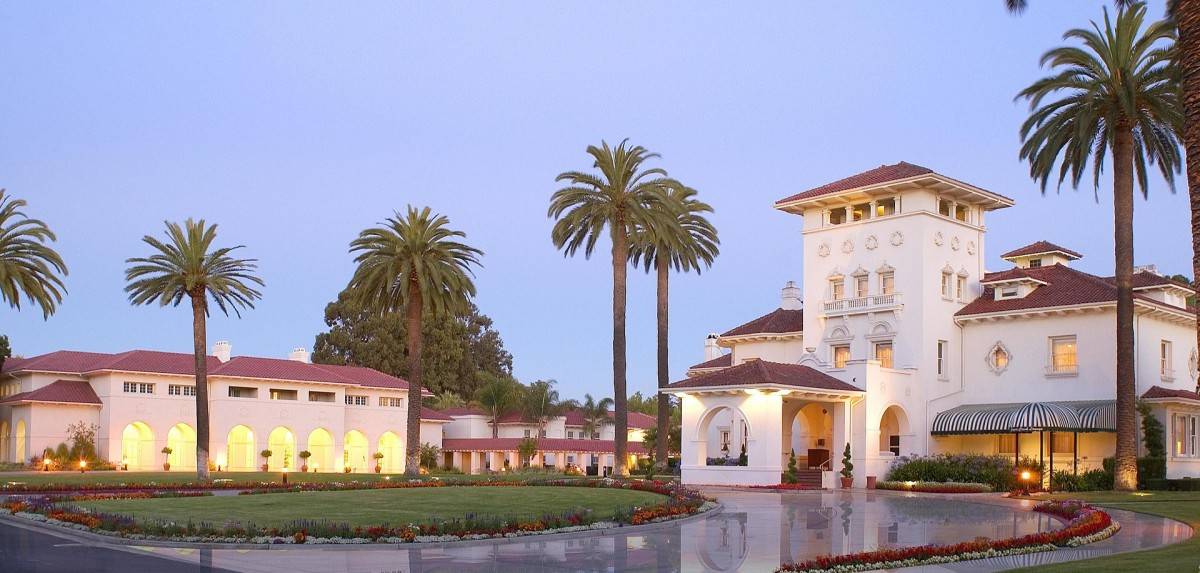
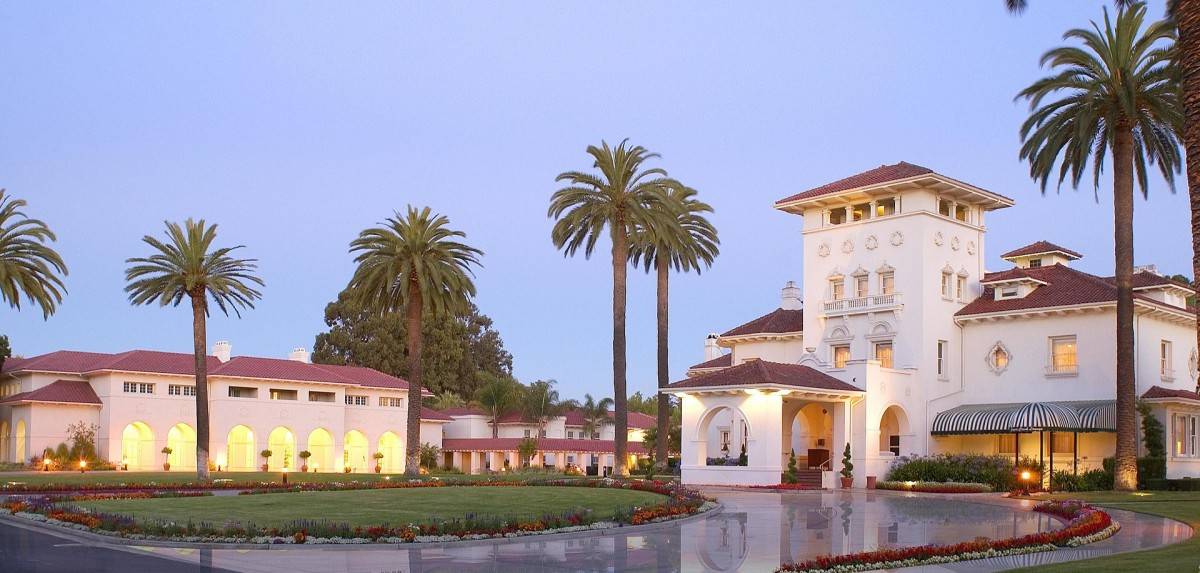
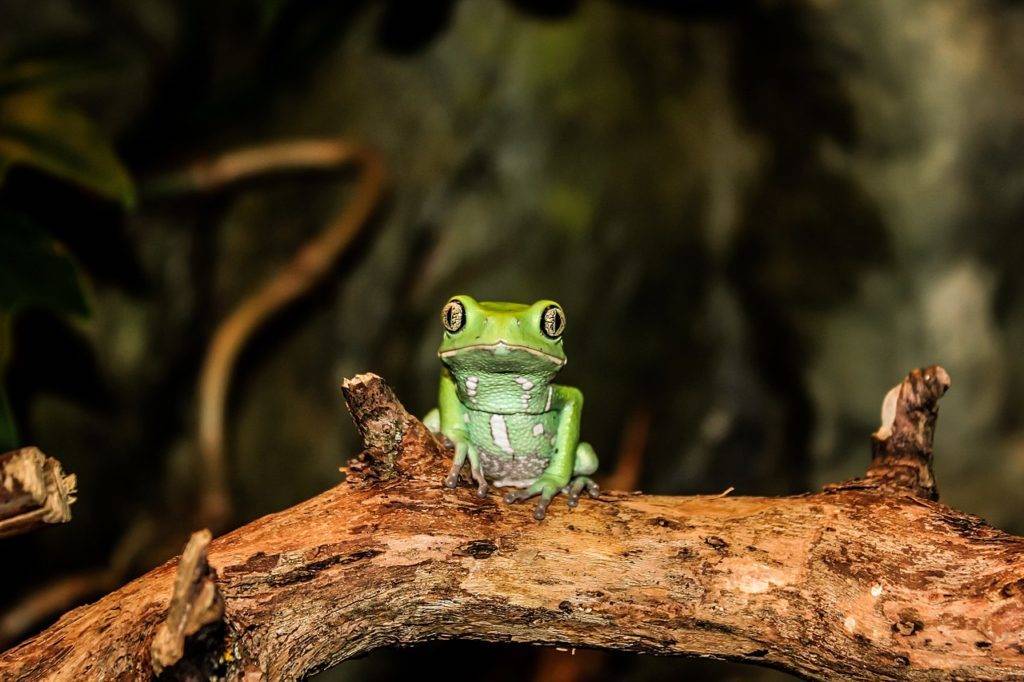
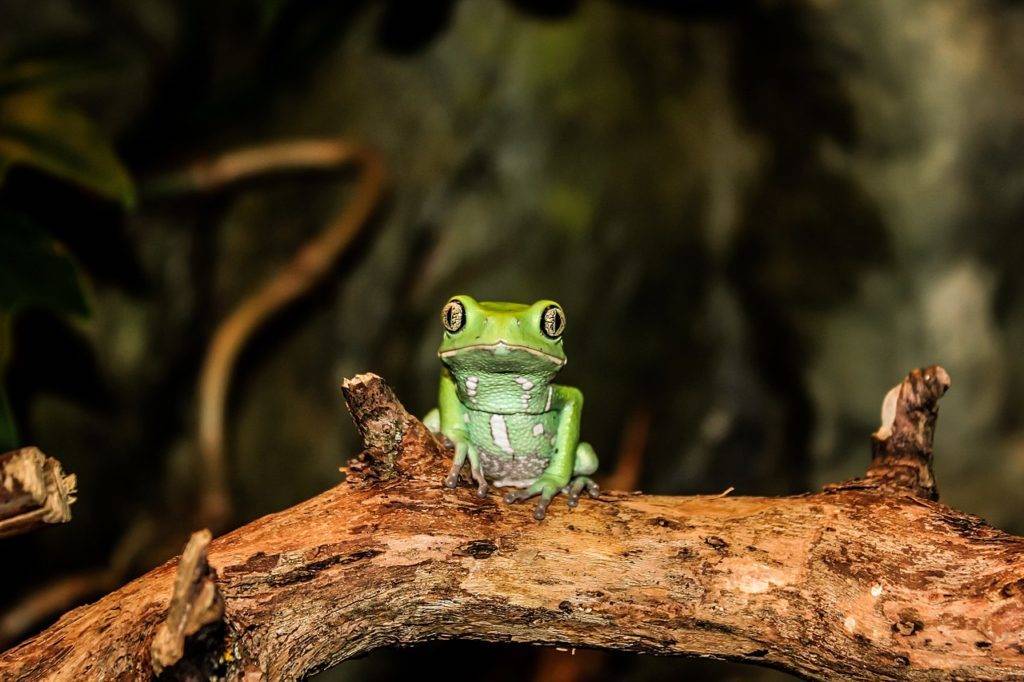
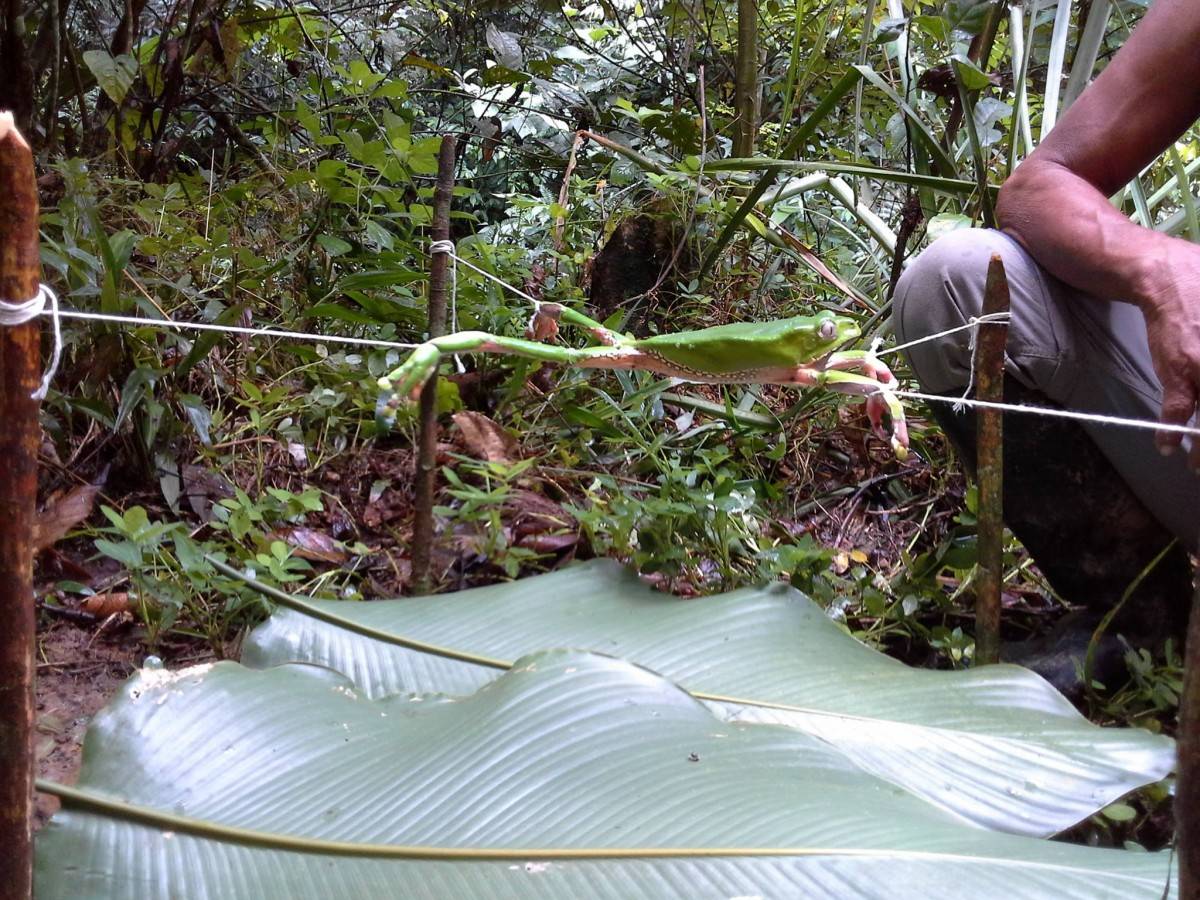
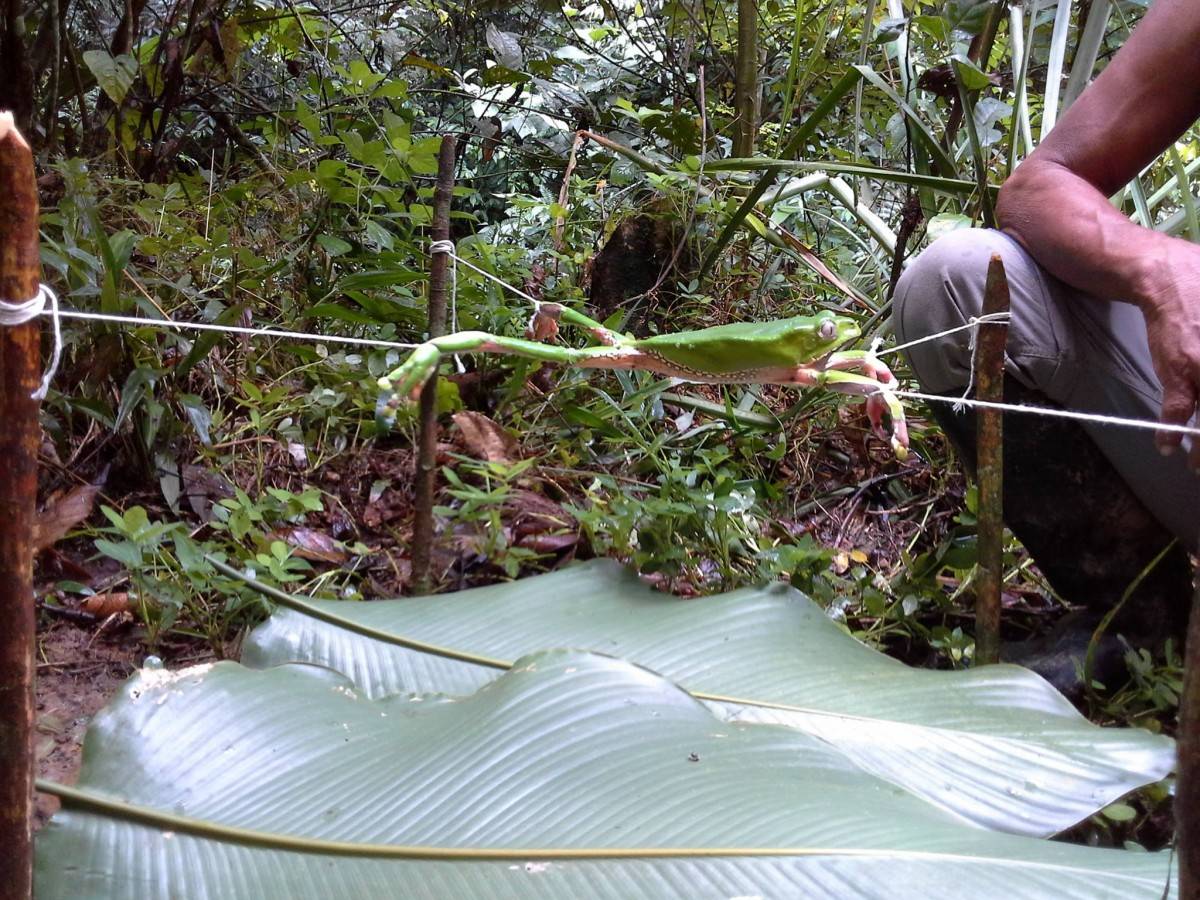
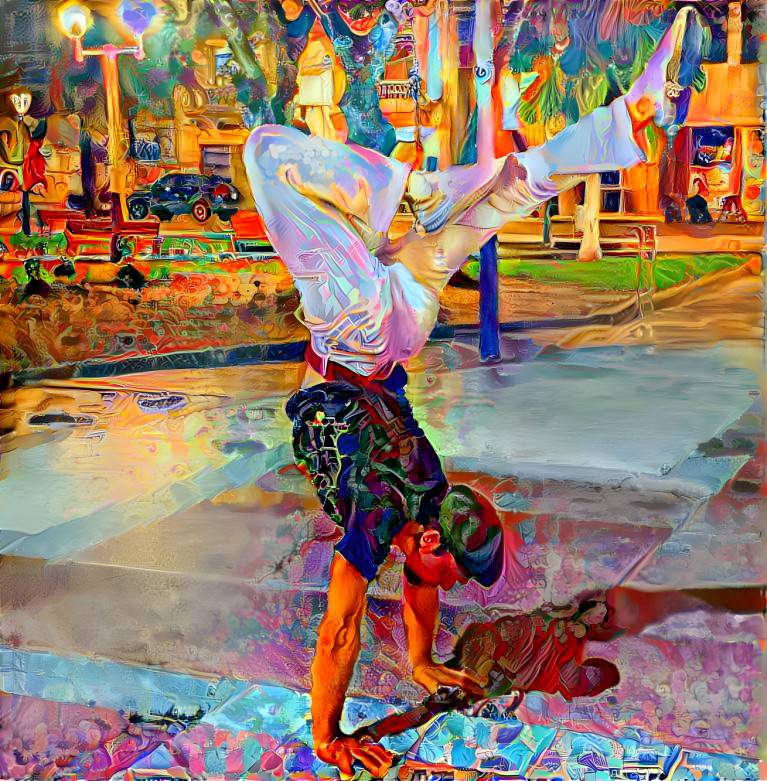
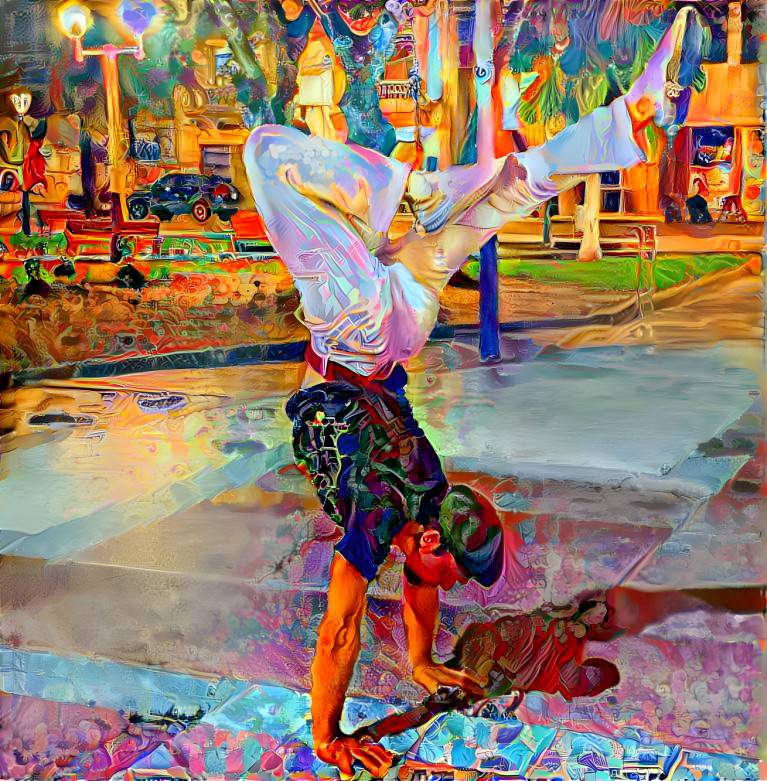
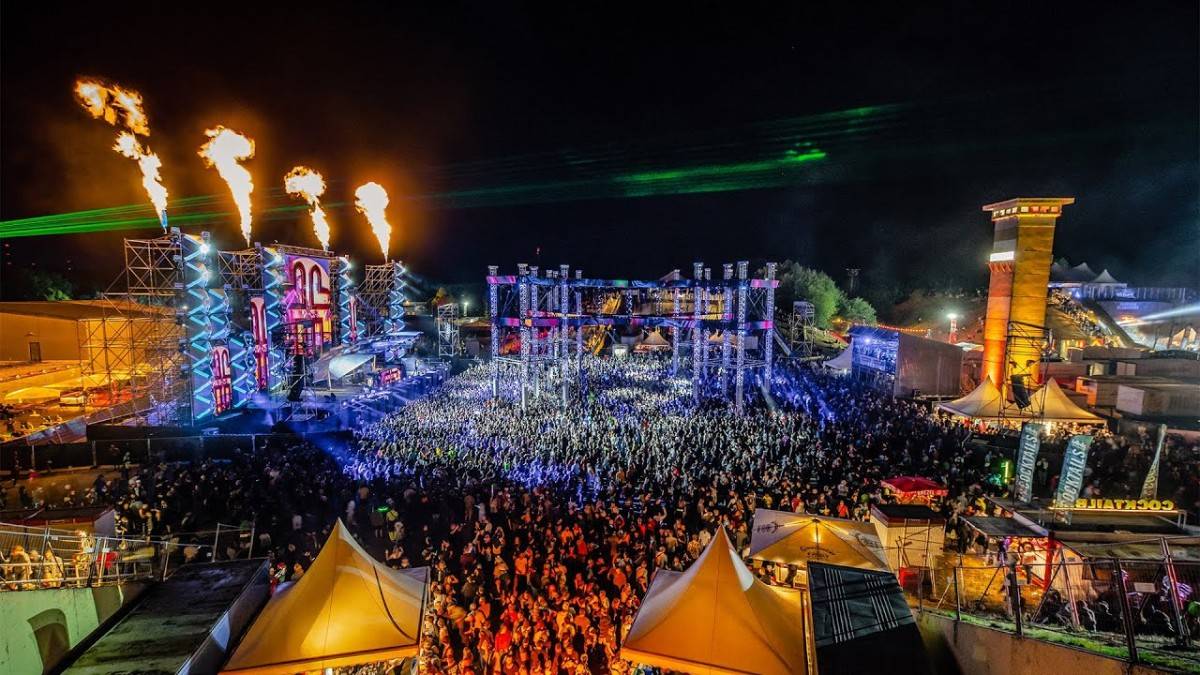
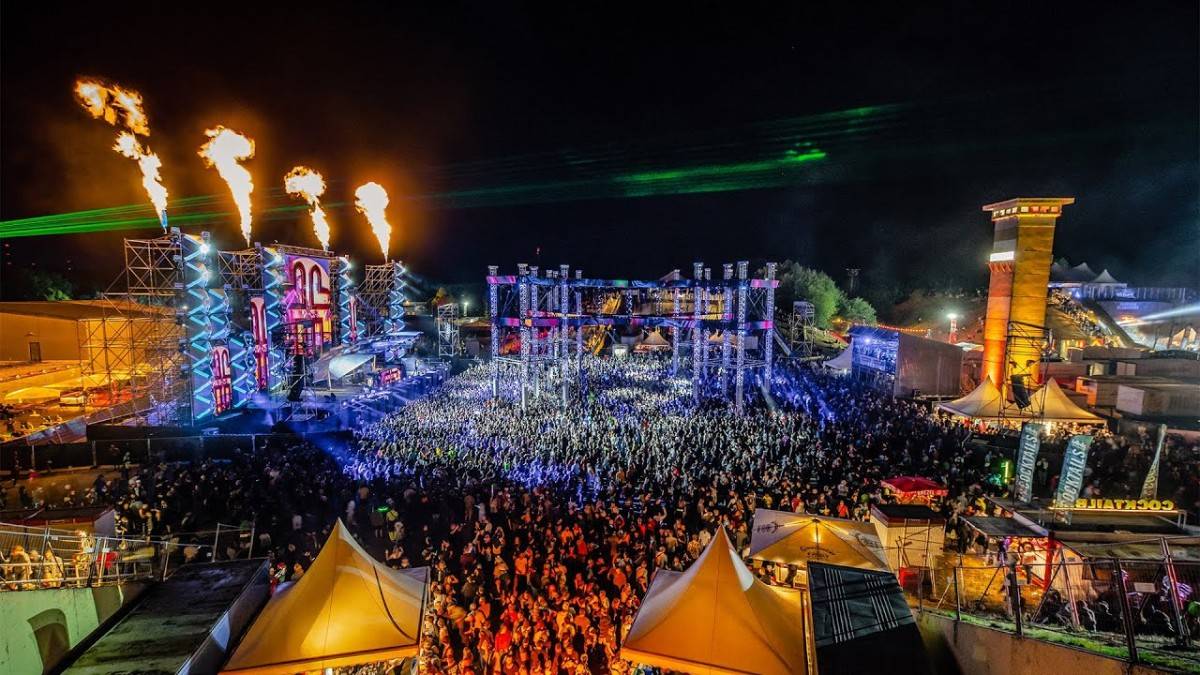
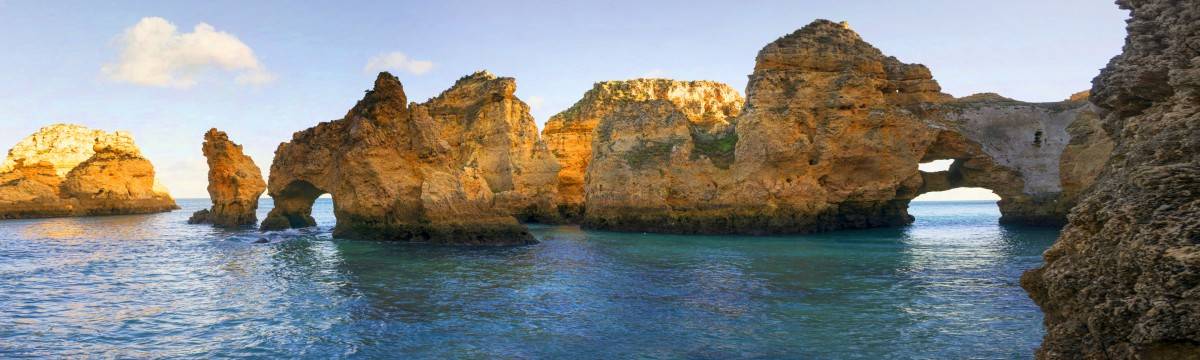
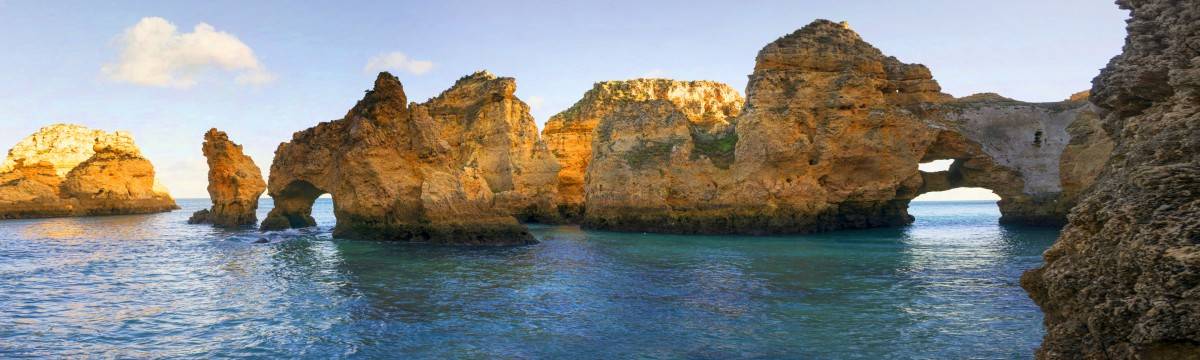
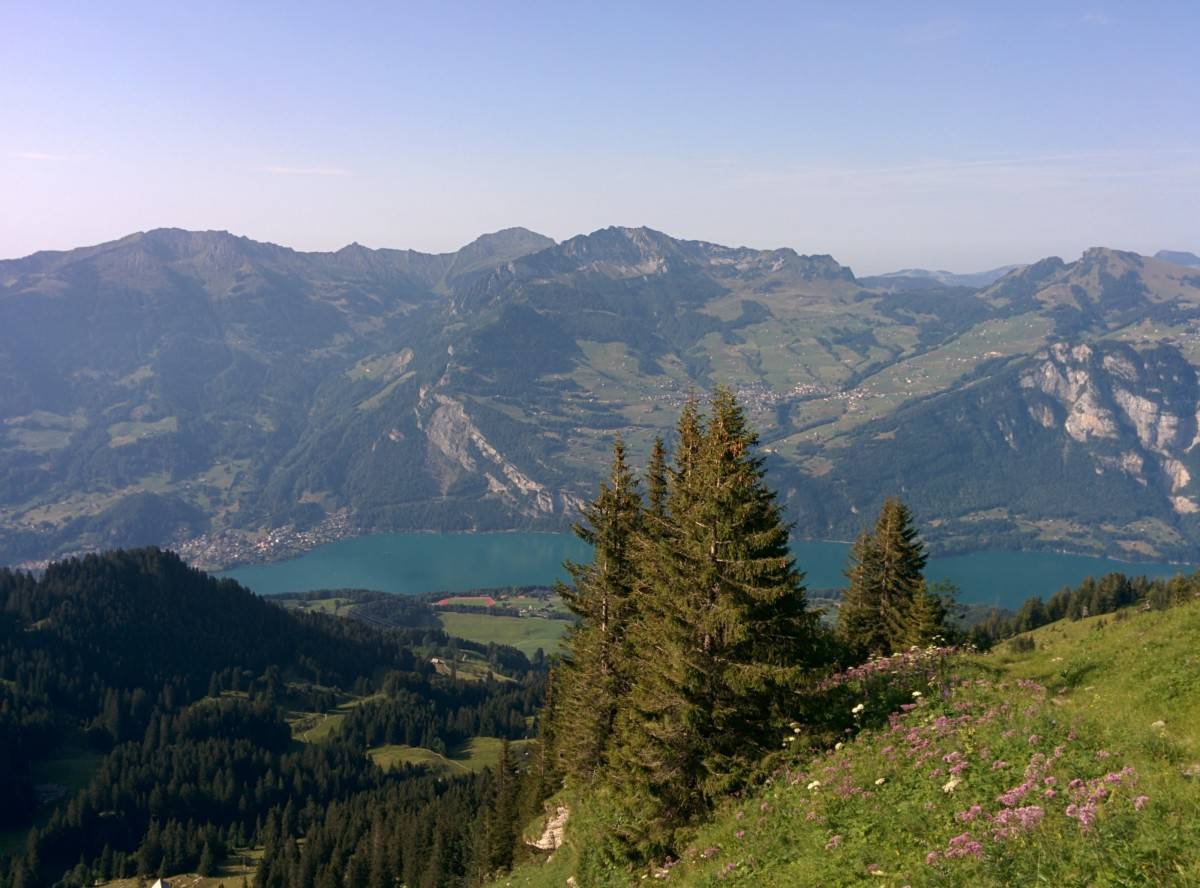
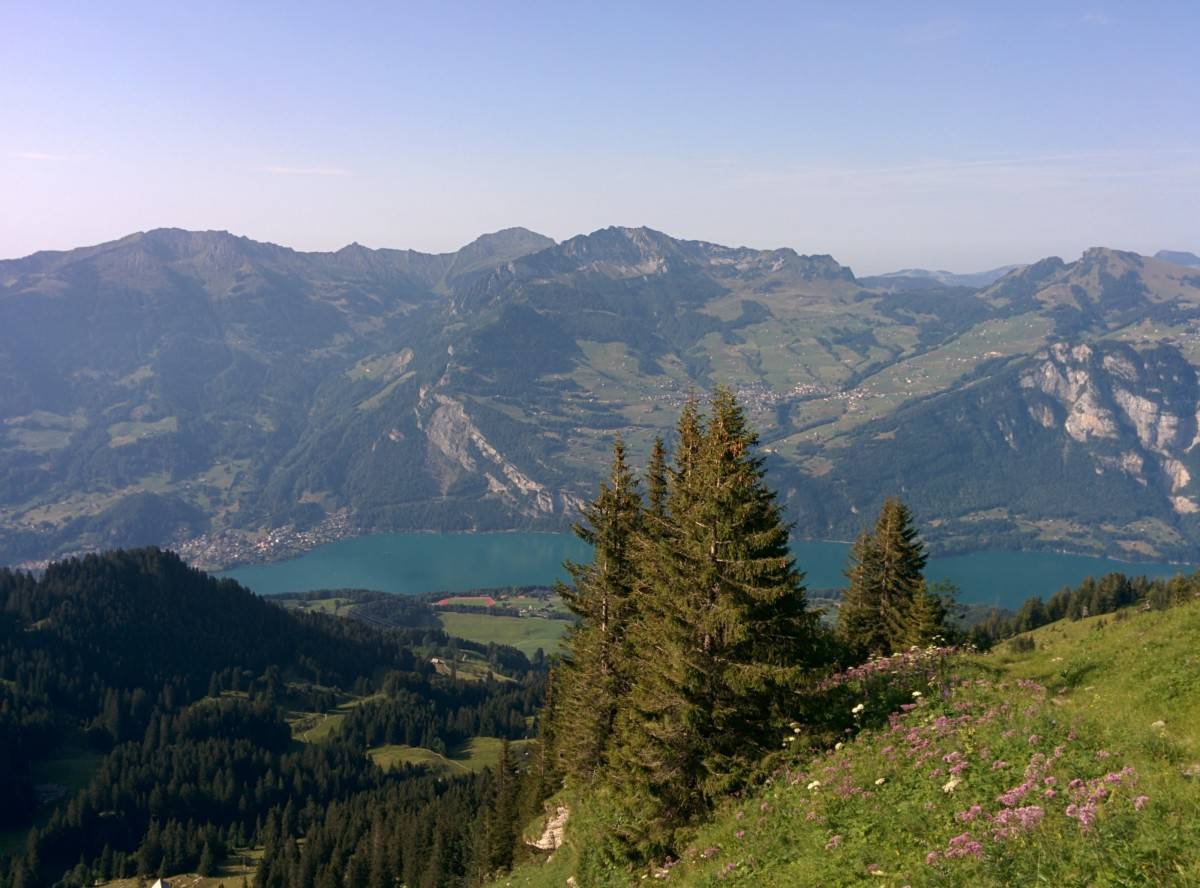
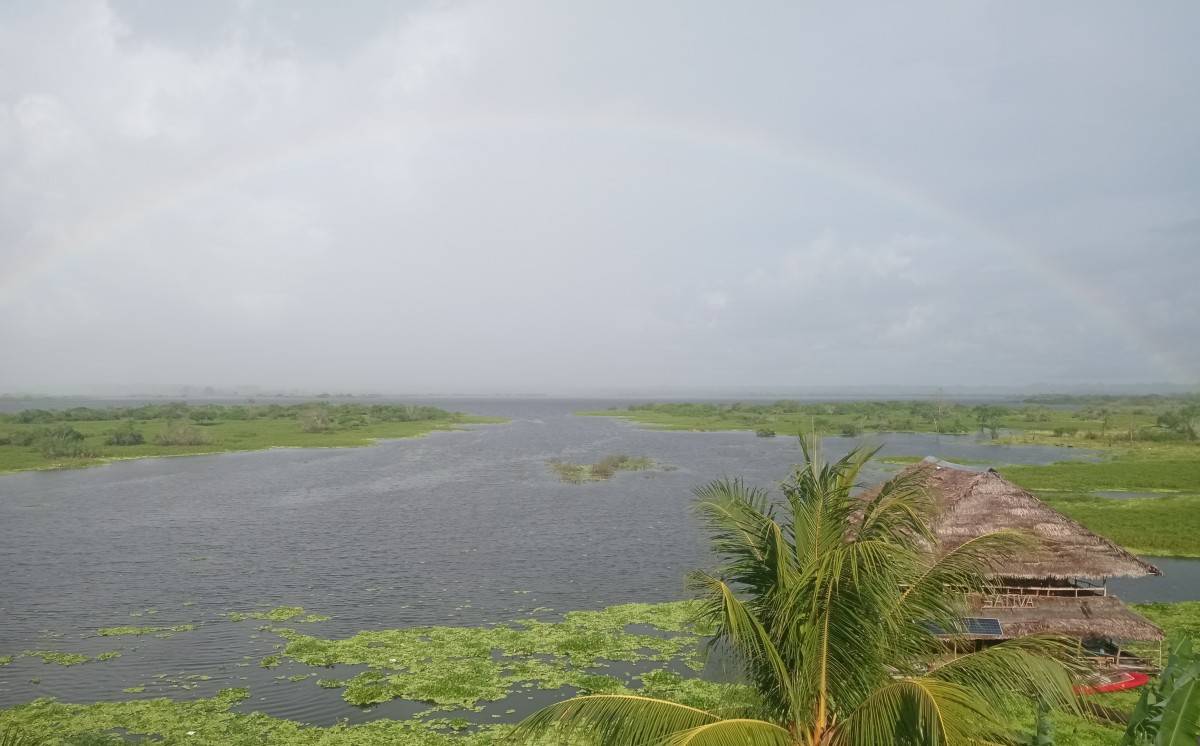
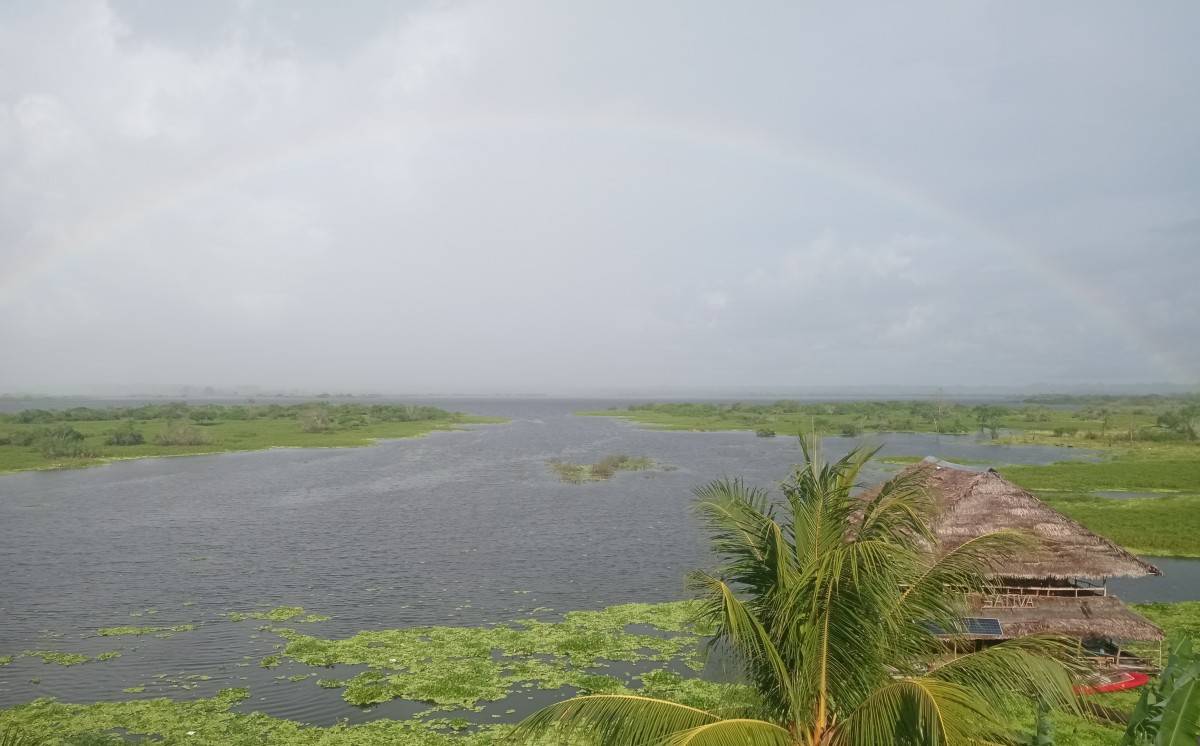
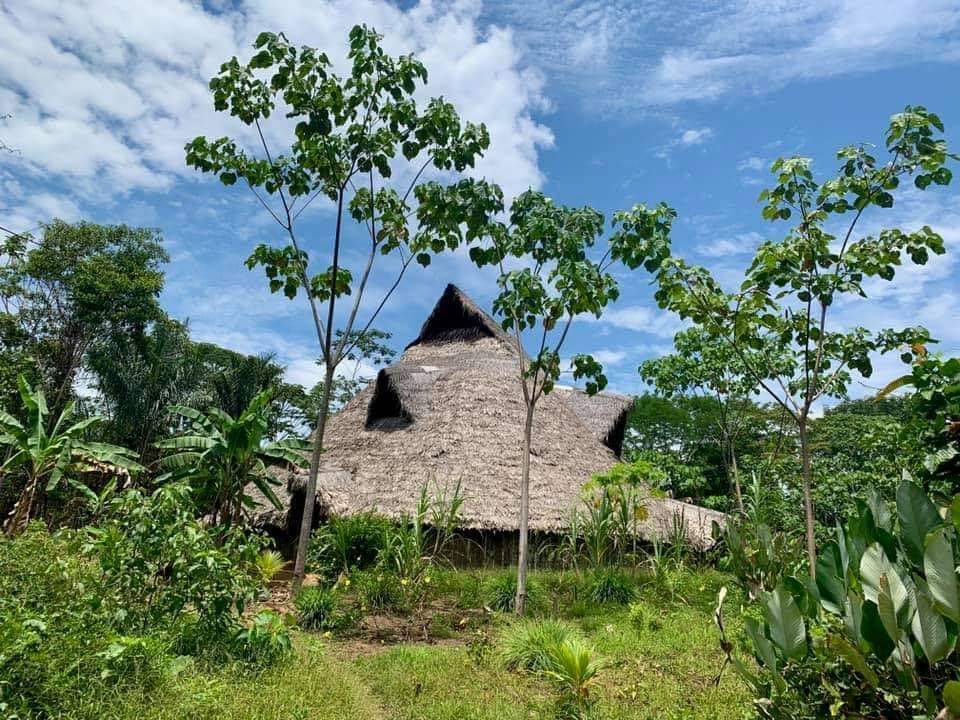
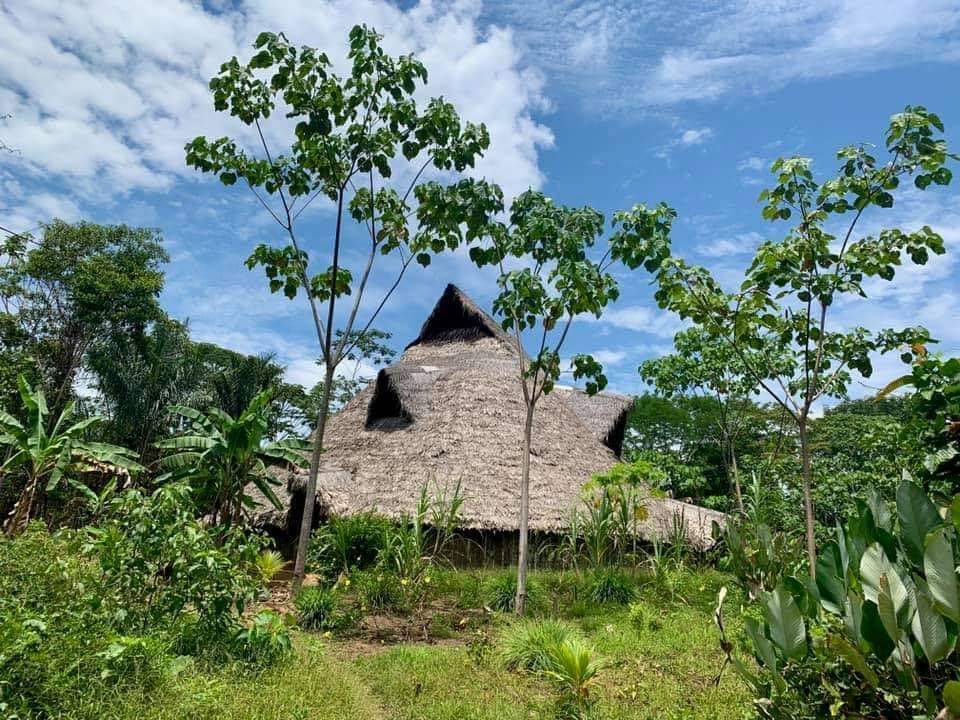
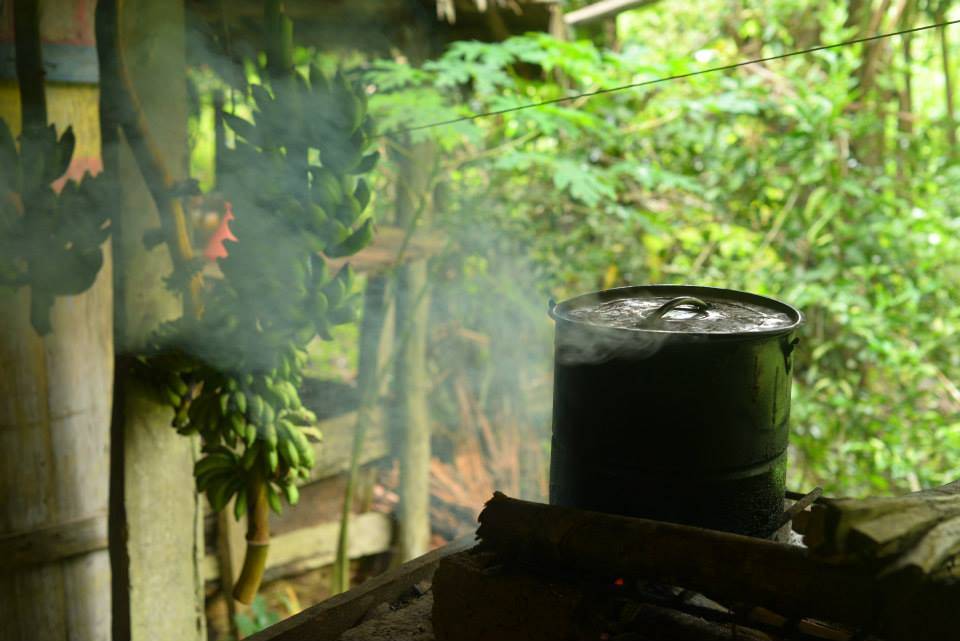
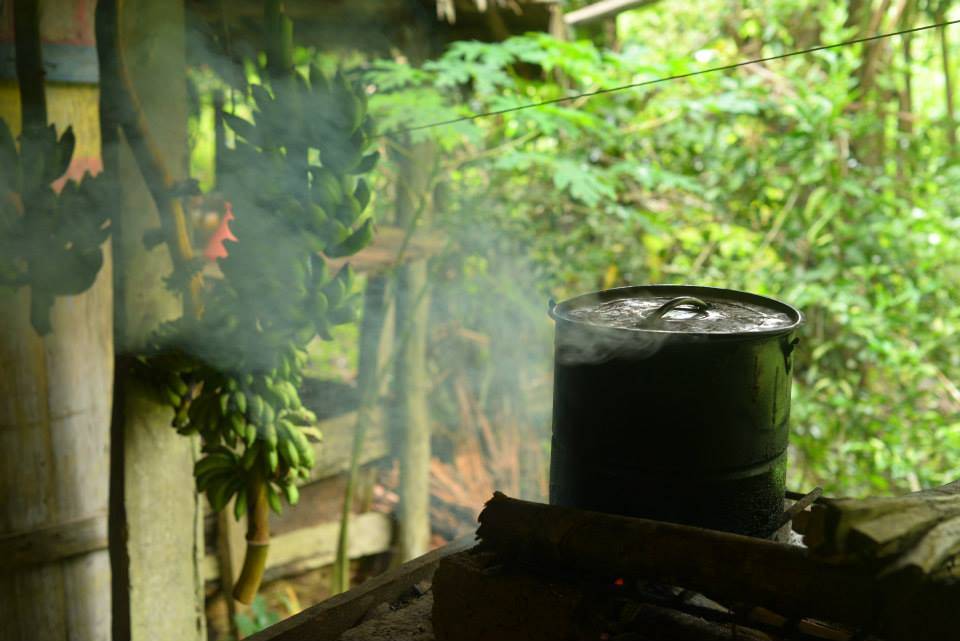
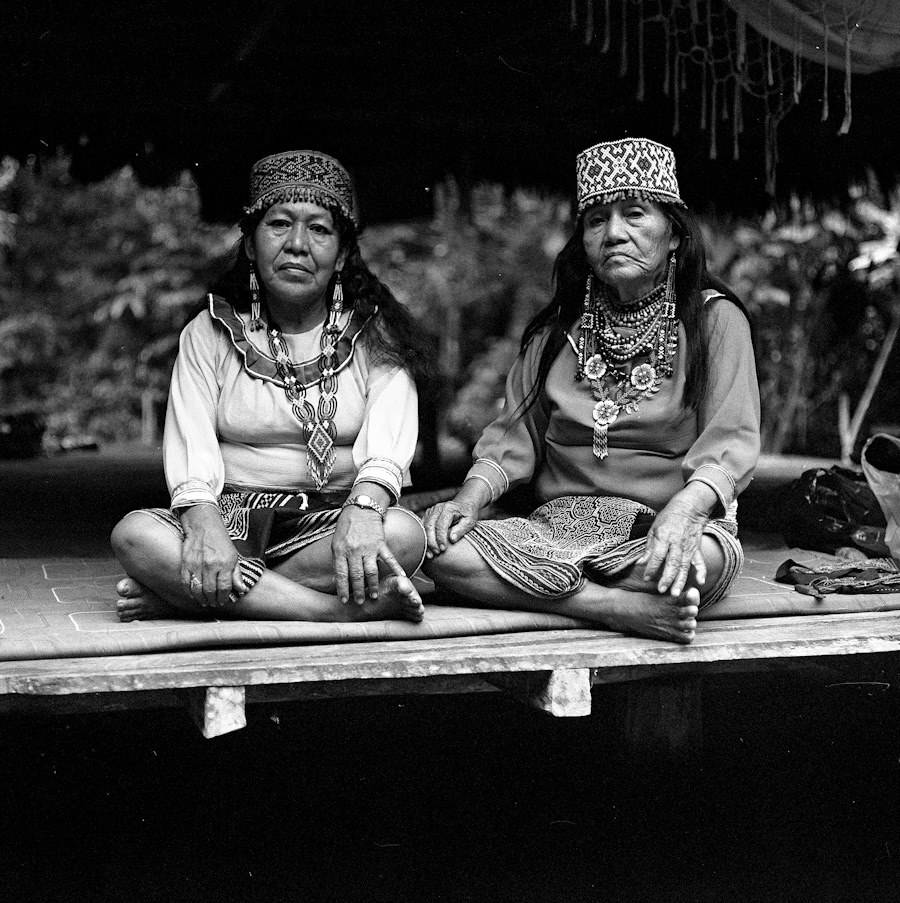
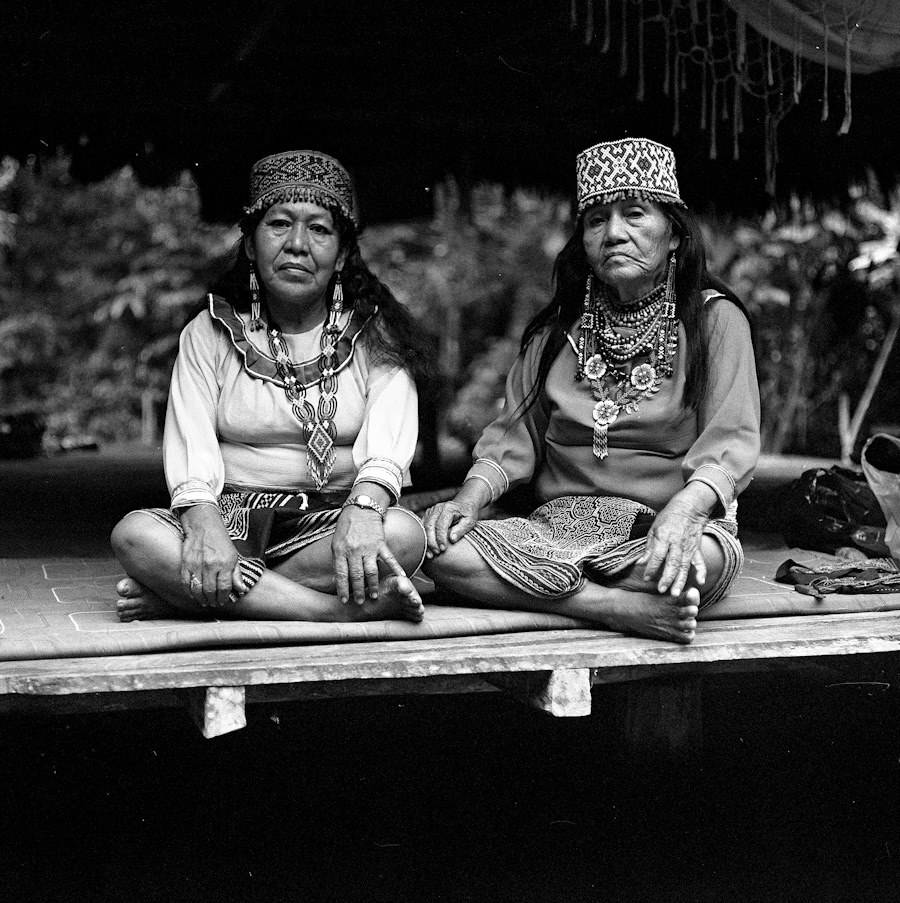
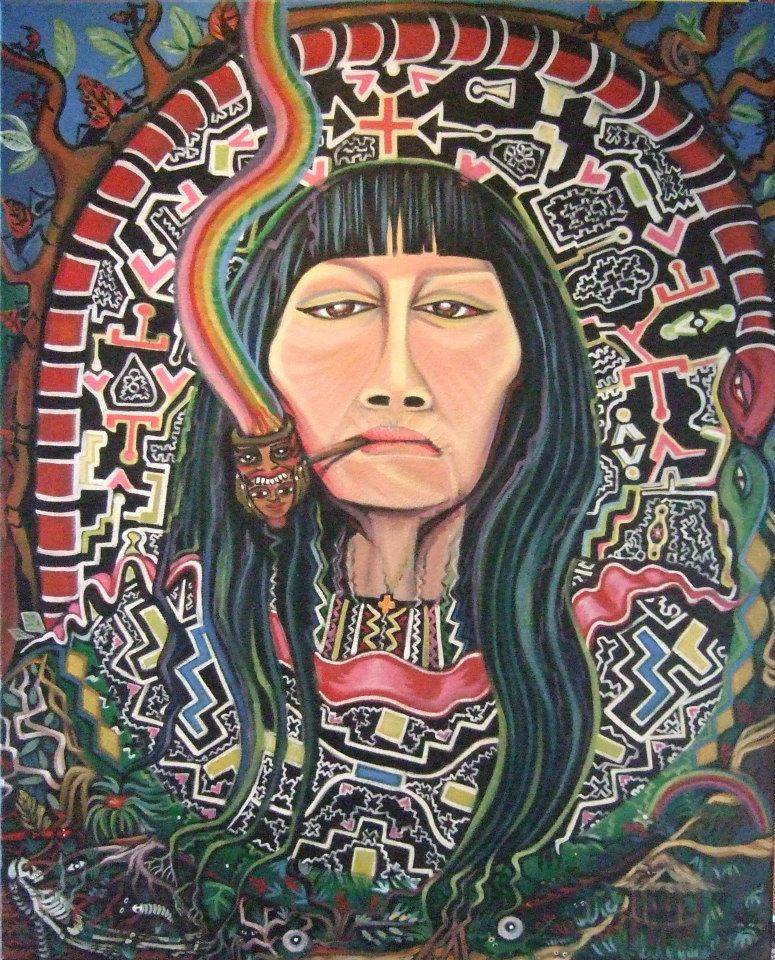
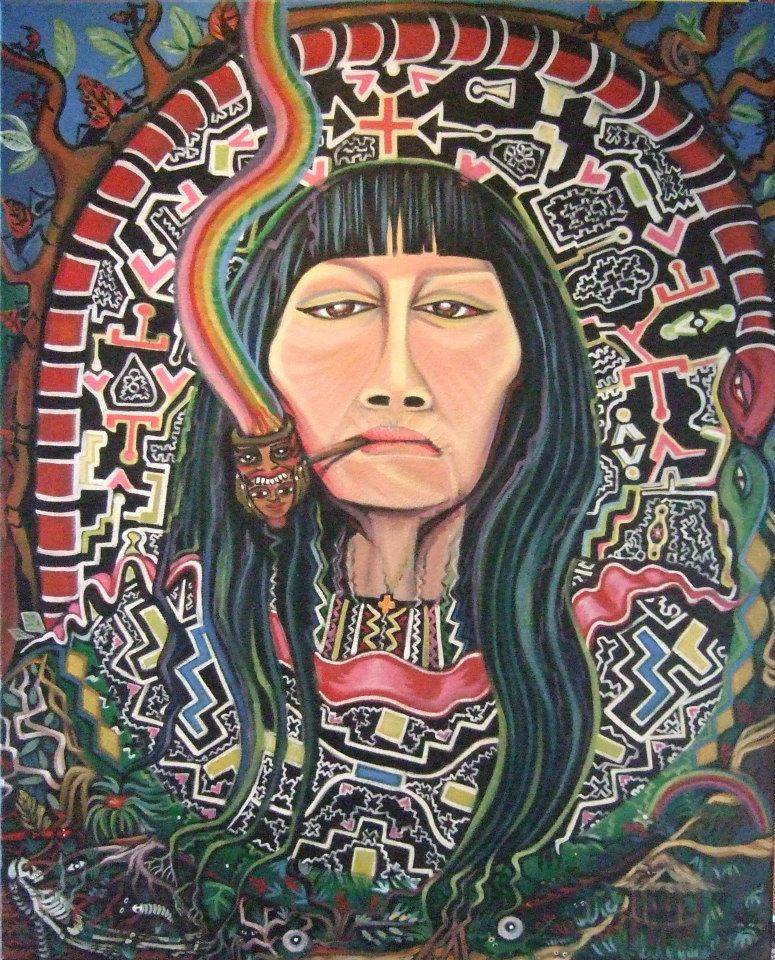
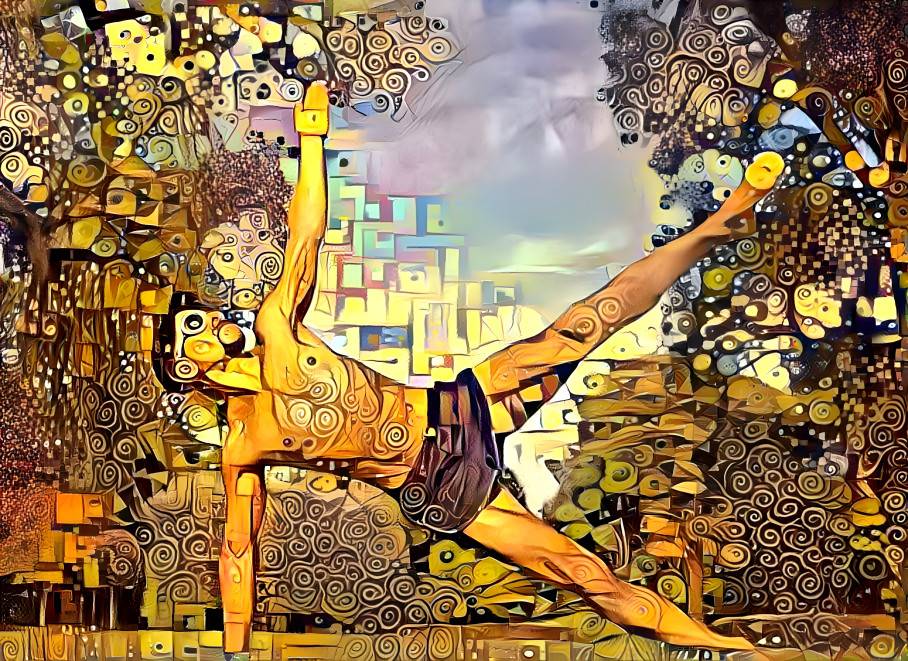
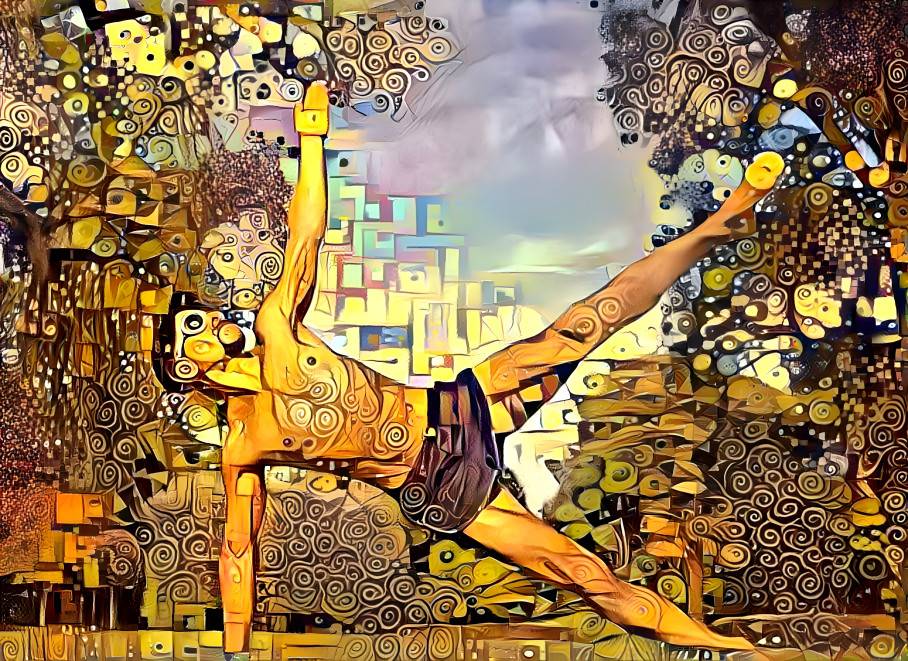
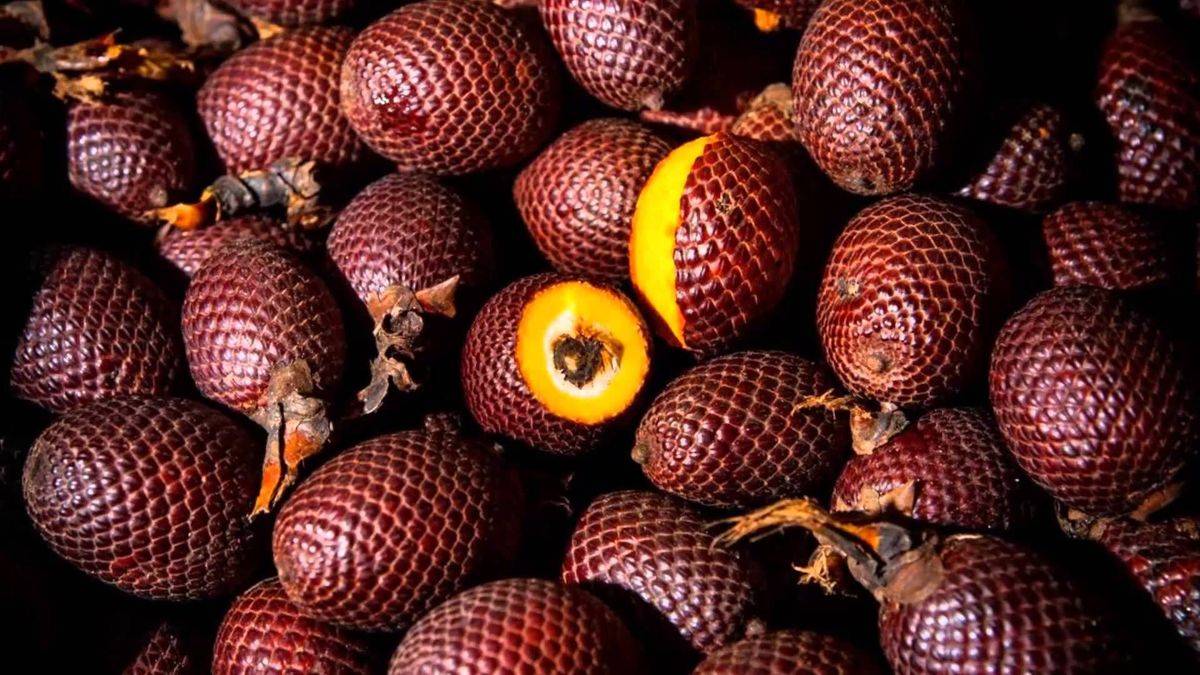
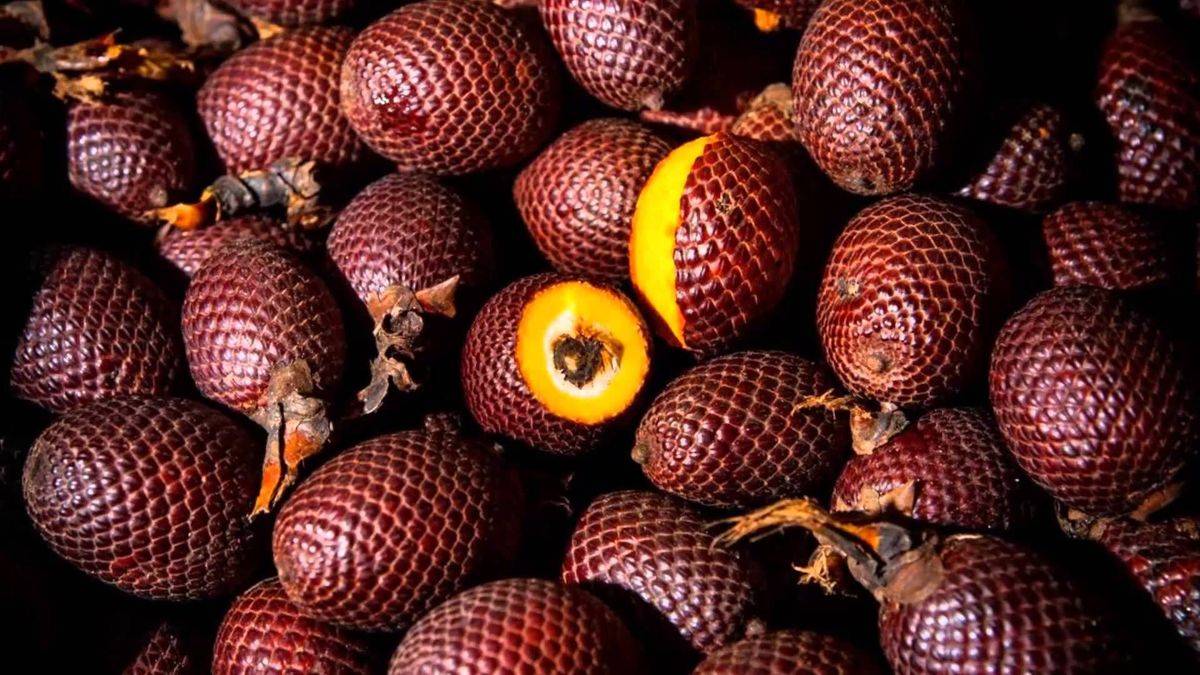
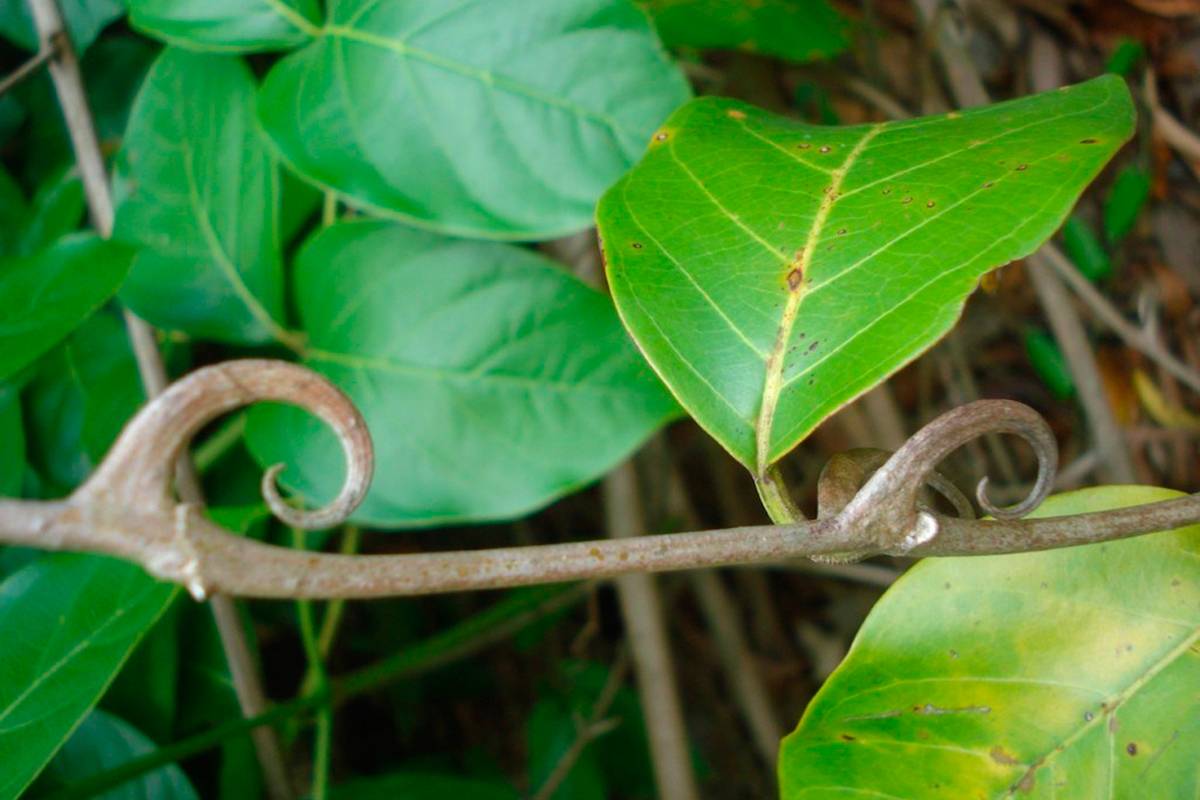
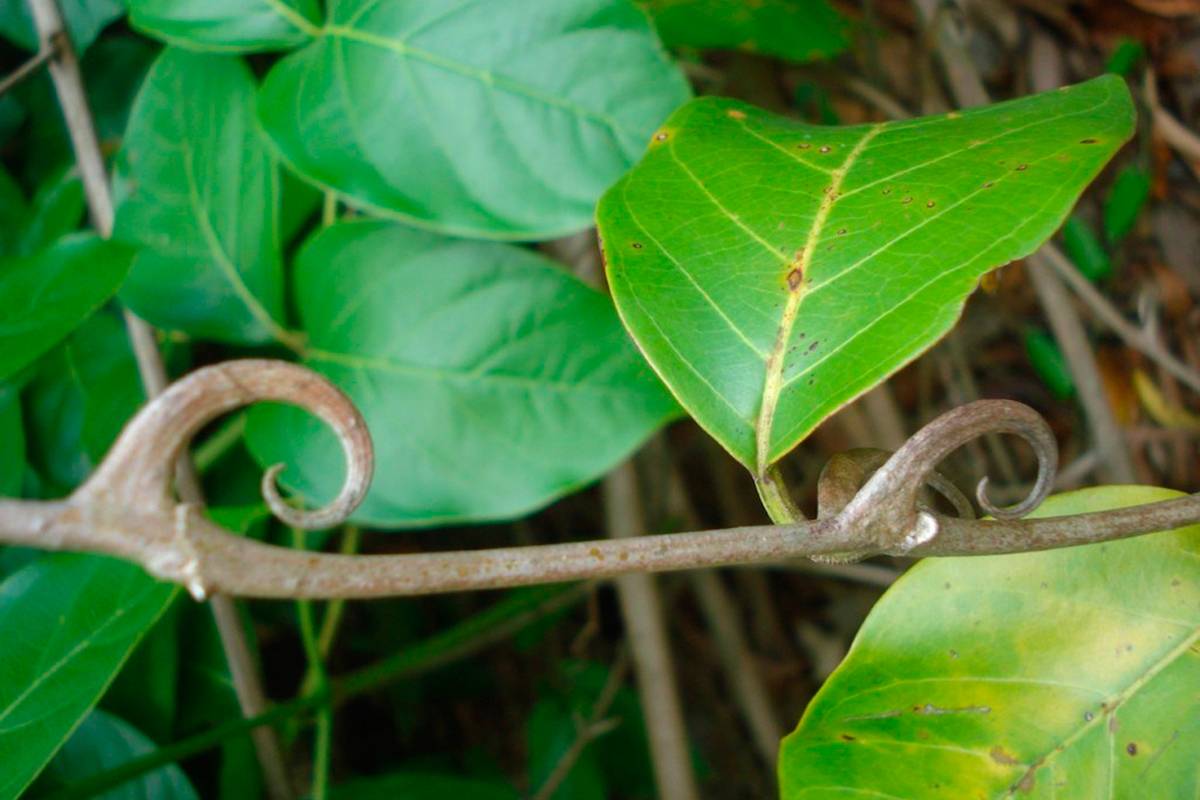
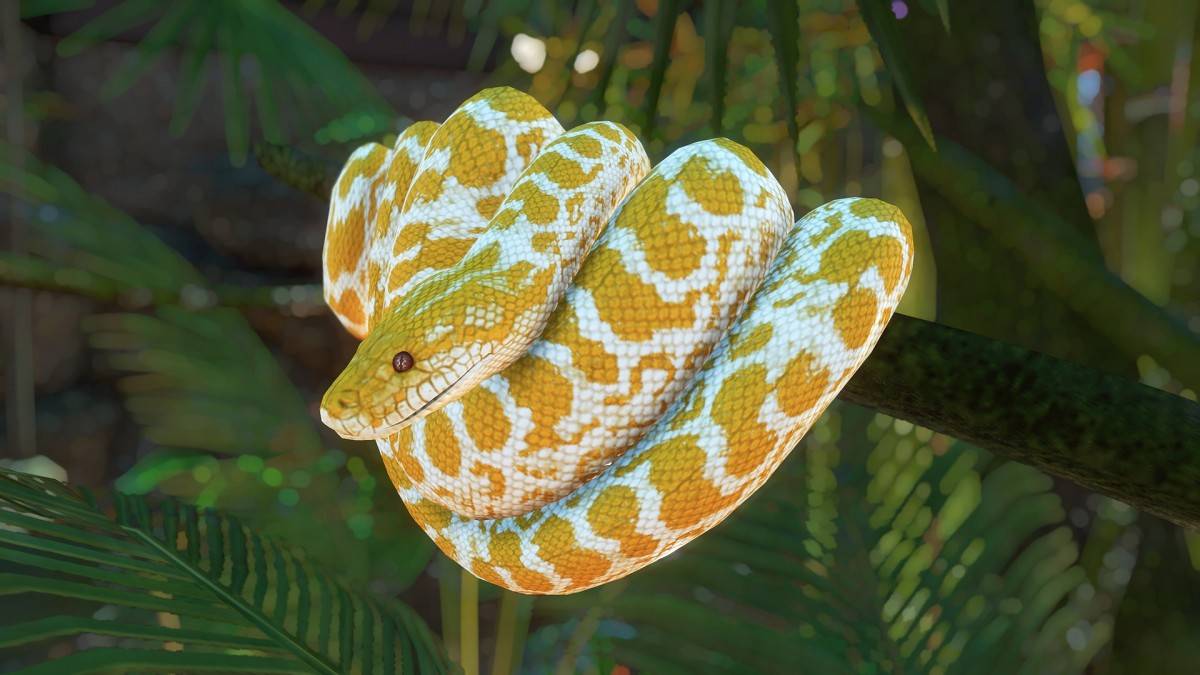
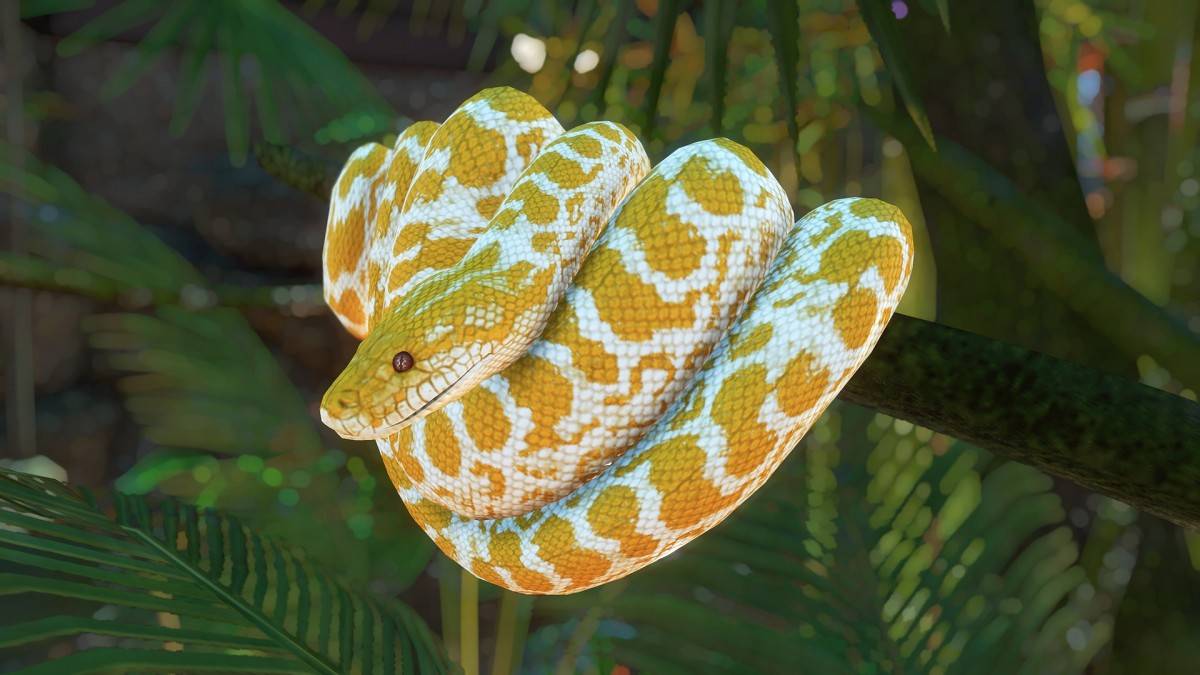
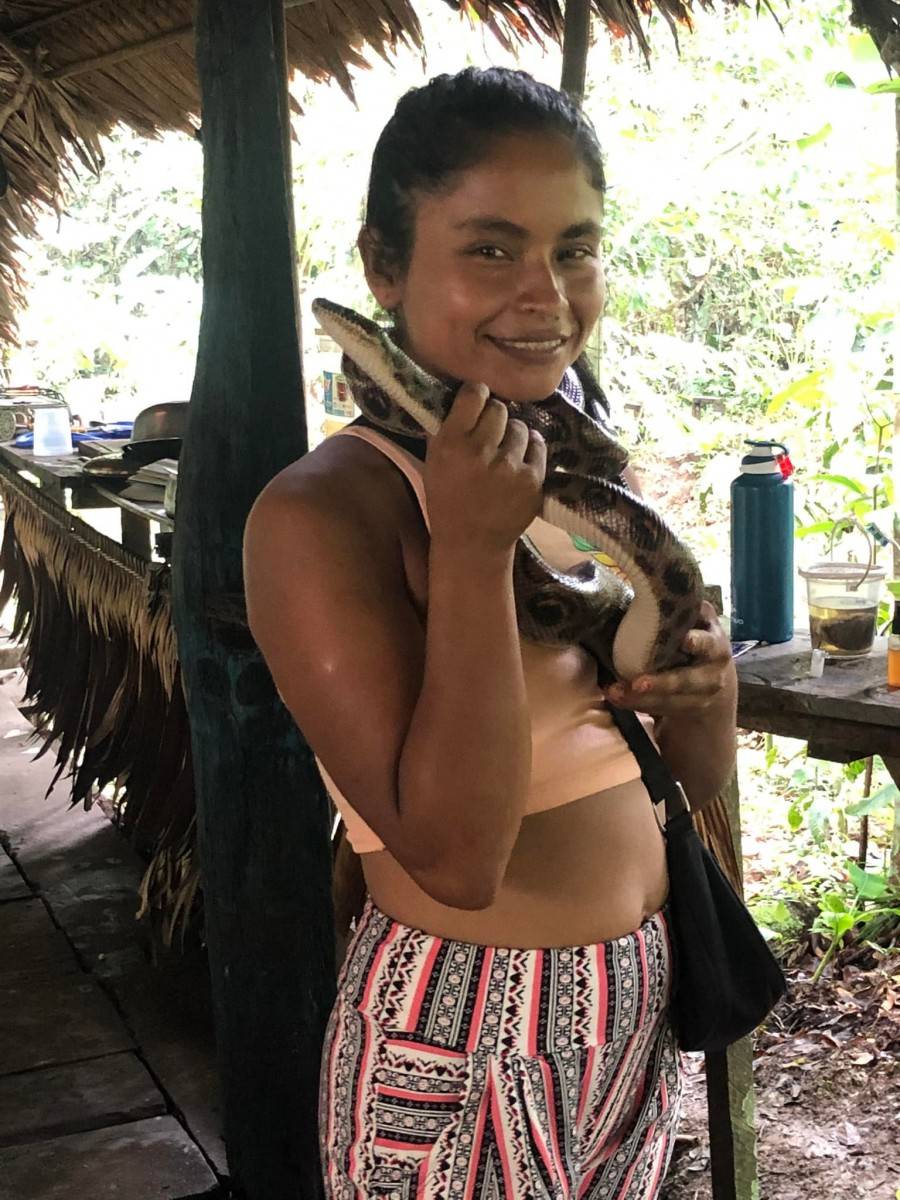
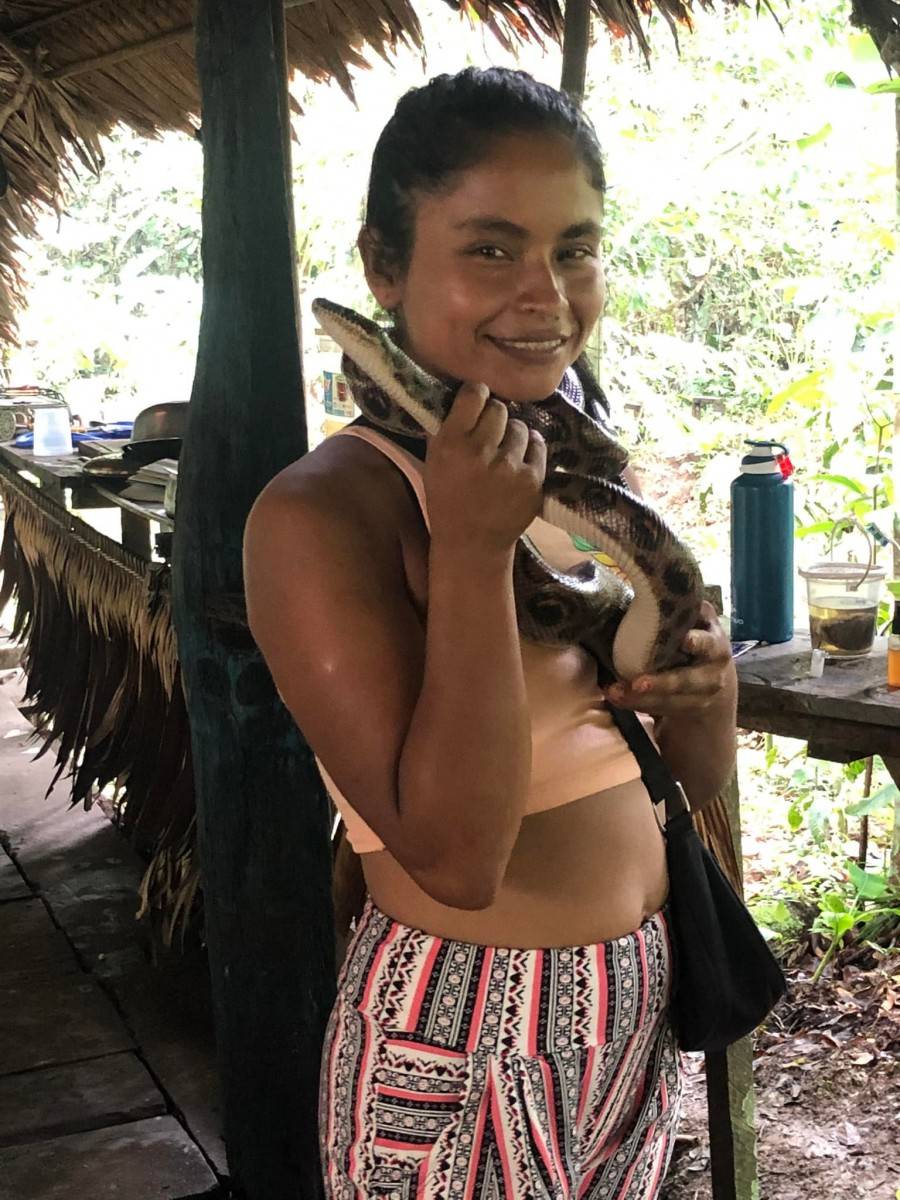
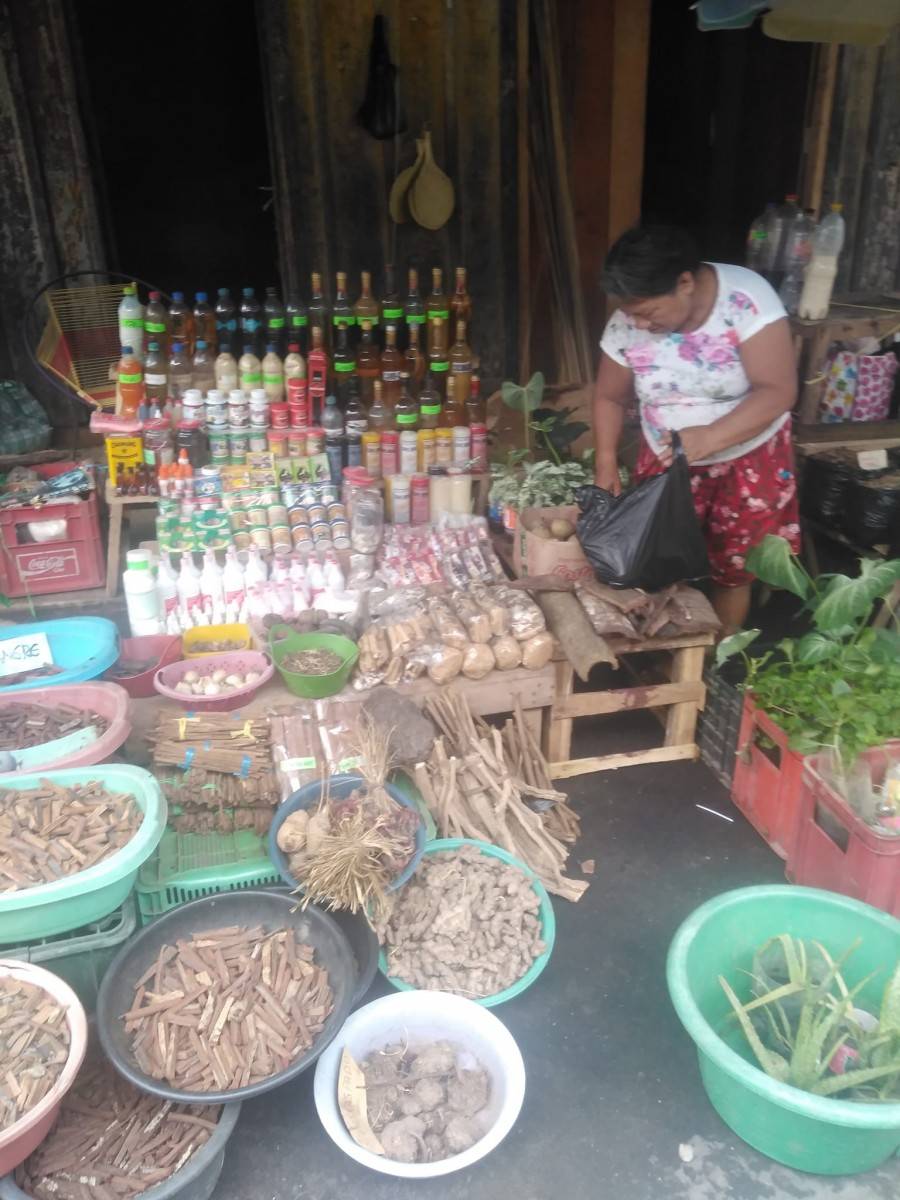
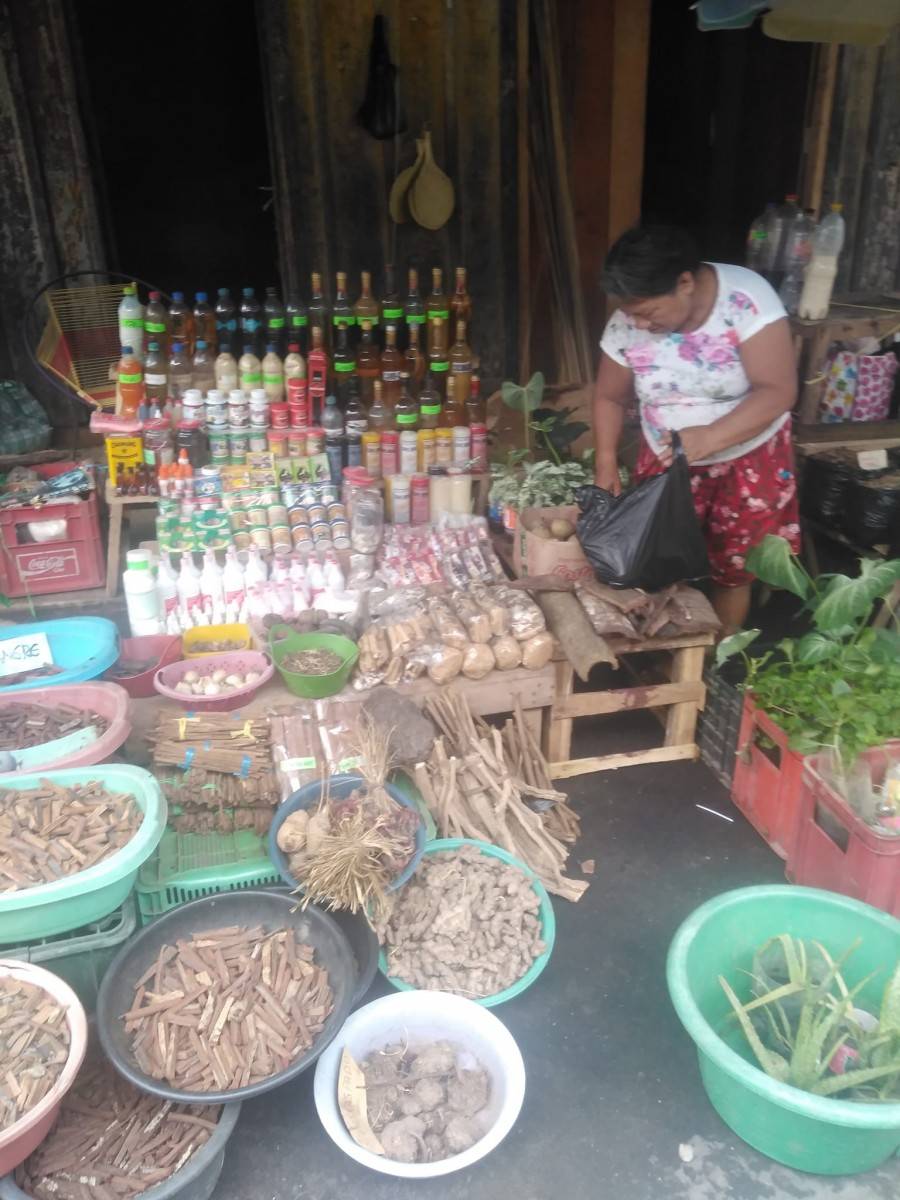
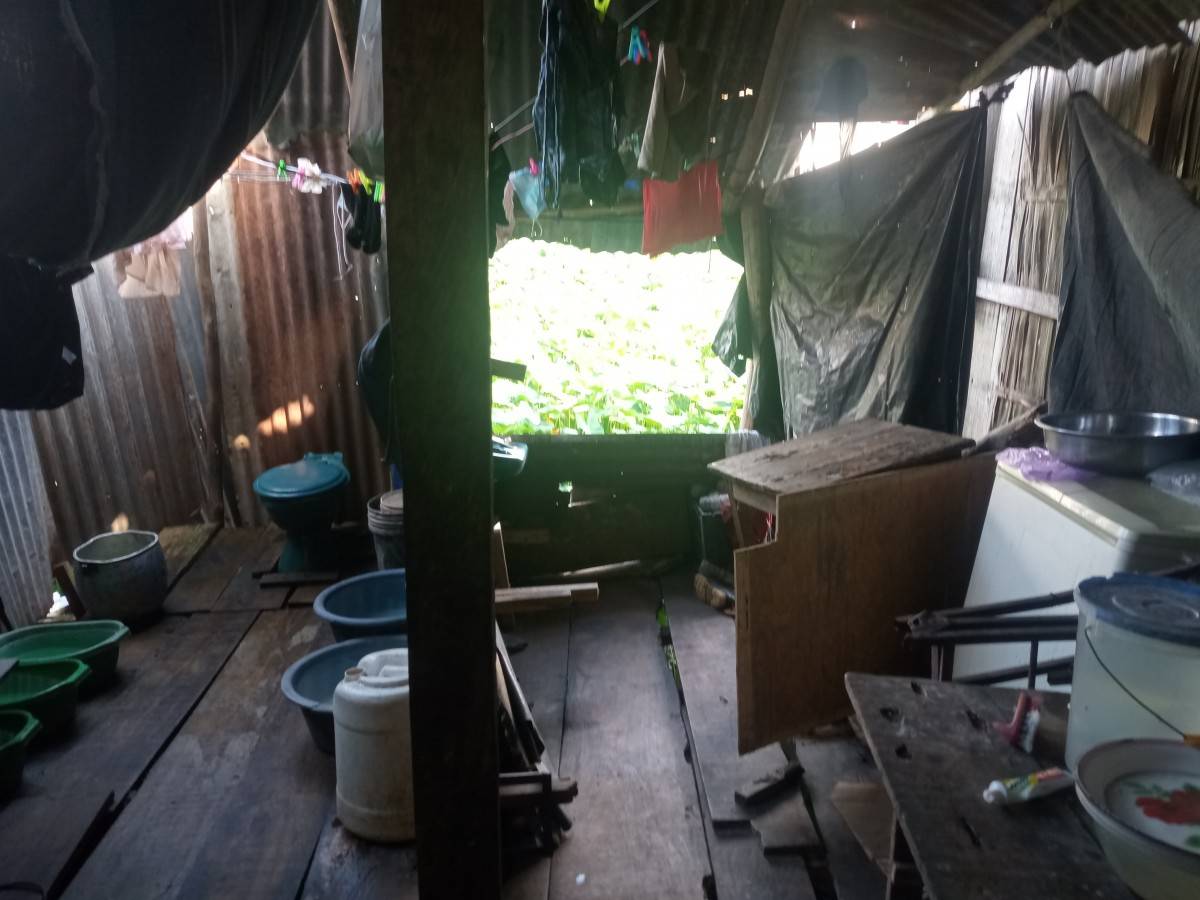
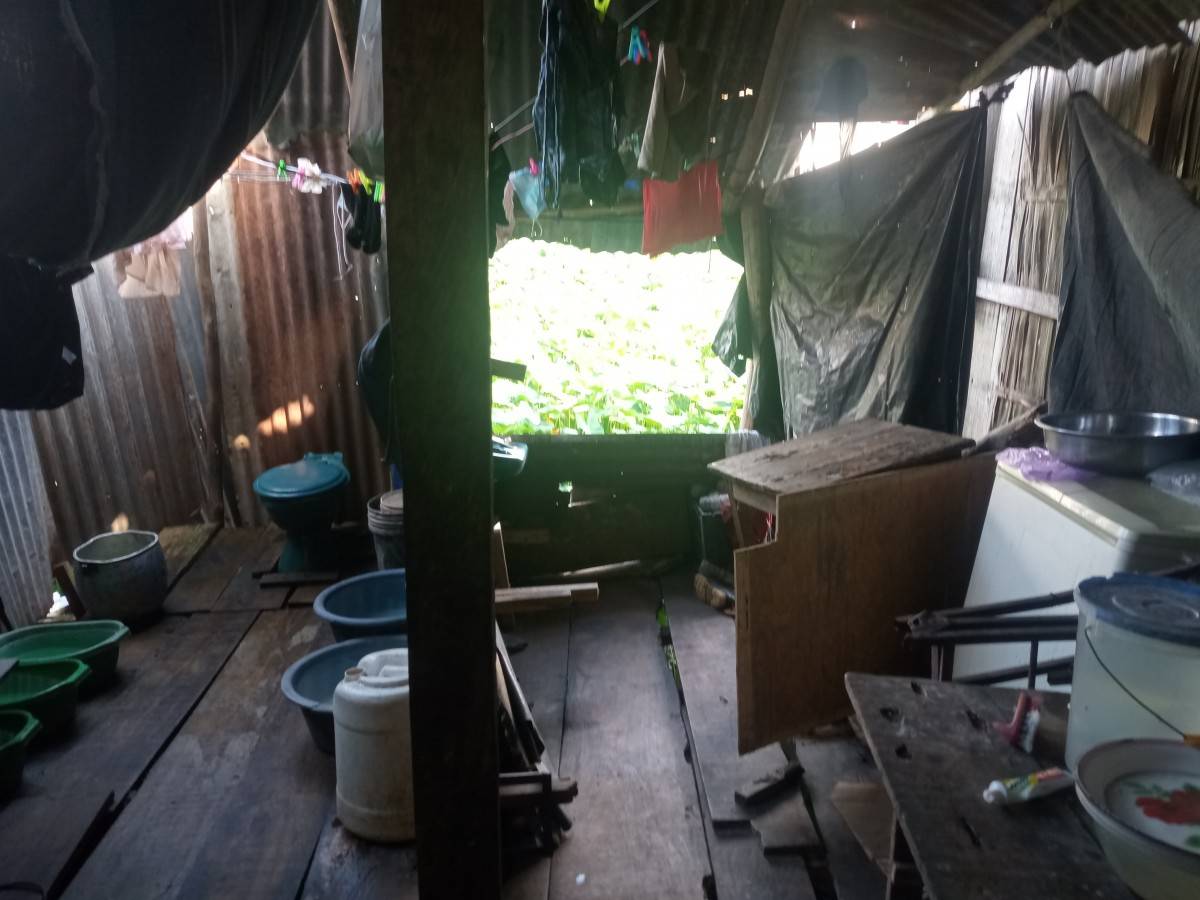
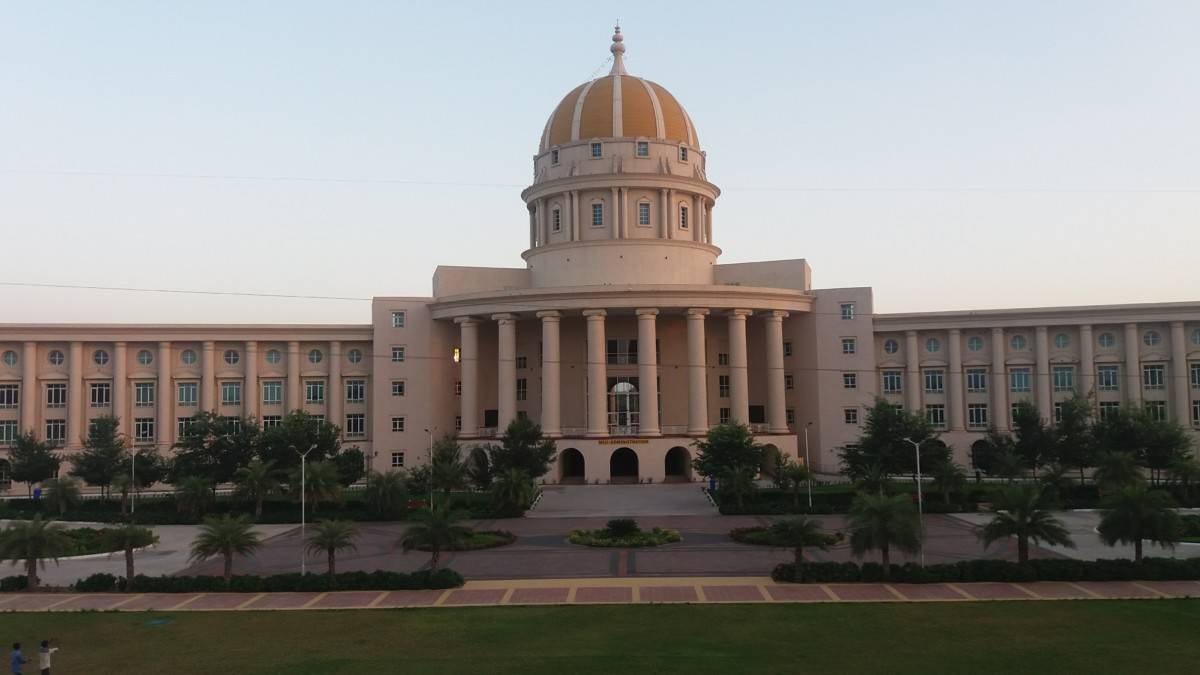
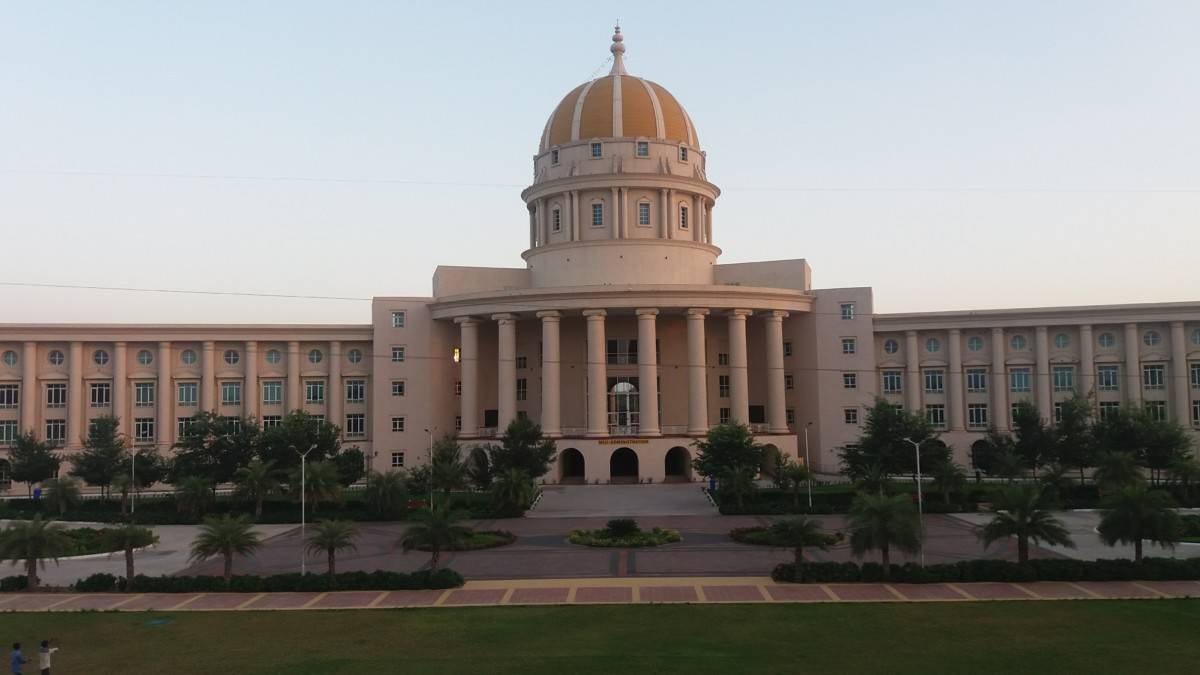
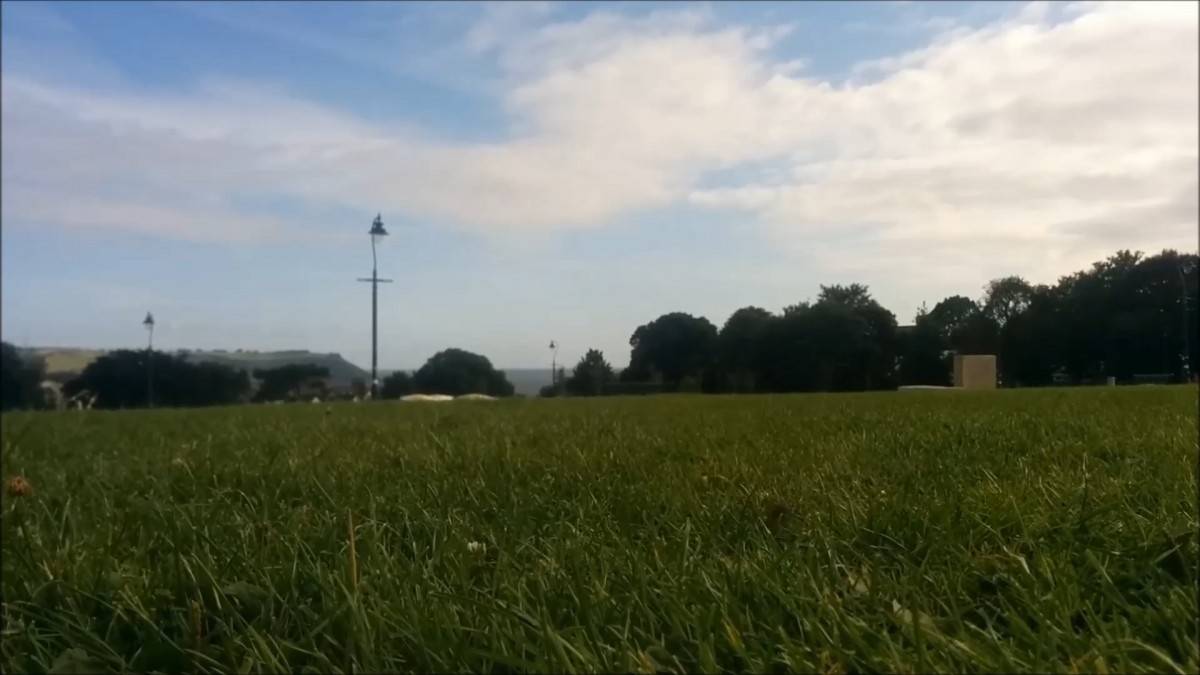
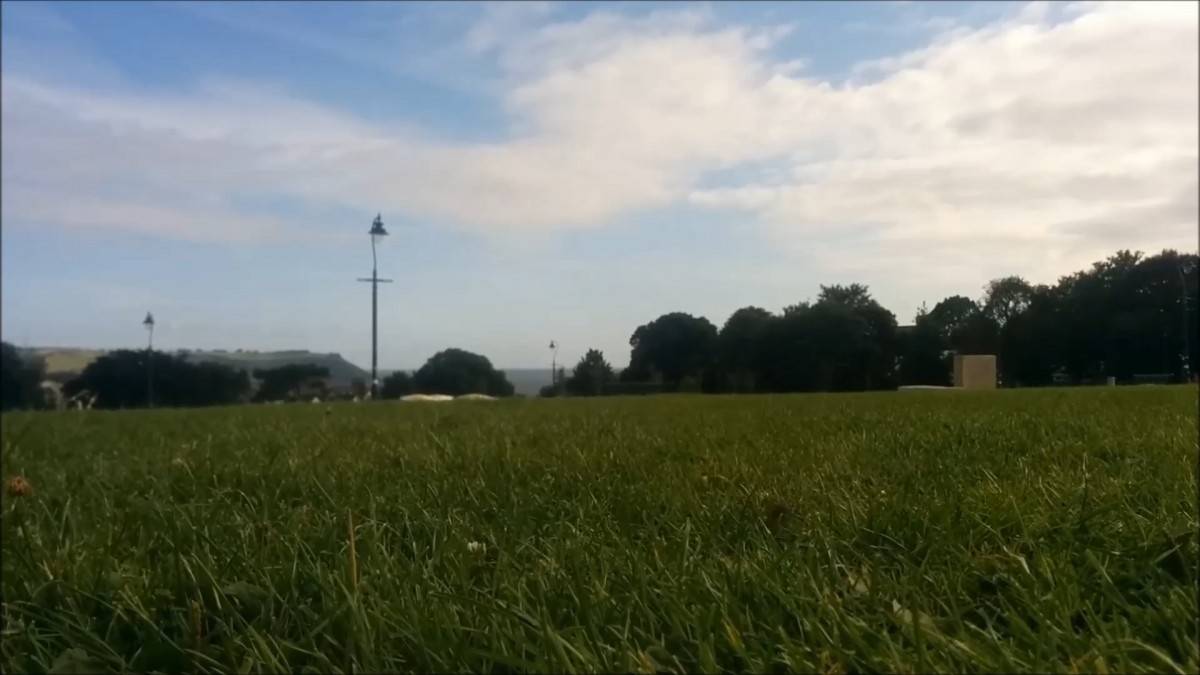
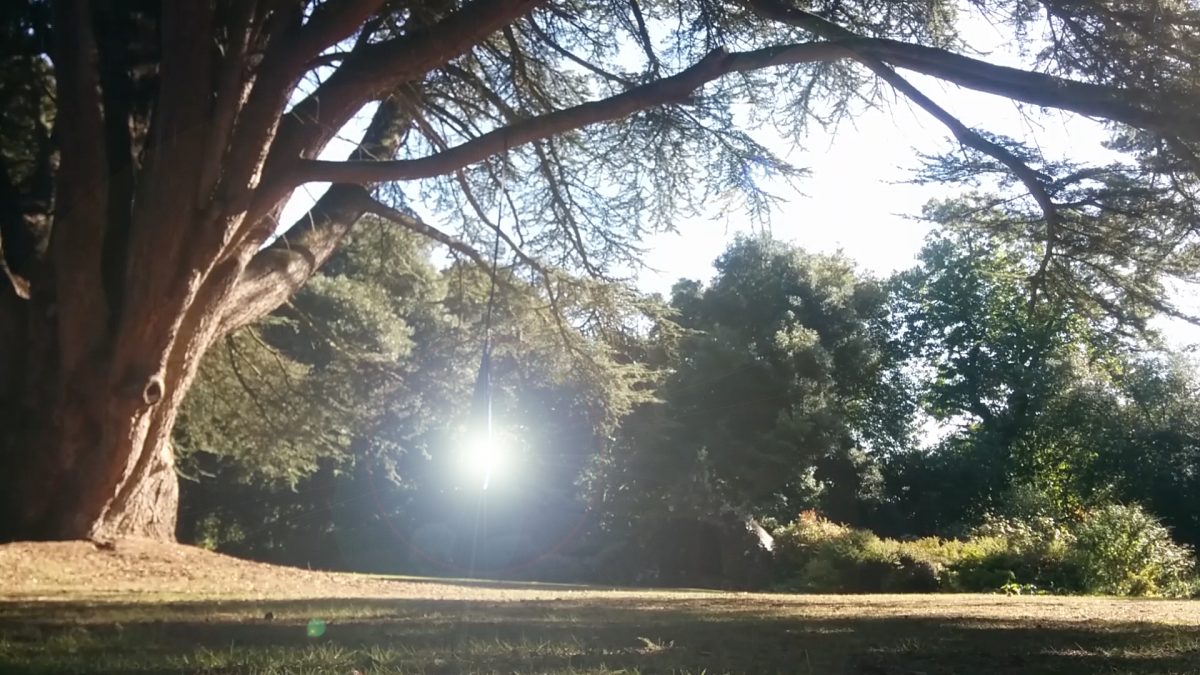
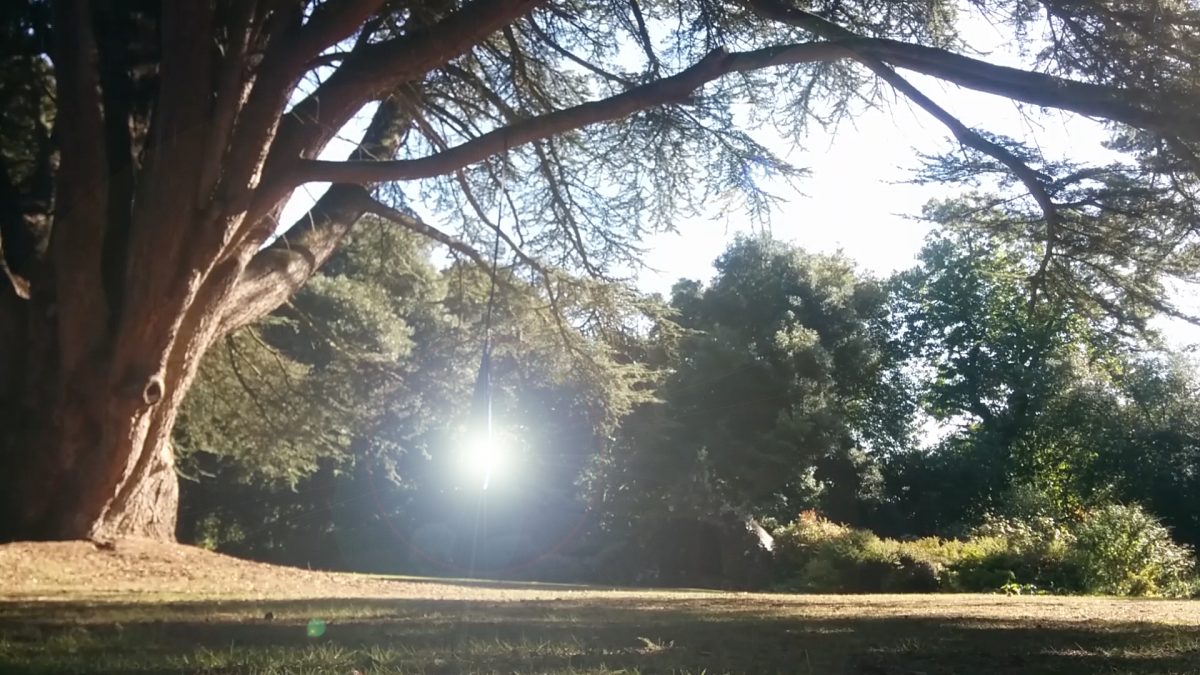
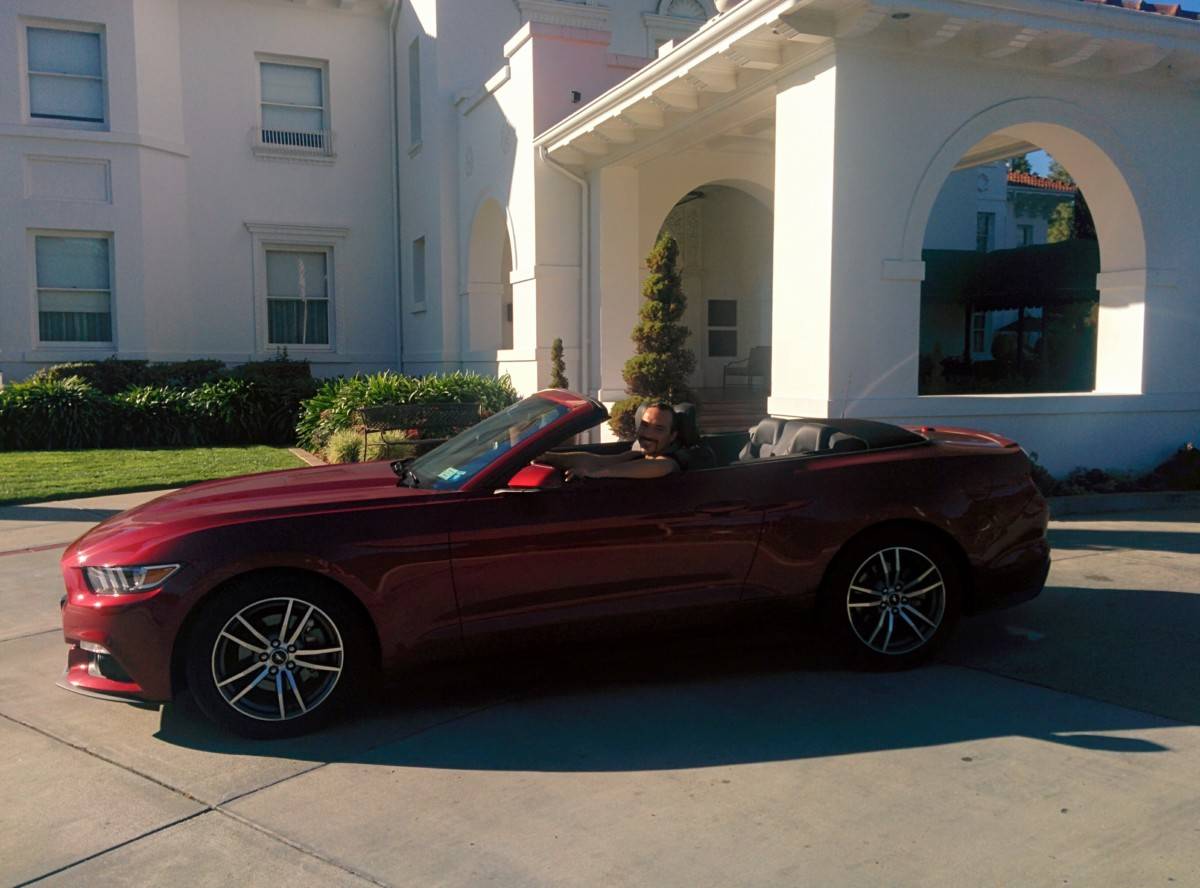
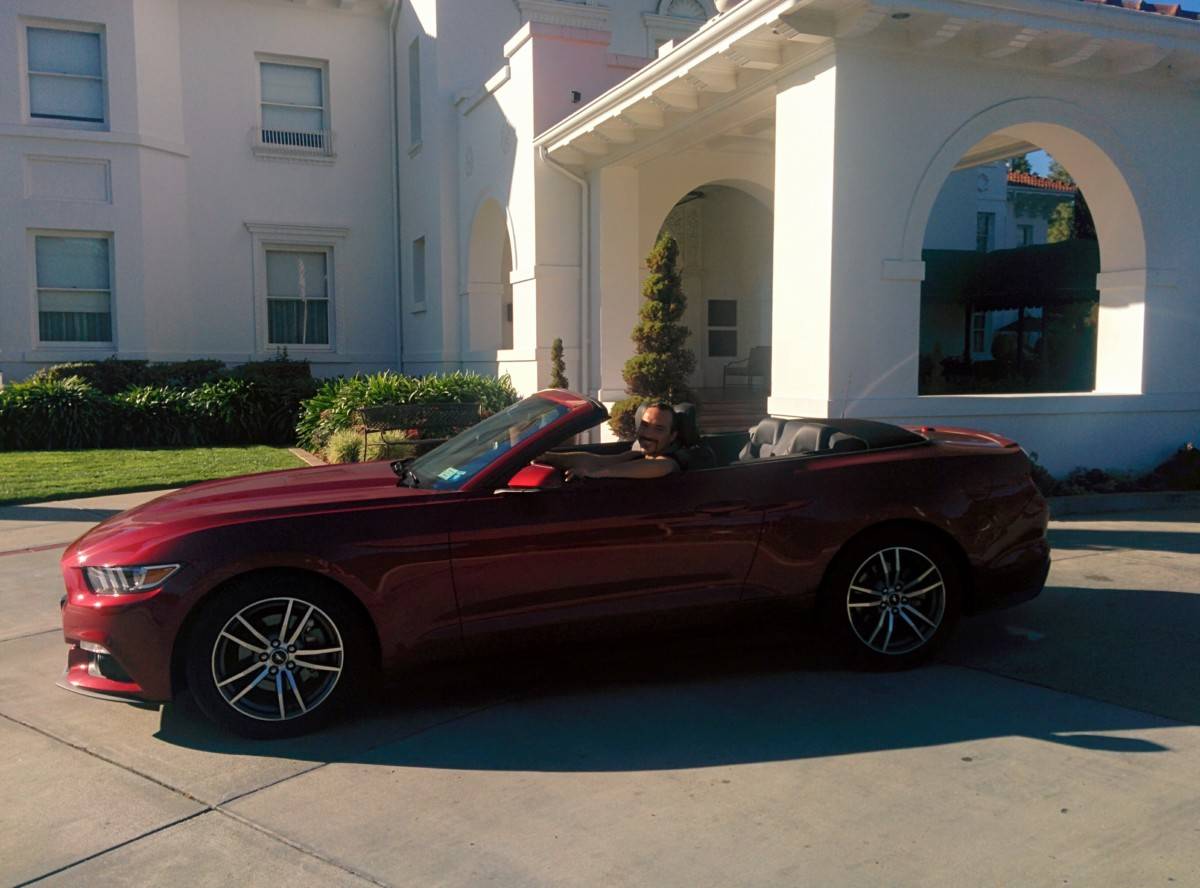
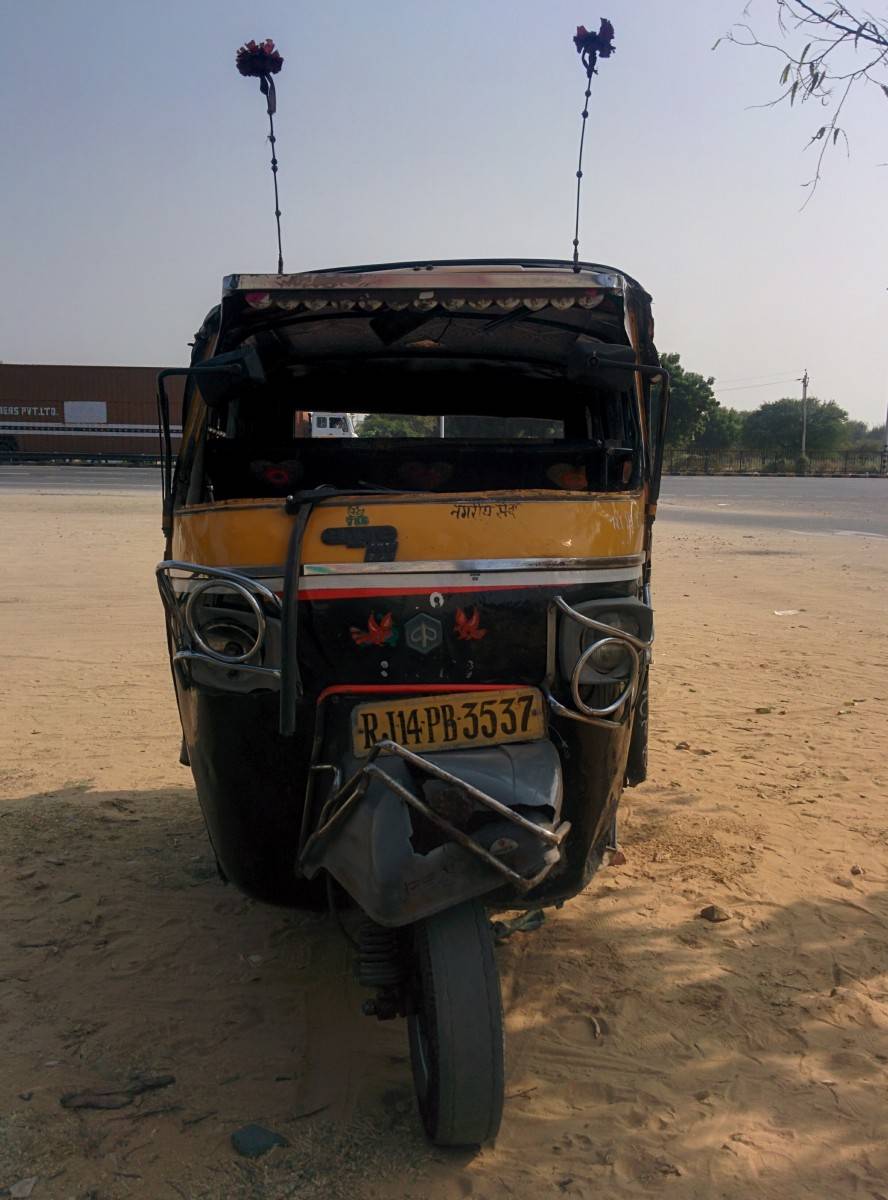
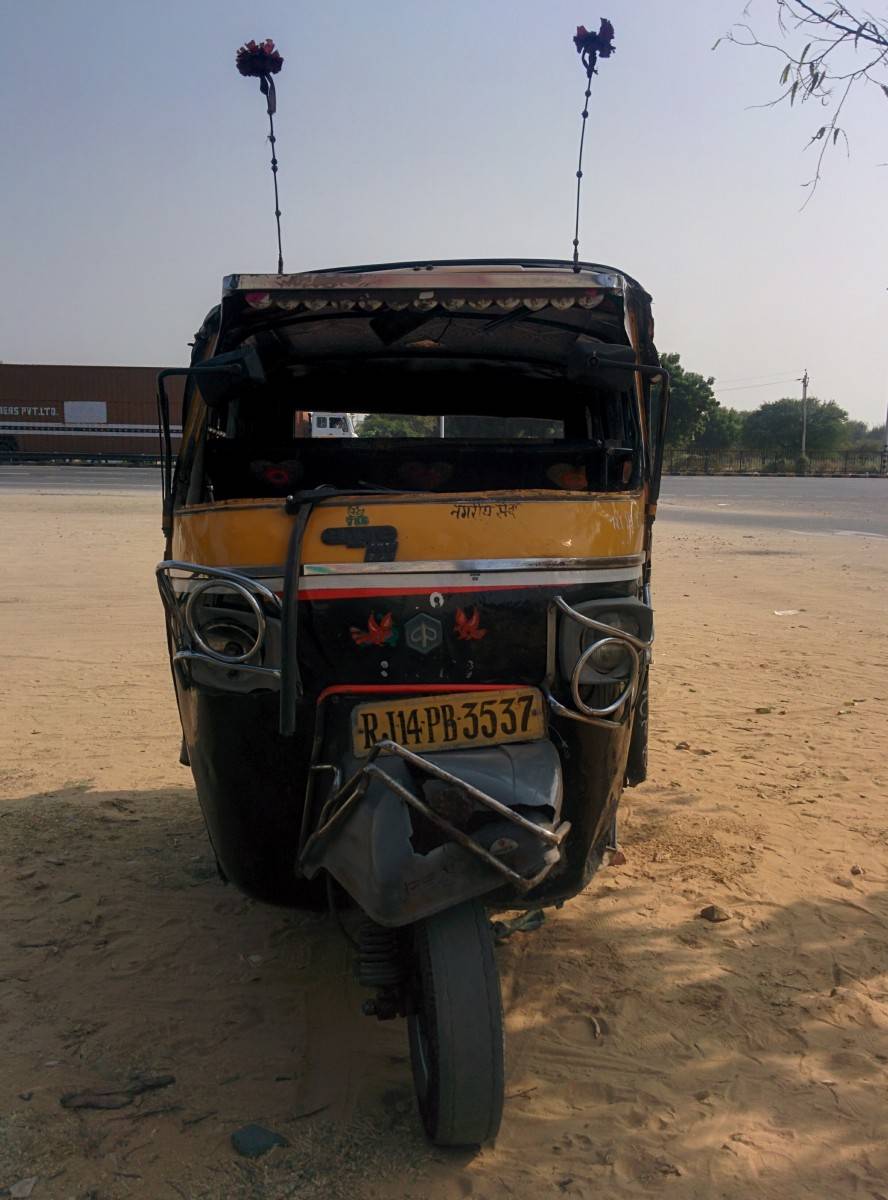
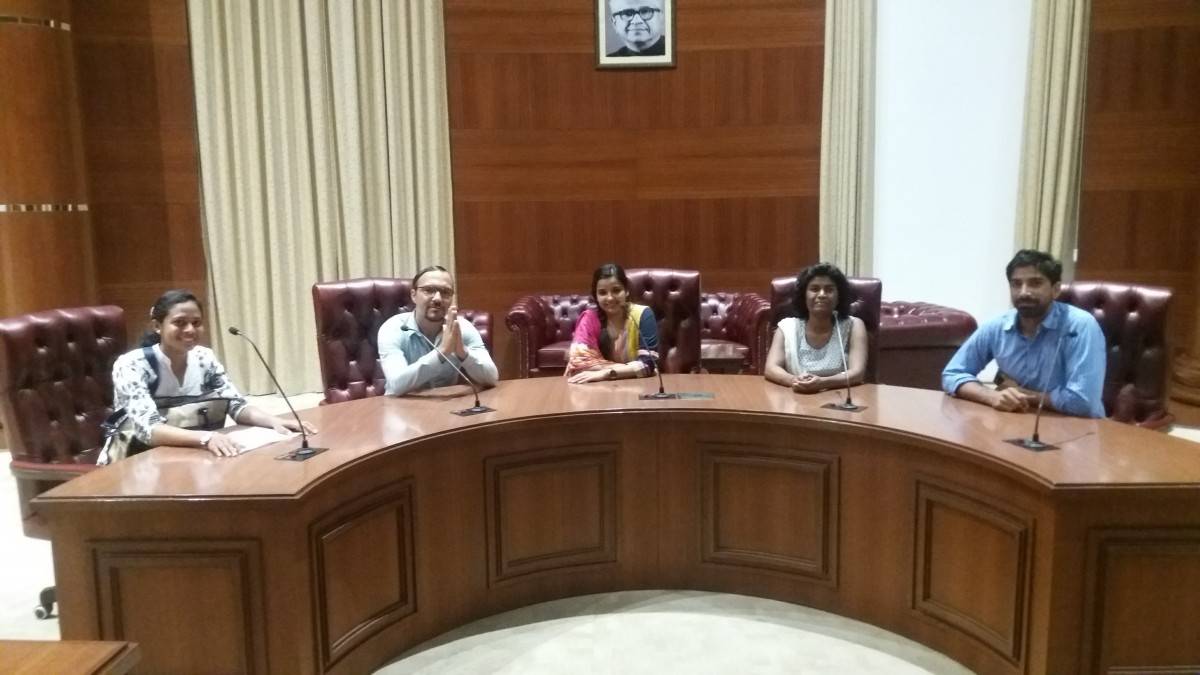
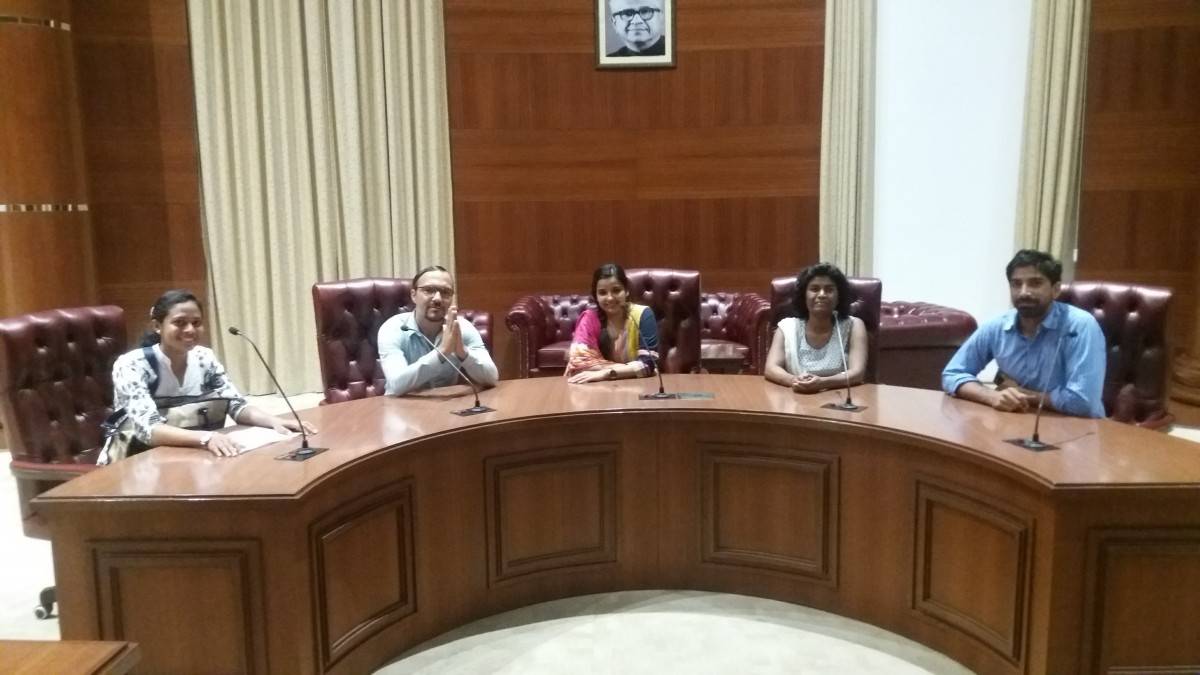
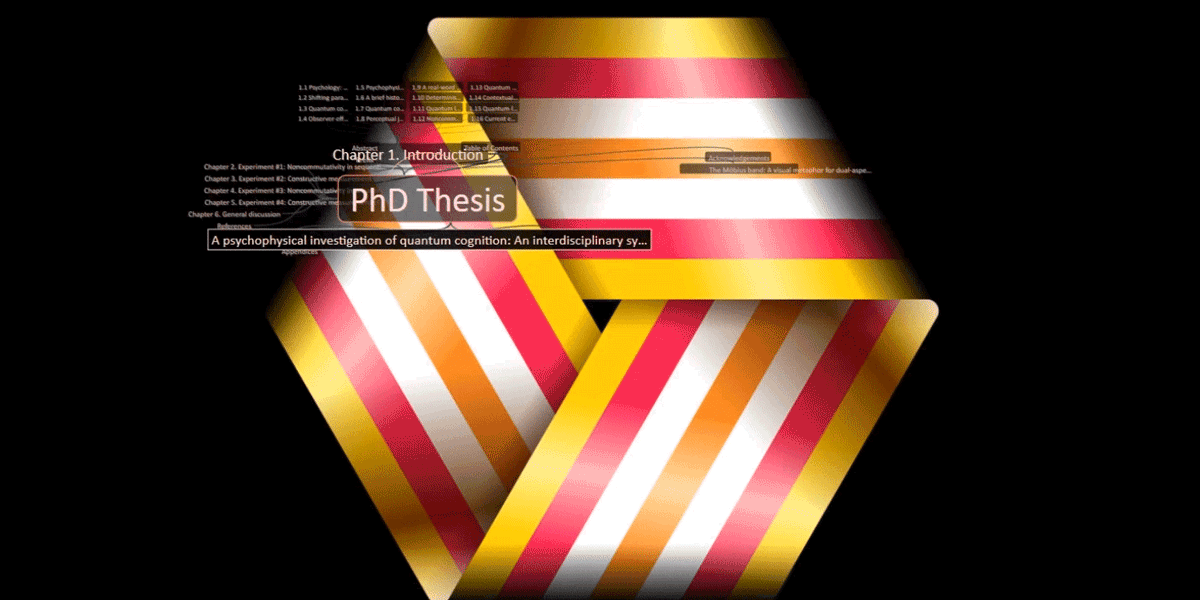
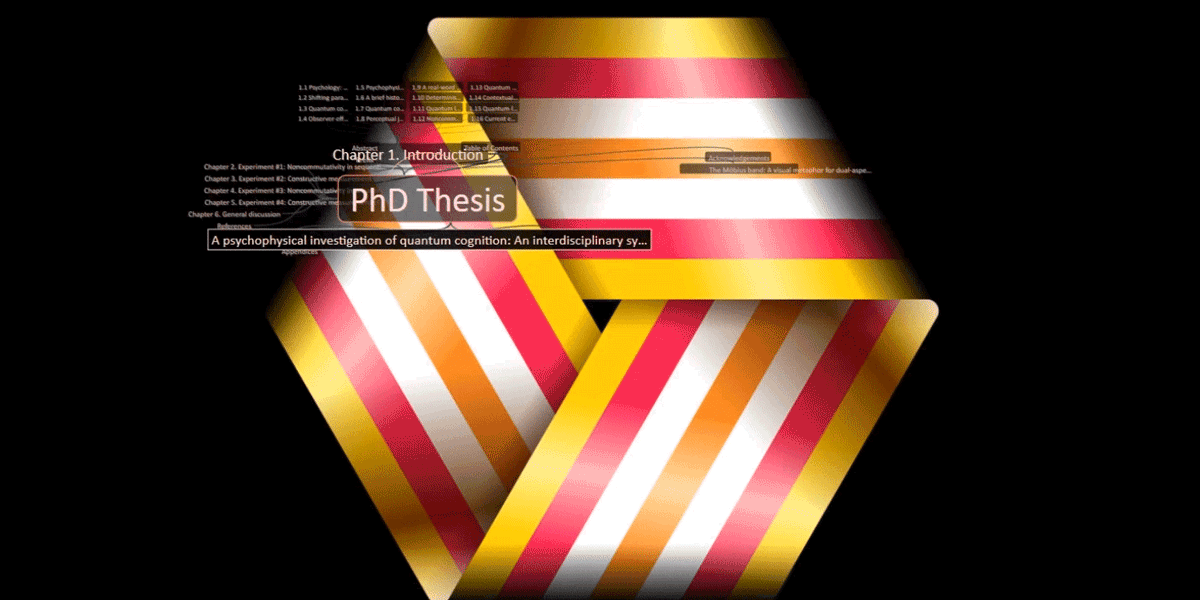
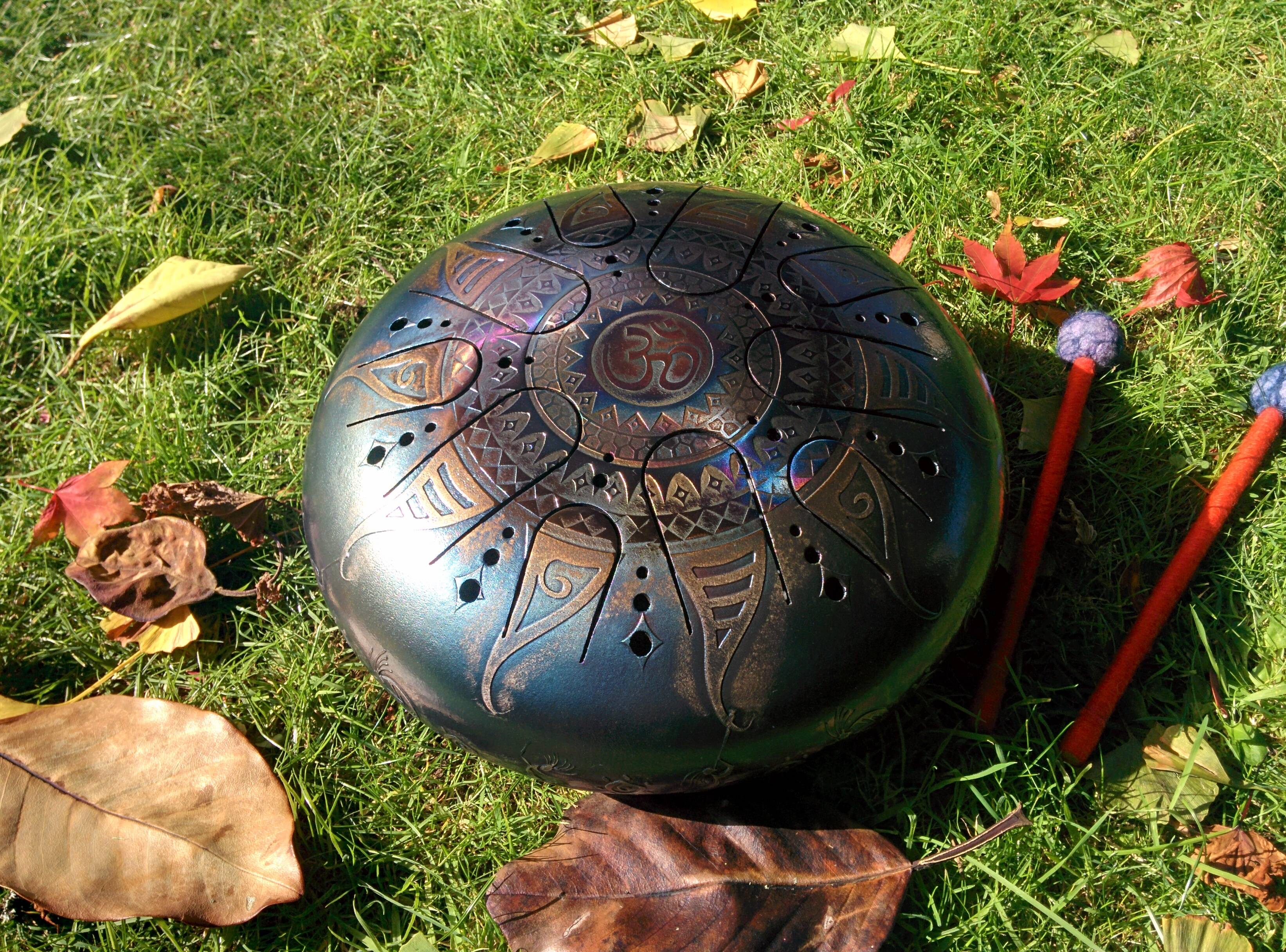
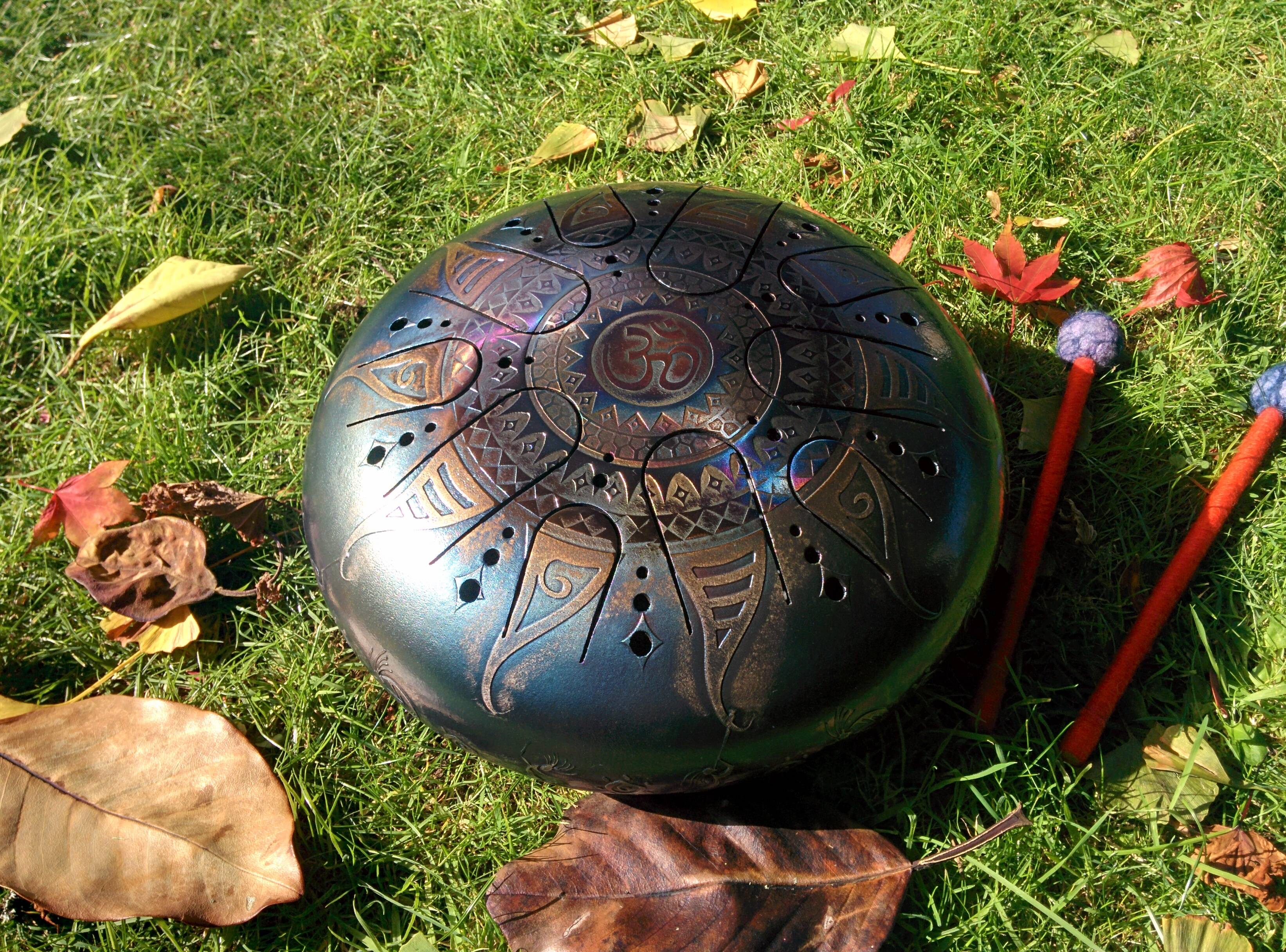
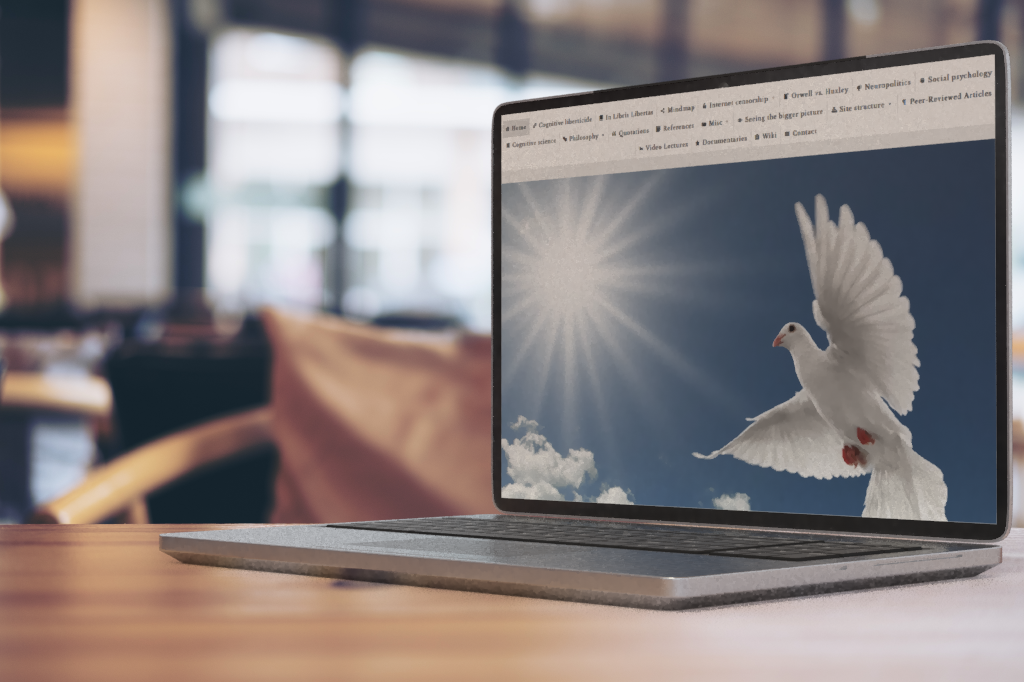
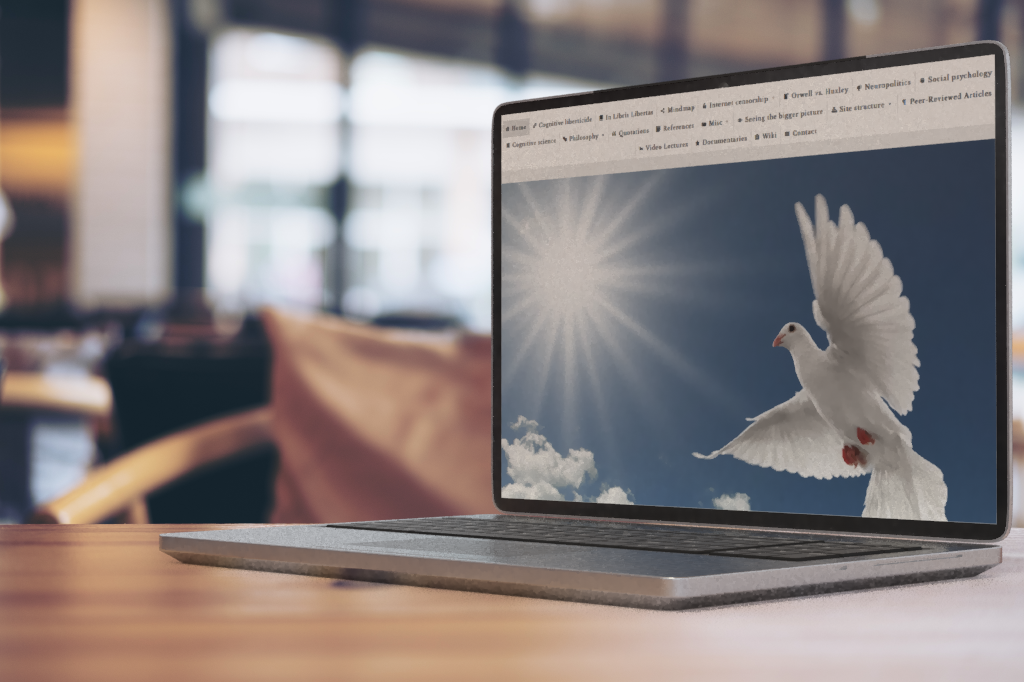
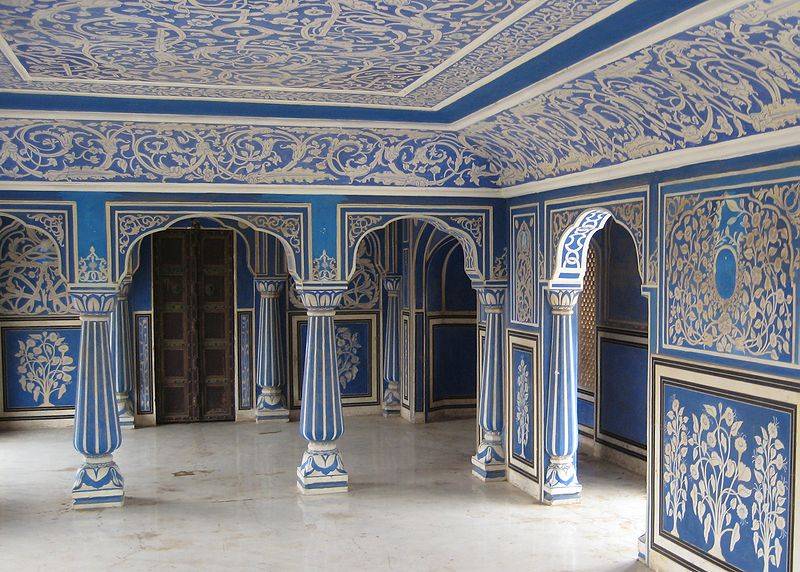
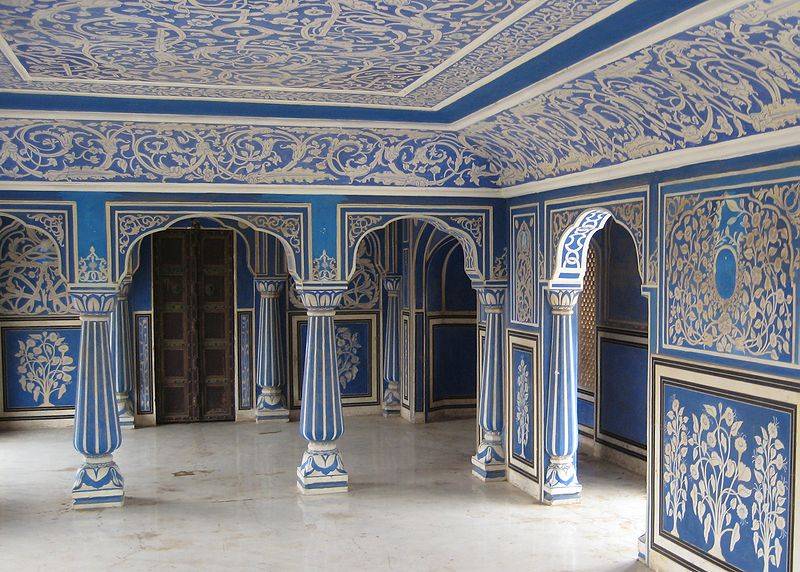
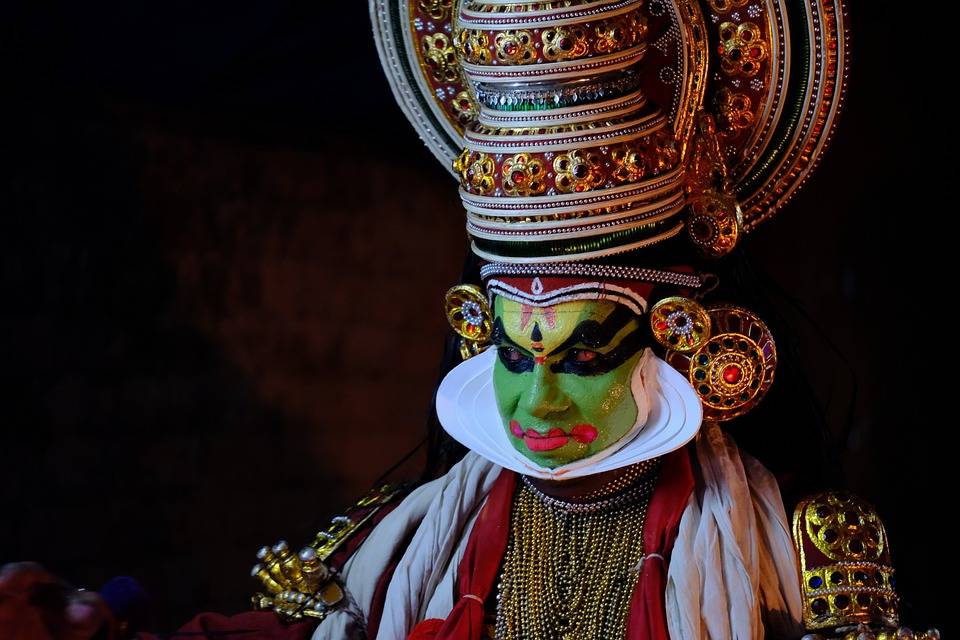
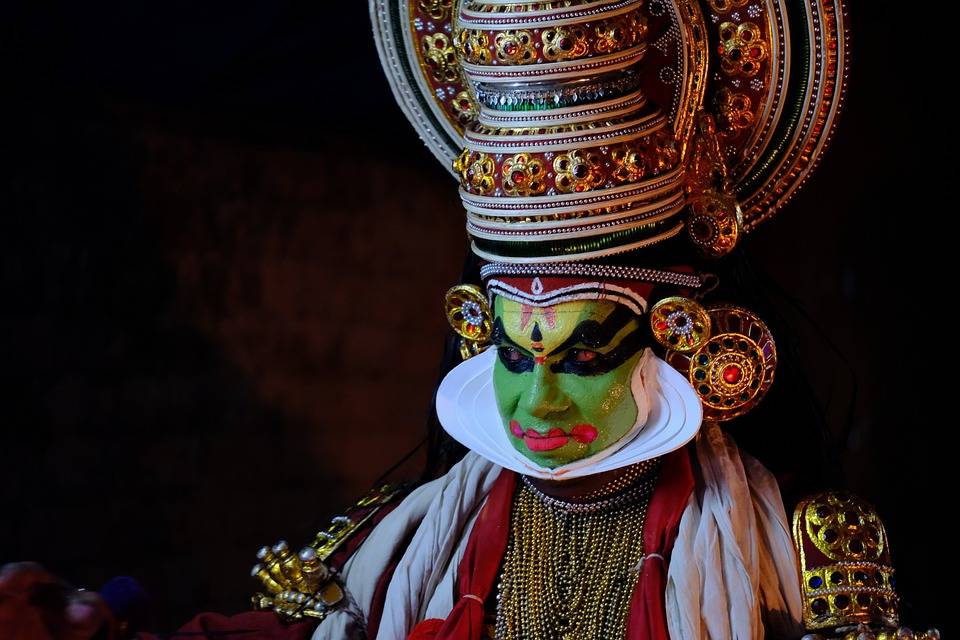
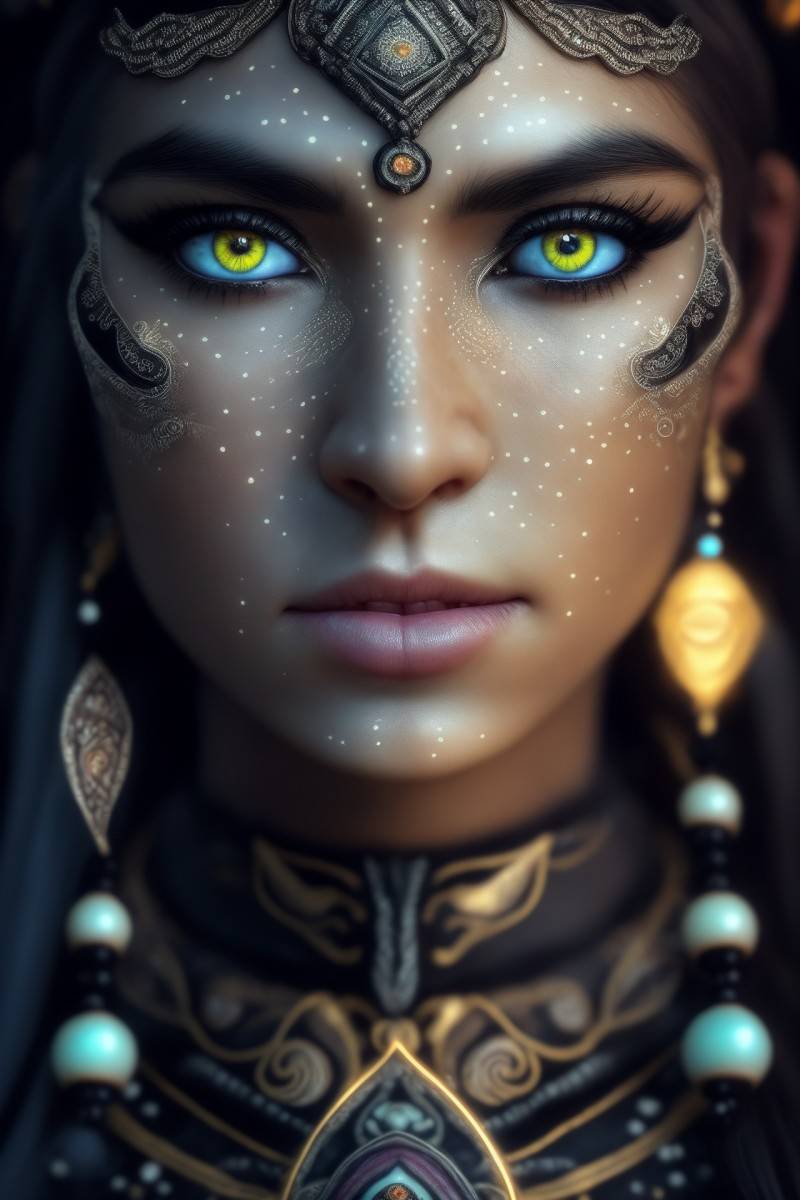
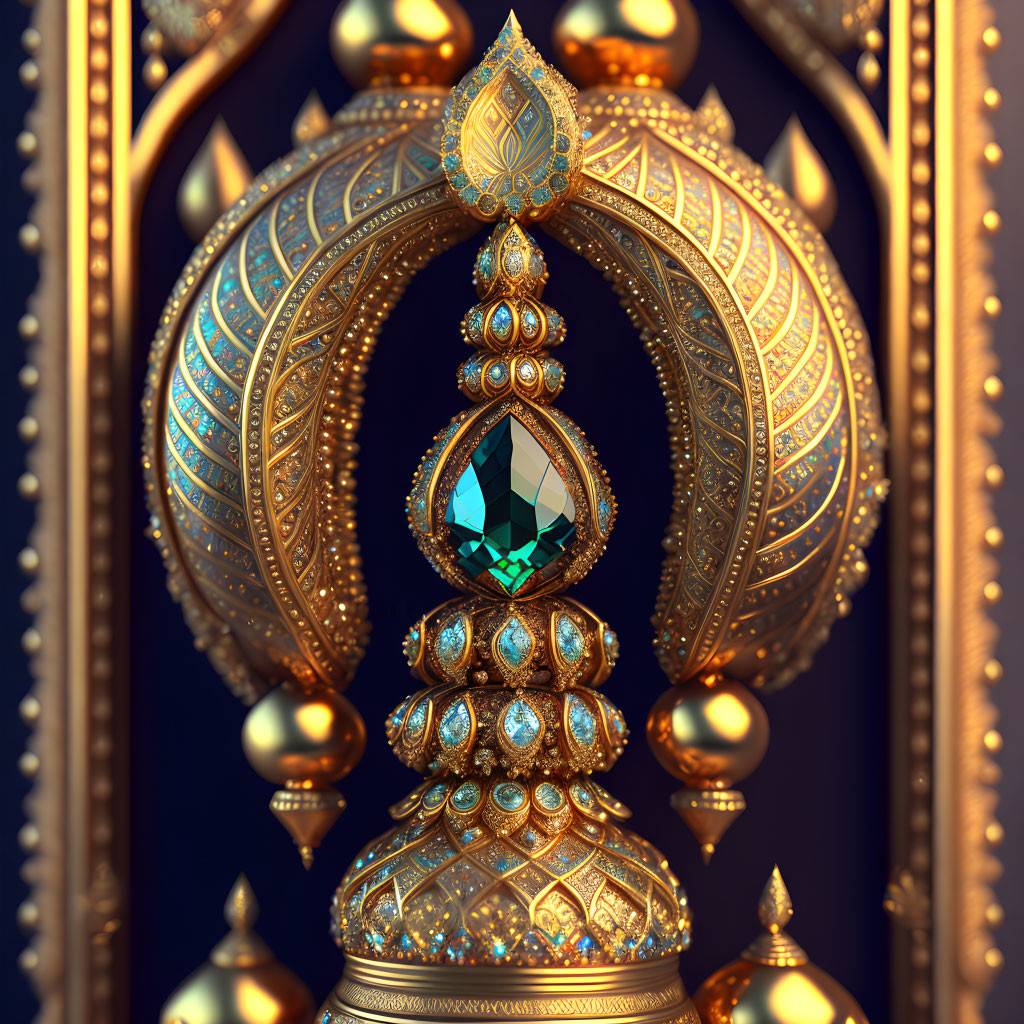
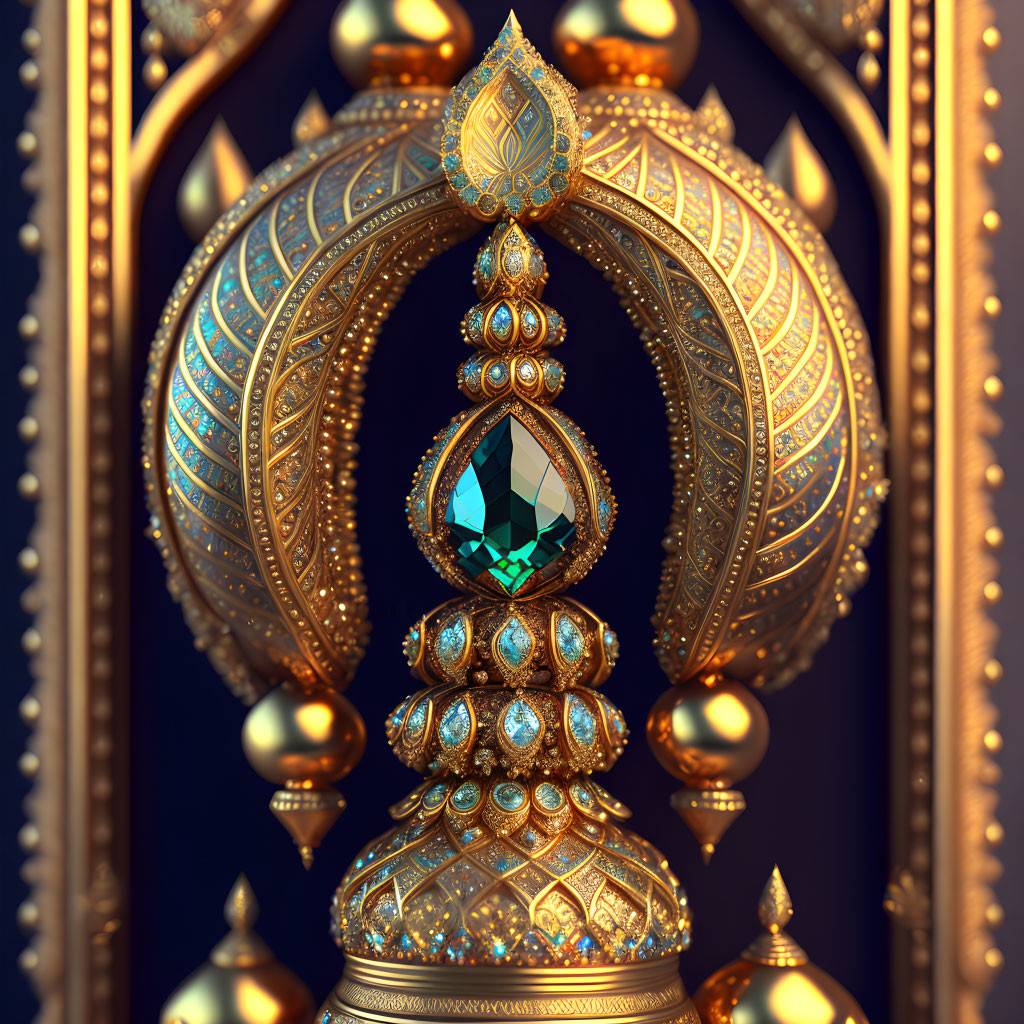
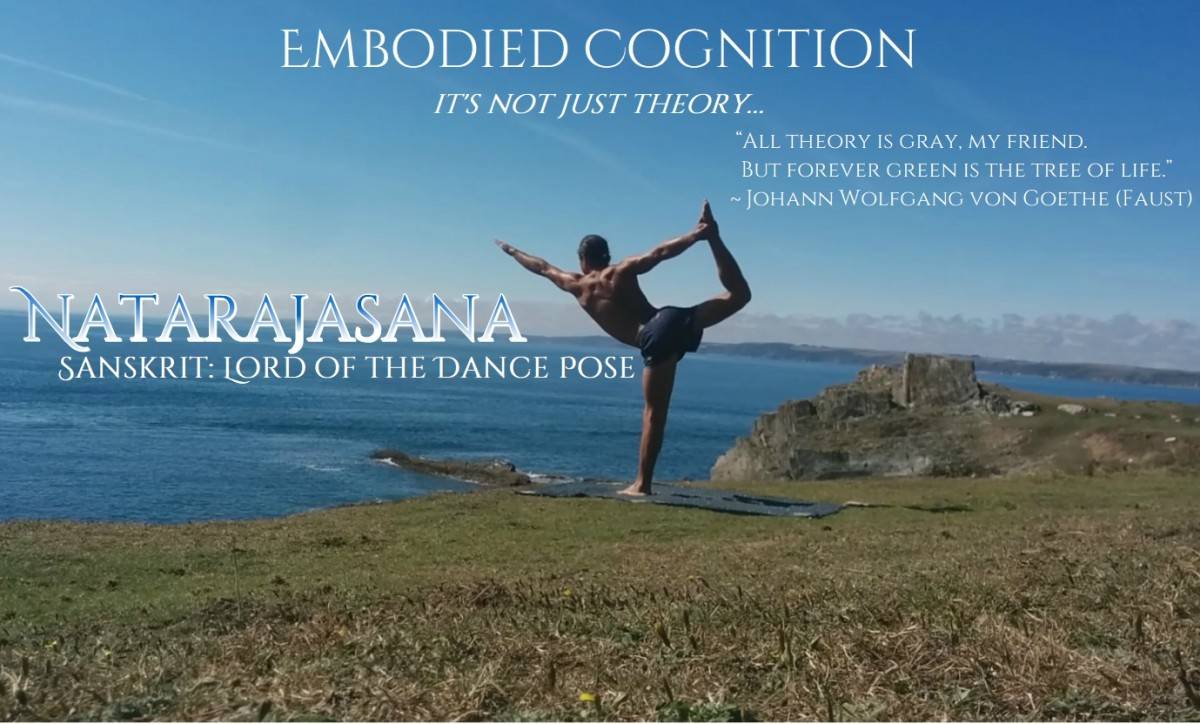
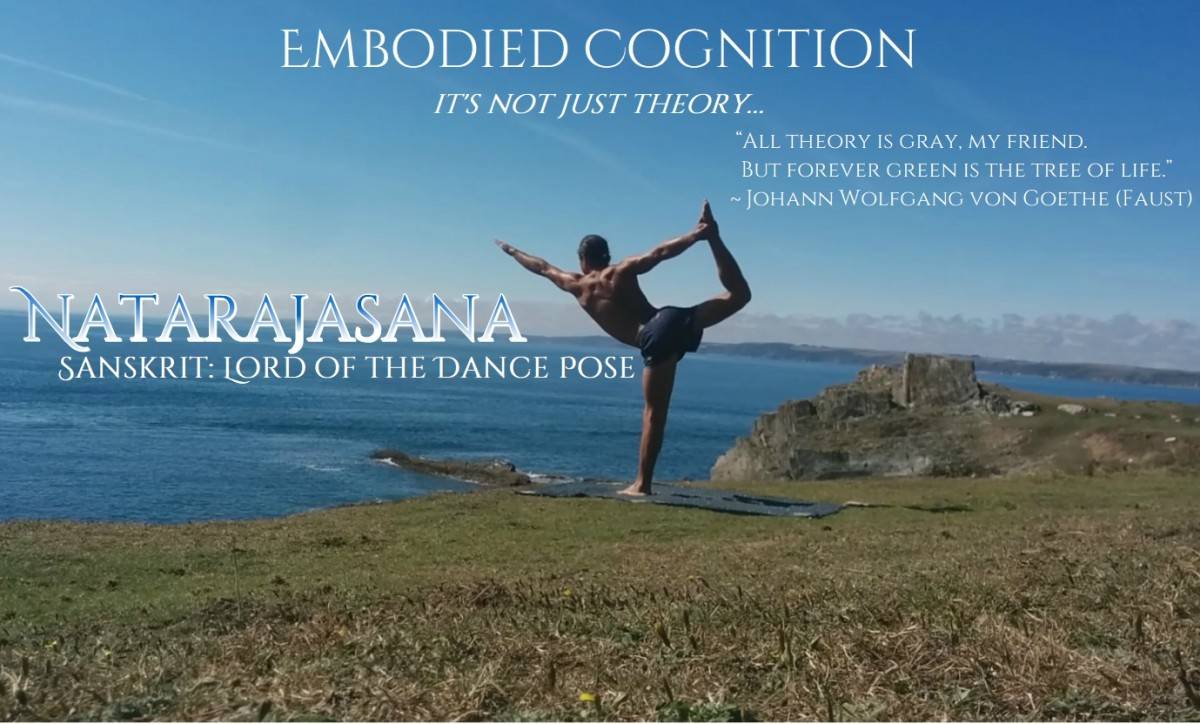
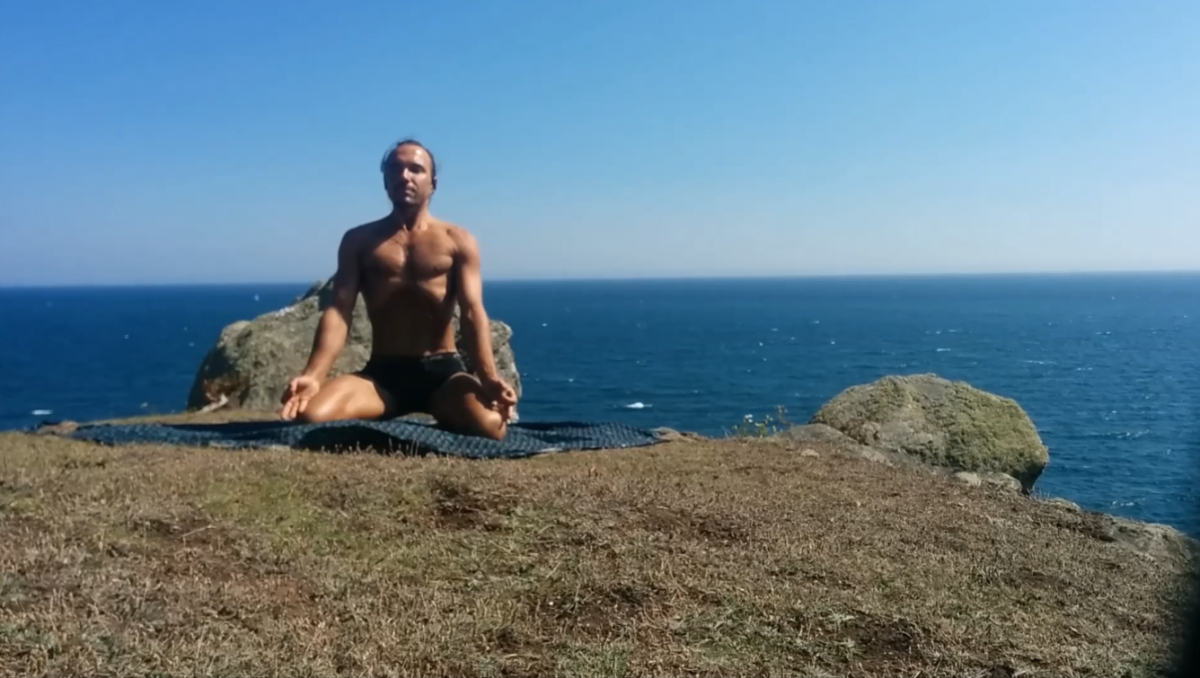
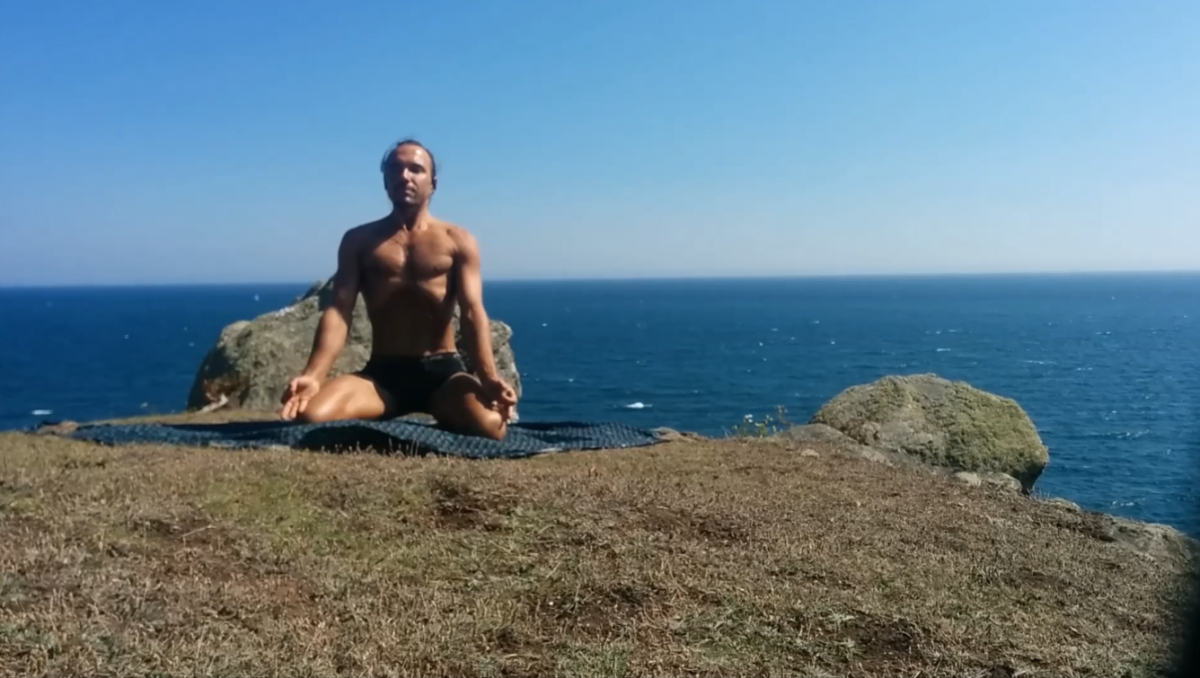
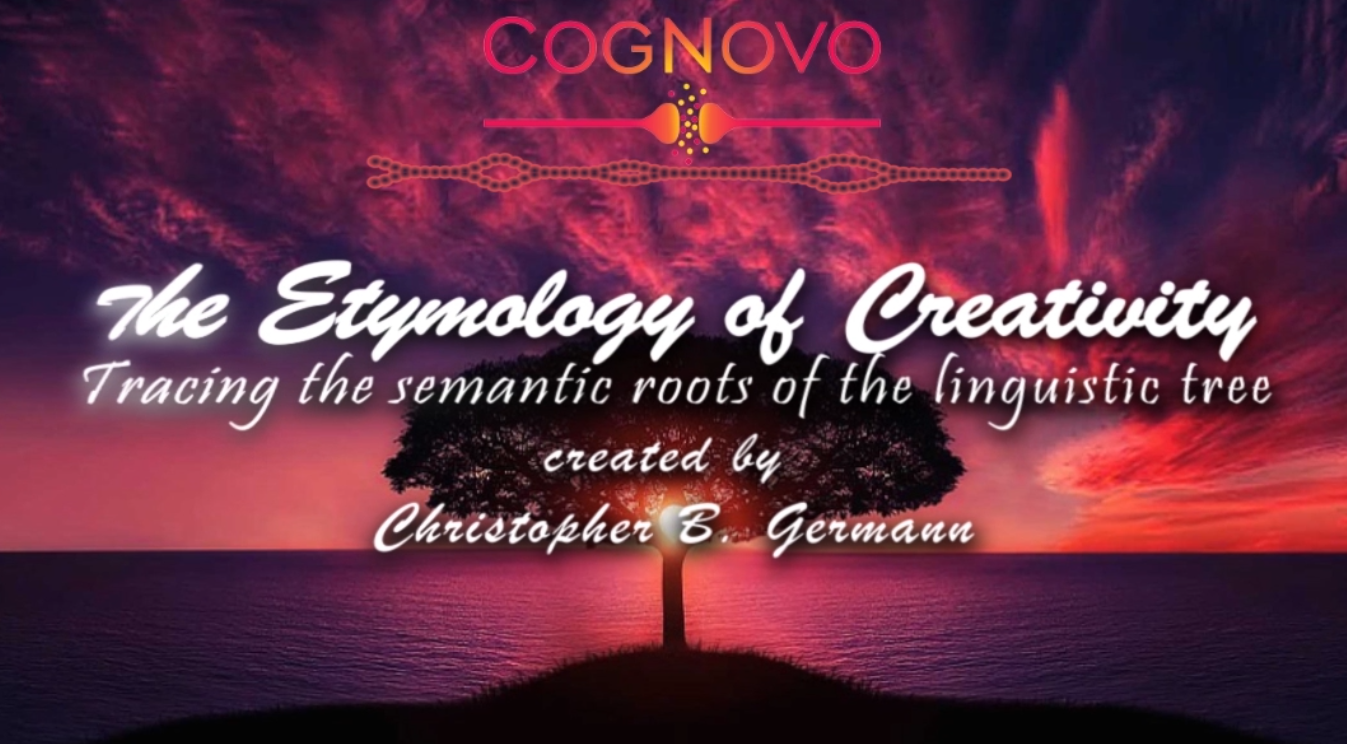
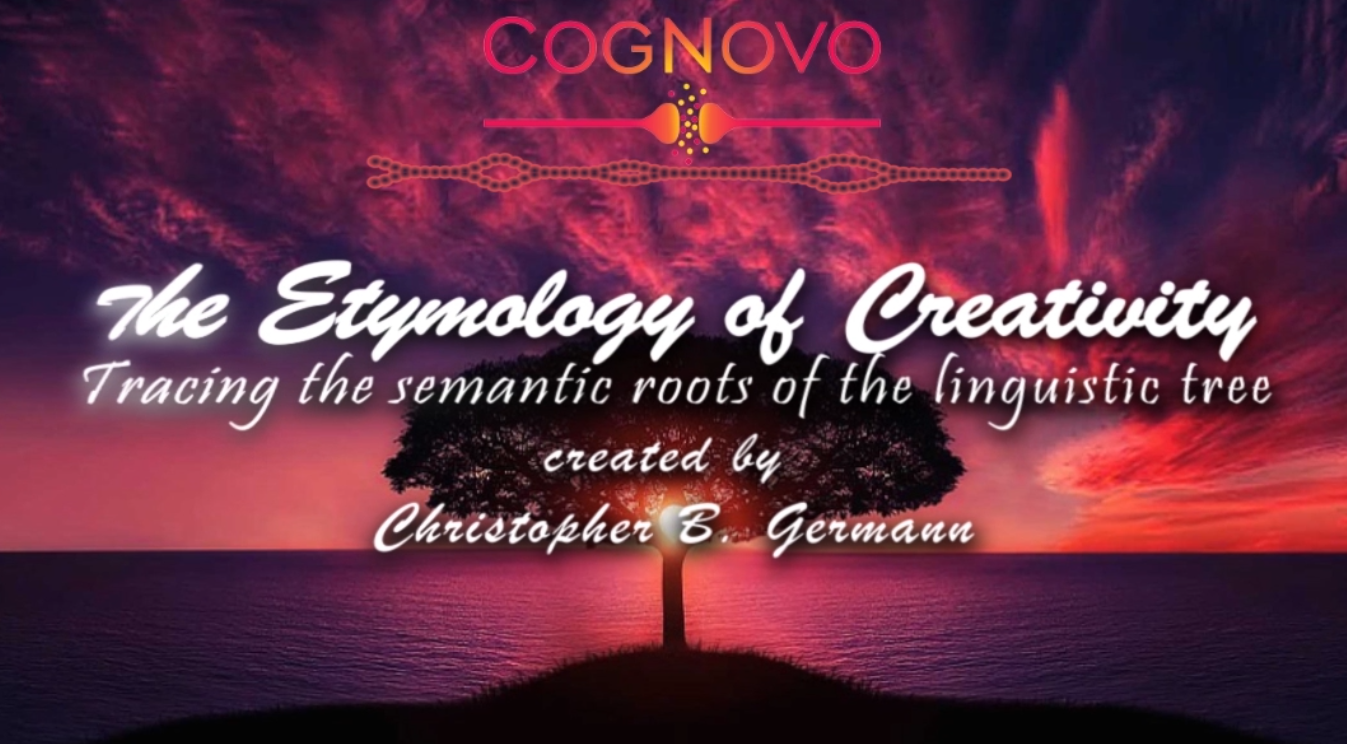
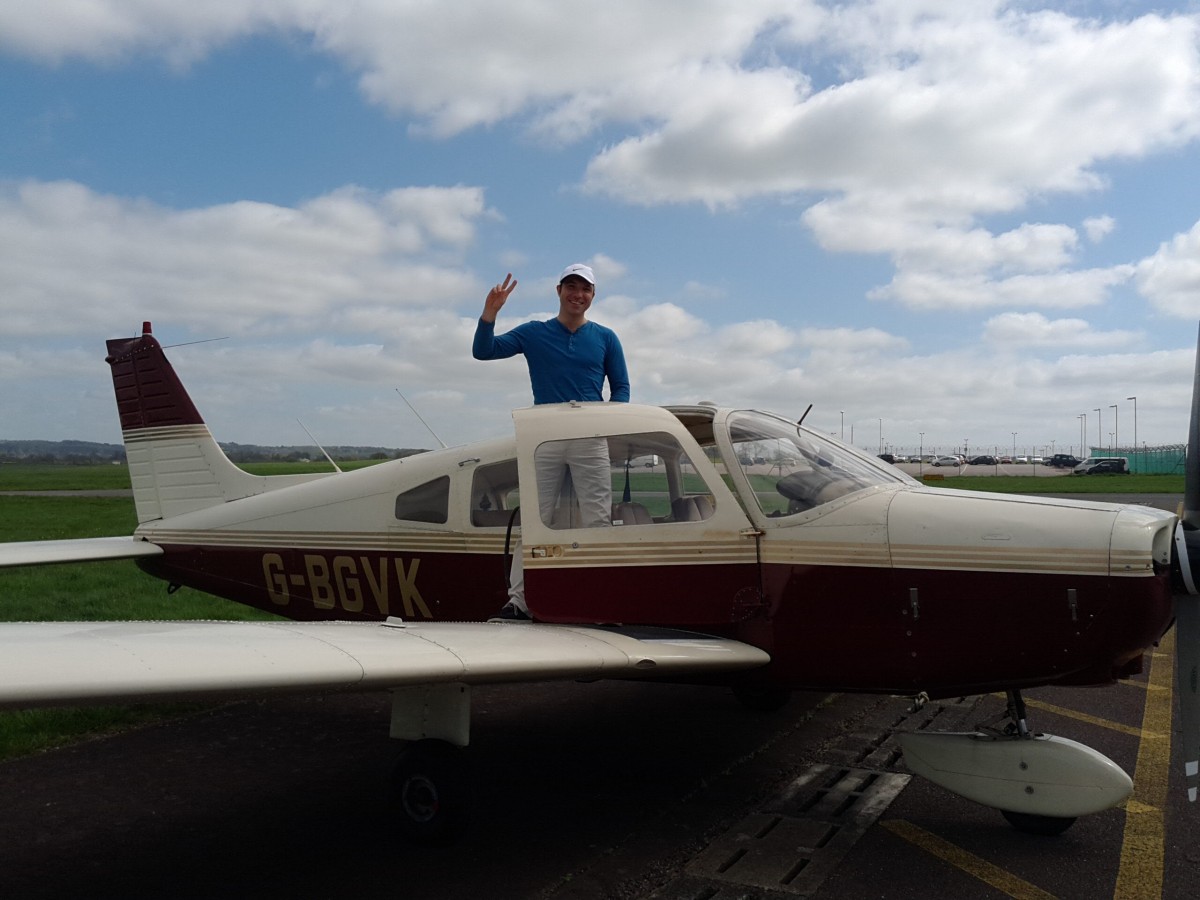
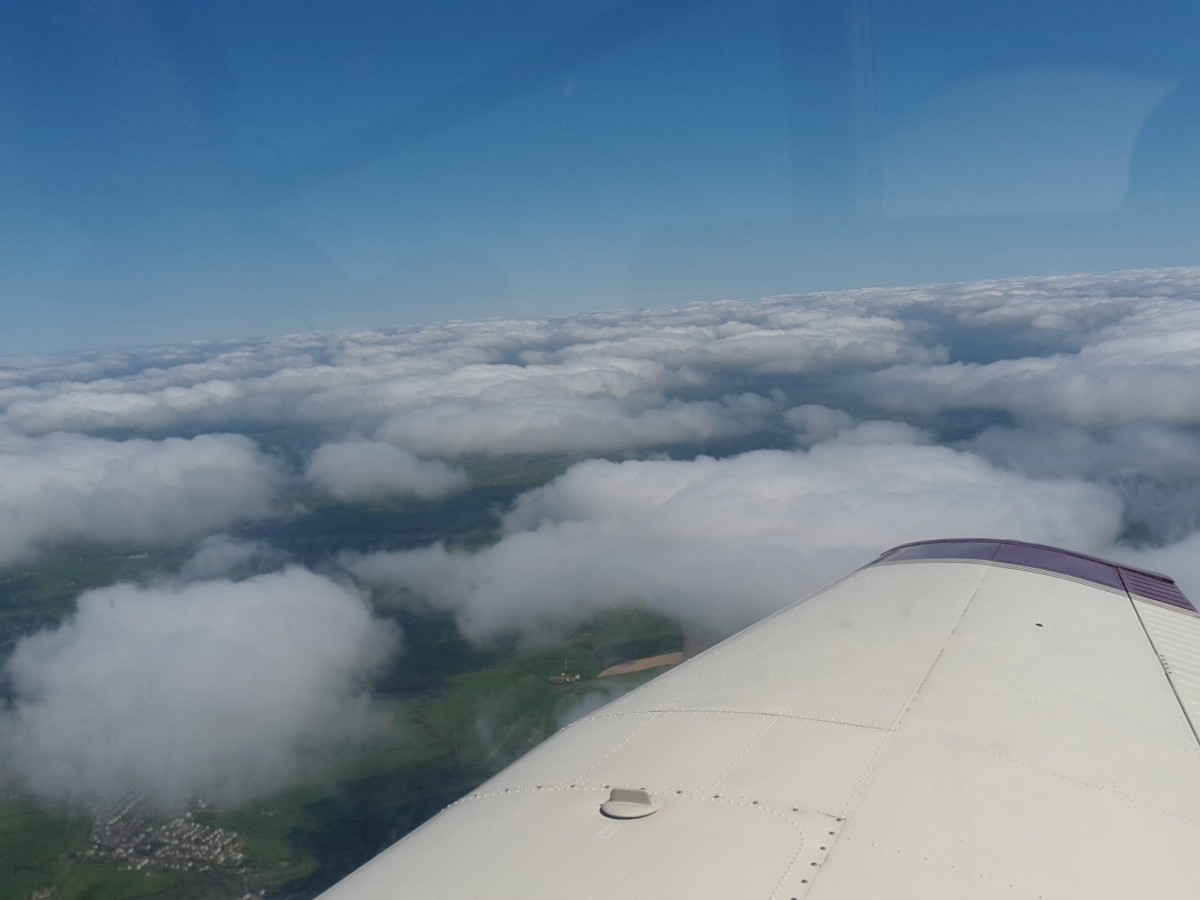
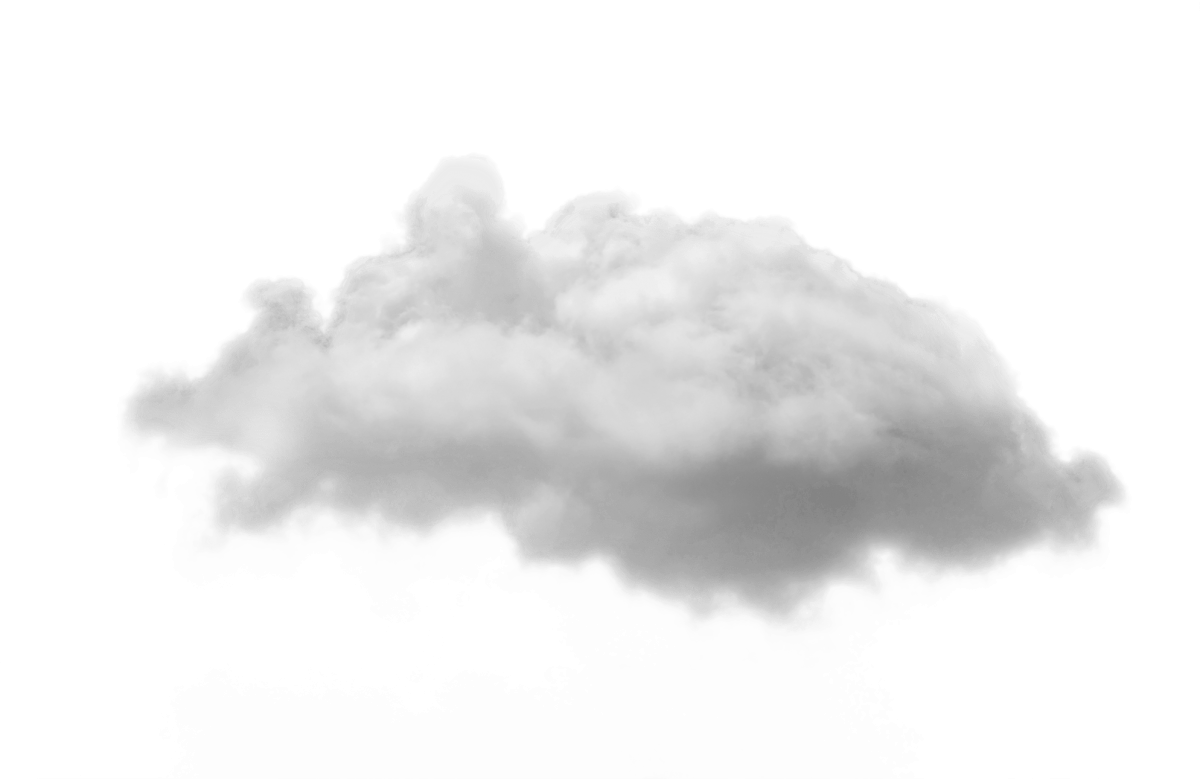

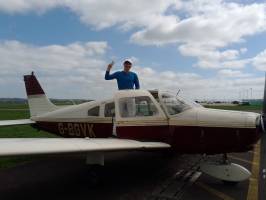
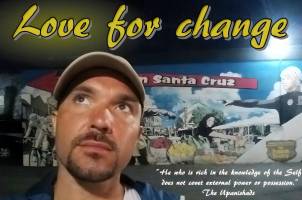
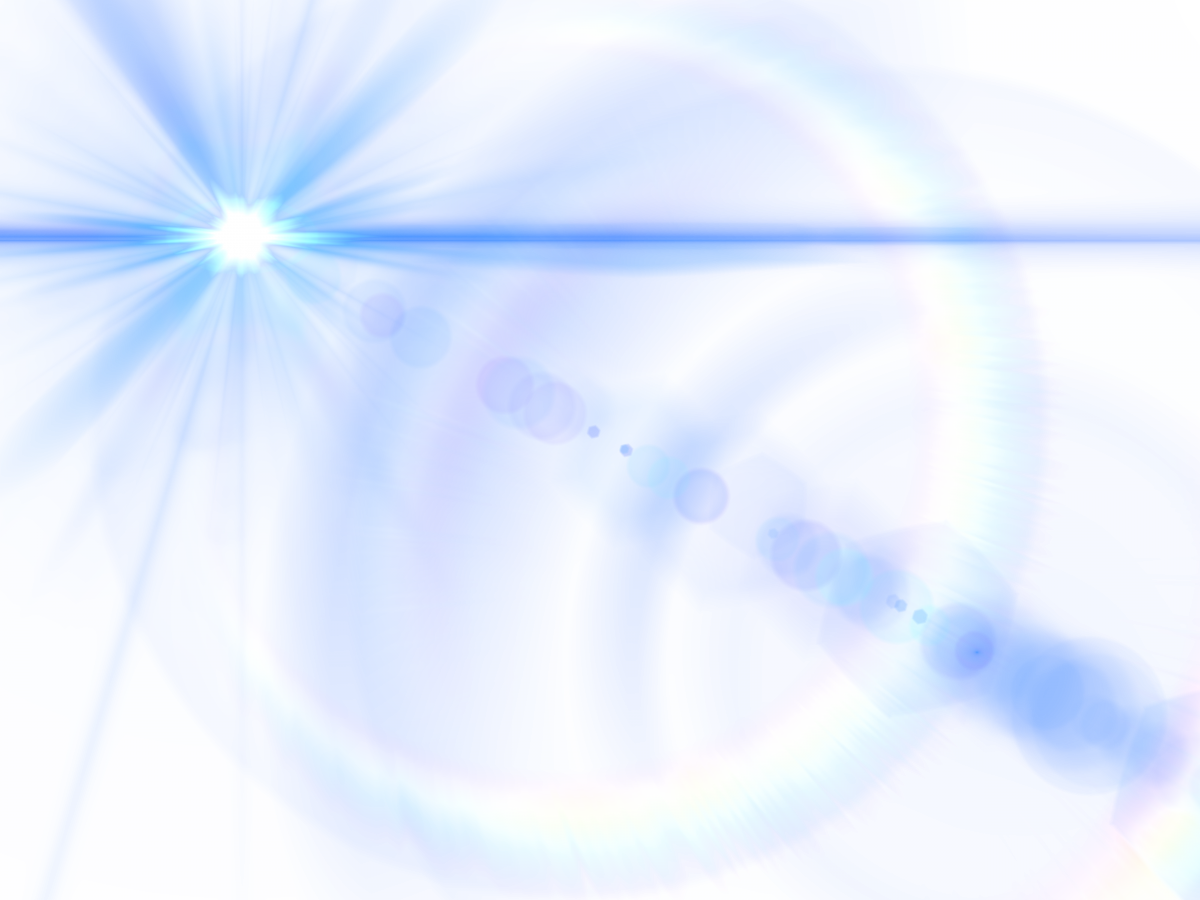
DeepDream is a psychophysical AI experiment that visualizes the patterns learned by a convoluted neural network. Similar to when a perceptually naive child watches clouds and tries to interpret random shapes, DeepDream over-interprets and enhances specific salient statistical patterns it detects in an image. It does so by forwarding an image through the Bayesian network, then calculating the gradient of the image with respect to the activations of a particular neighbouring layer. The image is then transformed to increase these activations, enhancing and perturbing the patterns seen by the network, and resulting in a dream-like image. This process was dubbed “Inceptionism” (a reference to InceptionNet, and the movie Inception). InceptionNet: https://arxiv.org/pdf/1409.4842.pdf
import tensorflow as tf import numpy as np import matplotlib as mpl import IPython.display as display import PIL.Image url = 'https://storage.googleapis.com/download.tensorflow.org/example_images/YellowLabradorLooking_new.jpg' # Download an image and read it into a NumPy array. def download(url, max_dim=None): name = url.split('/')[-1] image_path = tf.keras.utils.get_file(name, origin=url) img = PIL.Image.open(image_path) if max_dim: img.thumbnail((max_dim, max_dim)) return np.array(img) # Normalize an image def deprocess(img): img = 255*(img + 1.0)/2.0 return tf.cast(img, tf.uint8) # Display an image def show(img): display.display(PIL.Image.fromarray(np.array(img))) # Downsizing the image makes it easier to work with. original_img = download(url, max_dim=500) show(original_img) display.display(display.HTML('Image cc-by: Von.grzanka')) base_model = tf.keras.applications.InceptionV3(include_top=False, weights='imagenet') # Maximize the activations of these layers names = ['mixed3', 'mixed5'] layers = [base_model.get_layer(name).output for name in names] # Create the feature extraction model dream_model = tf.keras.Model(inputs=base_model.input, outputs=layers) def calc_loss(img, model): # Pass forward the image through the model to retrieve the activations. # Converts the image into a batch of size 1. img_batch = tf.expand_dims(img, axis=0) layer_activations = model(img_batch) if len(layer_activations) == 1: layer_activations = [layer_activations] losses = [] for act in layer_activations: loss = tf.math.reduce_mean(act) losses.append(loss) return tf.reduce_sum(losses)This tutorial contains a minimal implementation of DeepDream, as described by Alexander Mordvintsev.
Namarupa
Panta Rhei
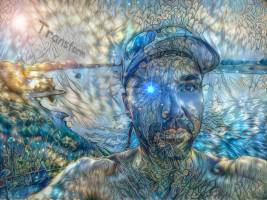
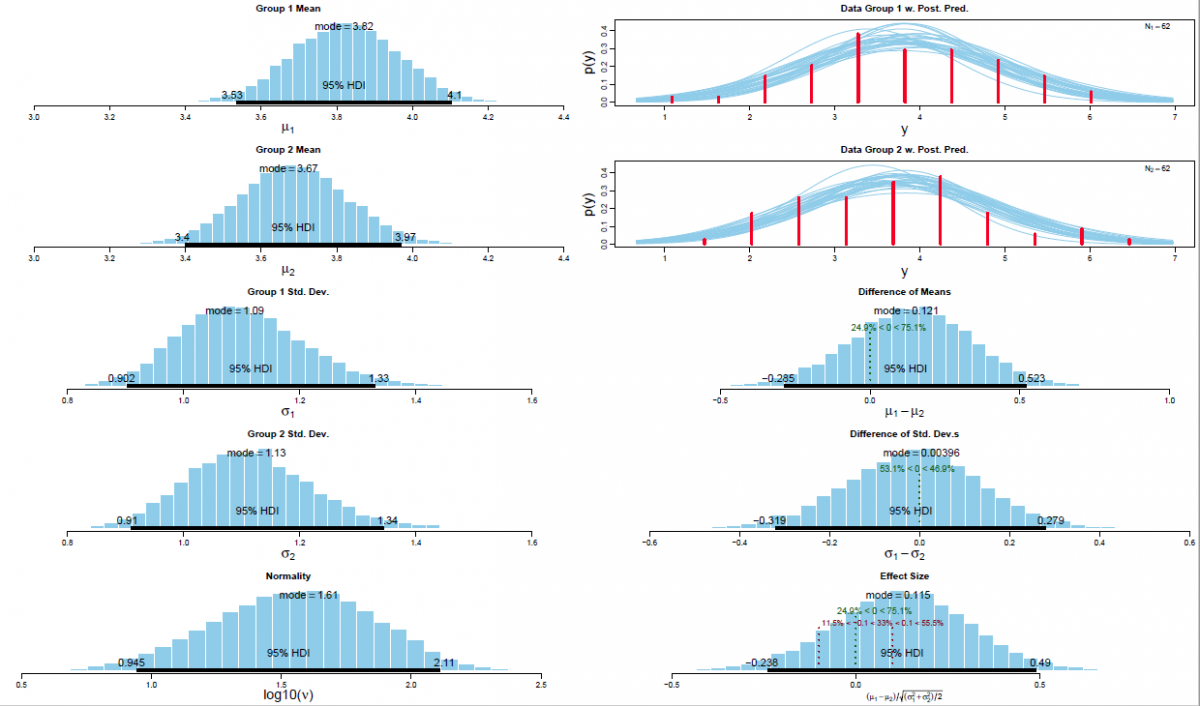
statistical null hypothesis significance testing (NHST) based on the fallacious/invalid logic associated with p-values and fixed α-levels.
via Markov chain Monte Carlo simulations
Mindless statistical rituals in science
A critique of Fisherian p-values
"Few researchers are aware that their own heroes rejected what they practice routinely. Awareness of the origins of the ritual and of its rejection could cause a virulent cognitive dissonance, in addition to dissonance with editors, reviewers, and dear colleagues. Suppression of conflicts and contradicting information is in the very nature of this social ritual.”
(Gigerenzer, 2004, p. 592)
***
Gigerenzer, G. (2004). Mindless statistics. The Journal of Socio-Economics, 33(5), 587–606. https://doi.org/10.1016/j.socec.2004.09.033
#clears all of R's memory rm(list=ls()) # Get the functions loaded into R's working memory # The function can also be downloaded from the following URL: # http://irrational-decisions.com/?page_id=1996 source("BEST.R") #download data from server dataexp2 <- read.table("https://www.irrational-decisions.com/phd-thesis/dataexp2.txt", header=TRUE, sep="", na.strings="NA", dec=".", strip.white=TRUE) # Specify data as vectors y1 = c(dataexp2$v00) y2 = c(dataexp2$v01) # Run the Bayesian analysis using the default broad priors described by Kruschke (2013) mcmcChain = BESTmcmc( y1 , y2 , priorOnly=FALSE , numSavedSteps=12000 , thinSteps=1 , showMCMC=TRUE ) postInfo = BESTplot( y1 , y2 , mcmcChain , ROPEeff=c(-0.1,0.1) ) #The function “BEST.R” can be downloaded from the CRAN (Comprehensive R Archive Network) repository under https://cran.r-project.org/web/packages/BEST/index.html
Plain numerical DOI: 10.3758/s13423-016-1221-4
DOI URL
directSciHub download
Show/hide publication abstract
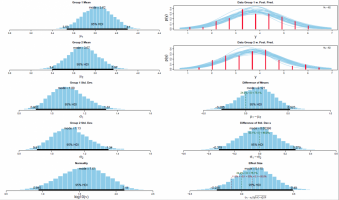
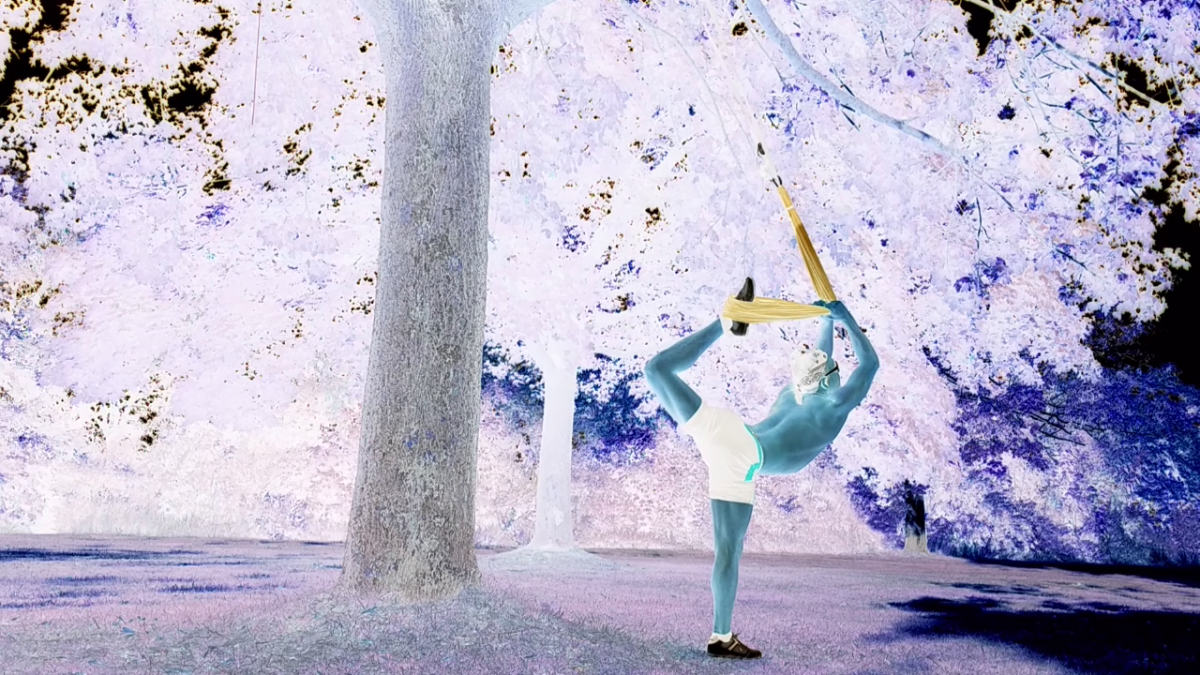
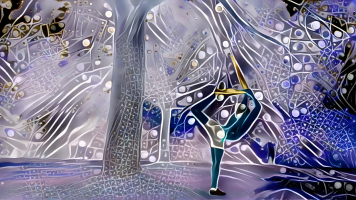
The Amazon deforestation rate in 2020 is the greatest of the decade.
I suggest that humanity should focus on saving the rainforest and not on fuzzy, propagandistic and theory-laden concepts such as "global warming". The rainforest is a clear quantitative indicator of "planetary health". It is thus a statistical criterion which can be measured with great accuracy. Complex systems thinking is needed. Interconnectivity is a key principle of life. The rainforest demonstrates this principle, i.e., the rainforest is an intelligent super-organism.
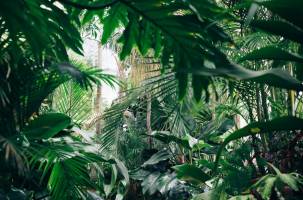
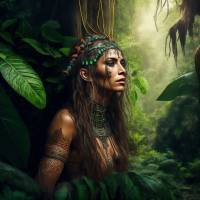
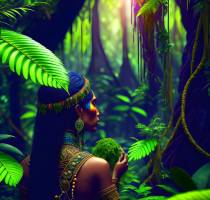
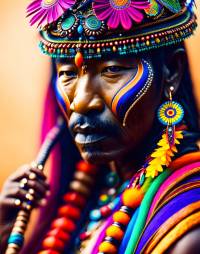
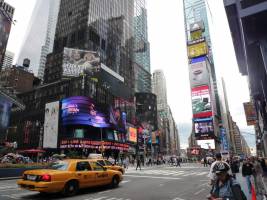
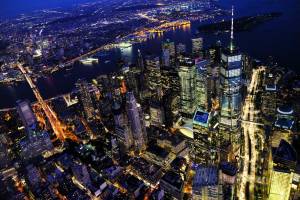
*in memoriam of the satanic Deep State
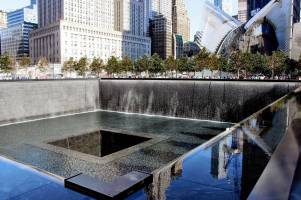
~Roscoe C. Brown
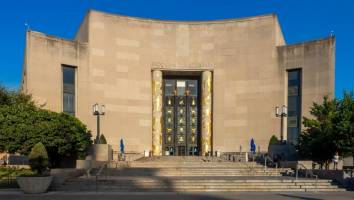
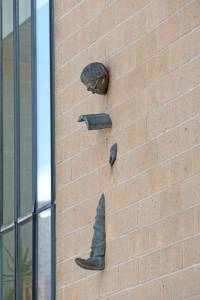
Dolce Hayes Mansion
in Californias Silicon Valley
2016
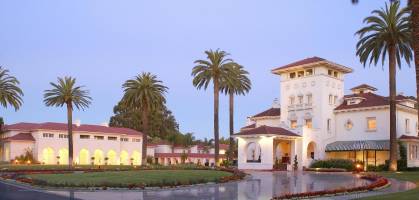
Plain numerical DOI: 10.1177/23978473221085746
DOI URL
directSciHub download
Show/hide publication abstract
Plain numerical DOI: 10.1038/s41598-020-78527-4
DOI URL
directSciHub download
Show/hide publication abstract
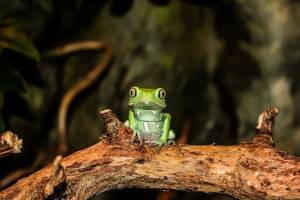
Plain numerical DOI: 10.1177/23978473221085746
DOI URL
directSciHub download
Show/hide publication abstract
Plain numerical DOI: 10.1038/s41598-020-78527-4
DOI URL
directSciHub download
Show/hide publication abstract
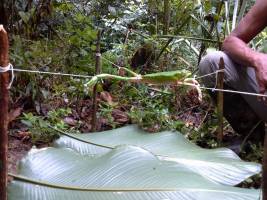
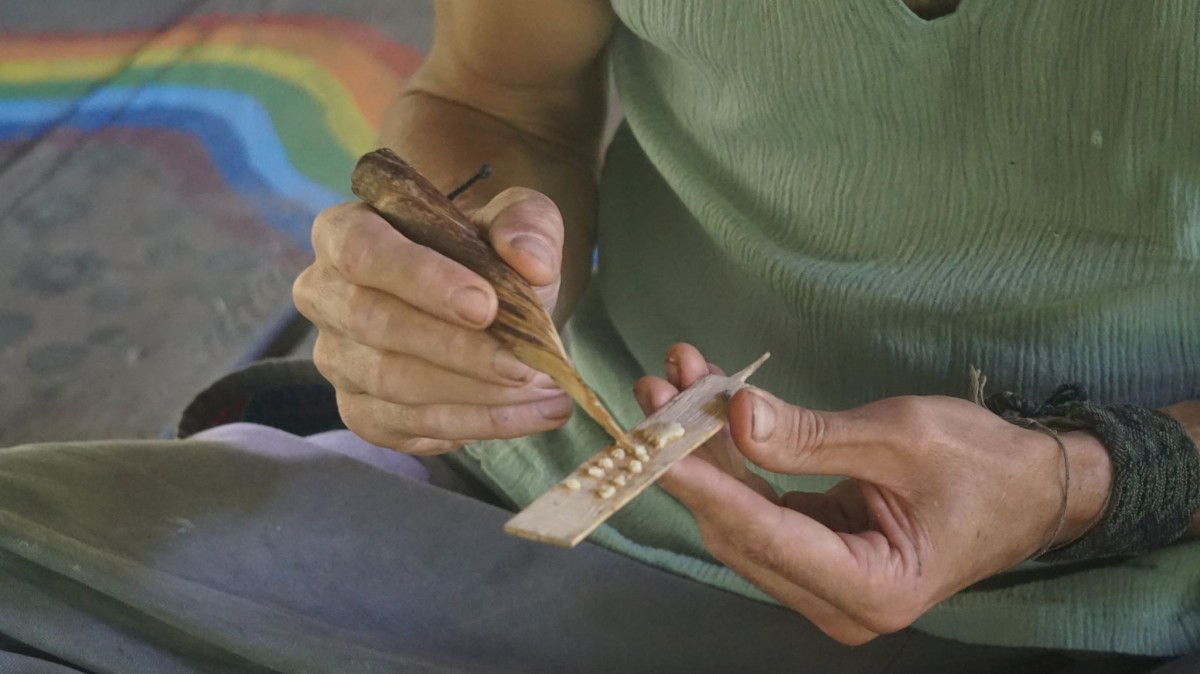
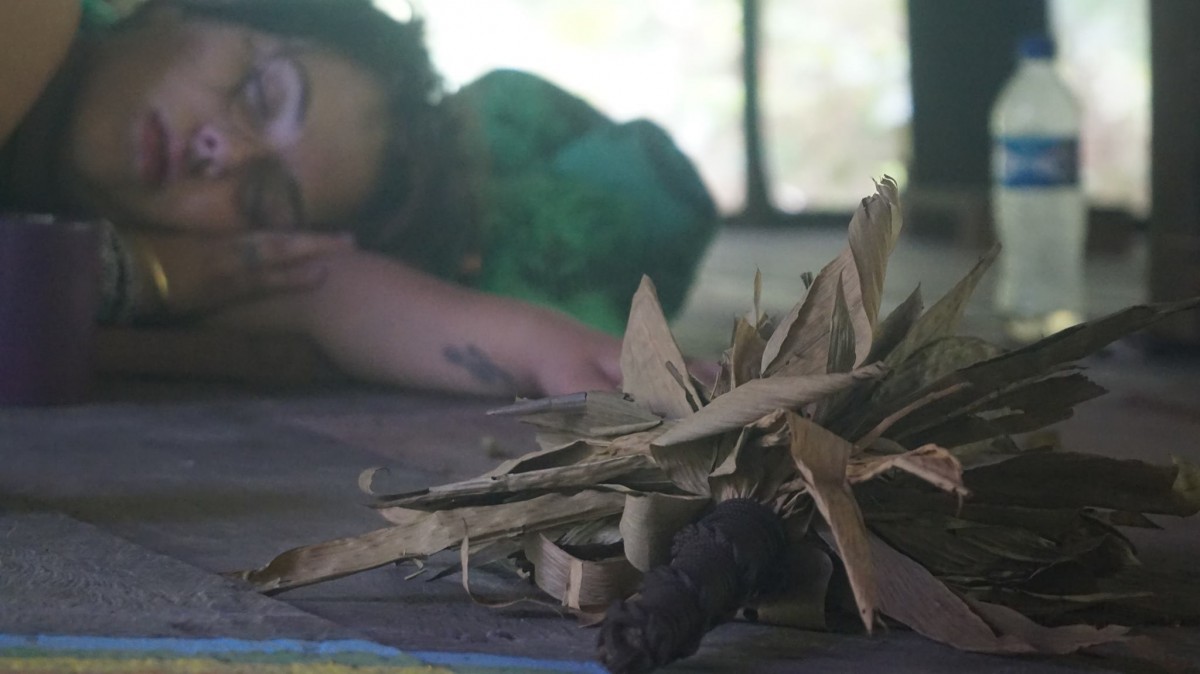
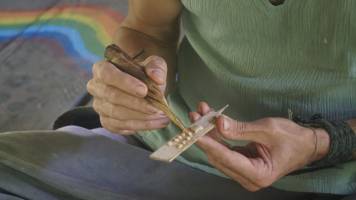
Iquitos
Peru
2022
♫ Marinera
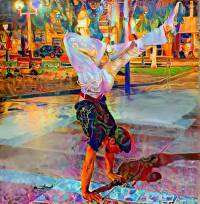
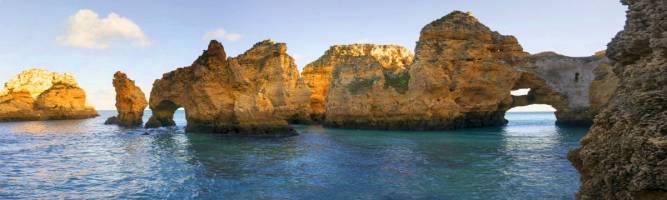
Switzerland, Filzbach 2015

Peruvian Amazon
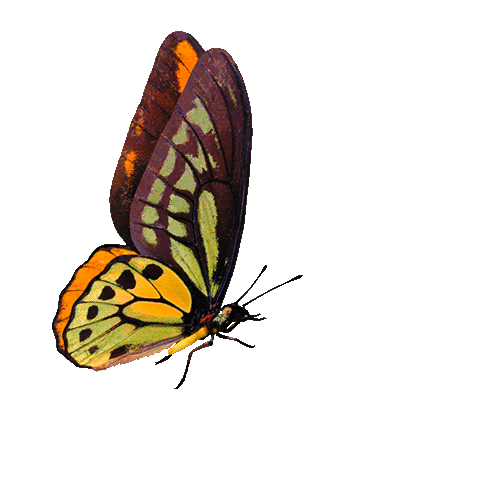
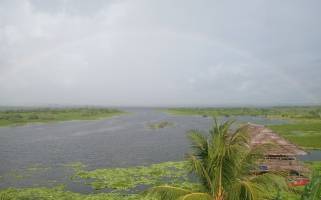
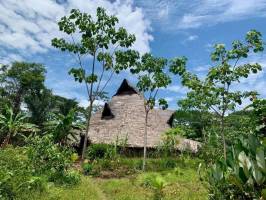
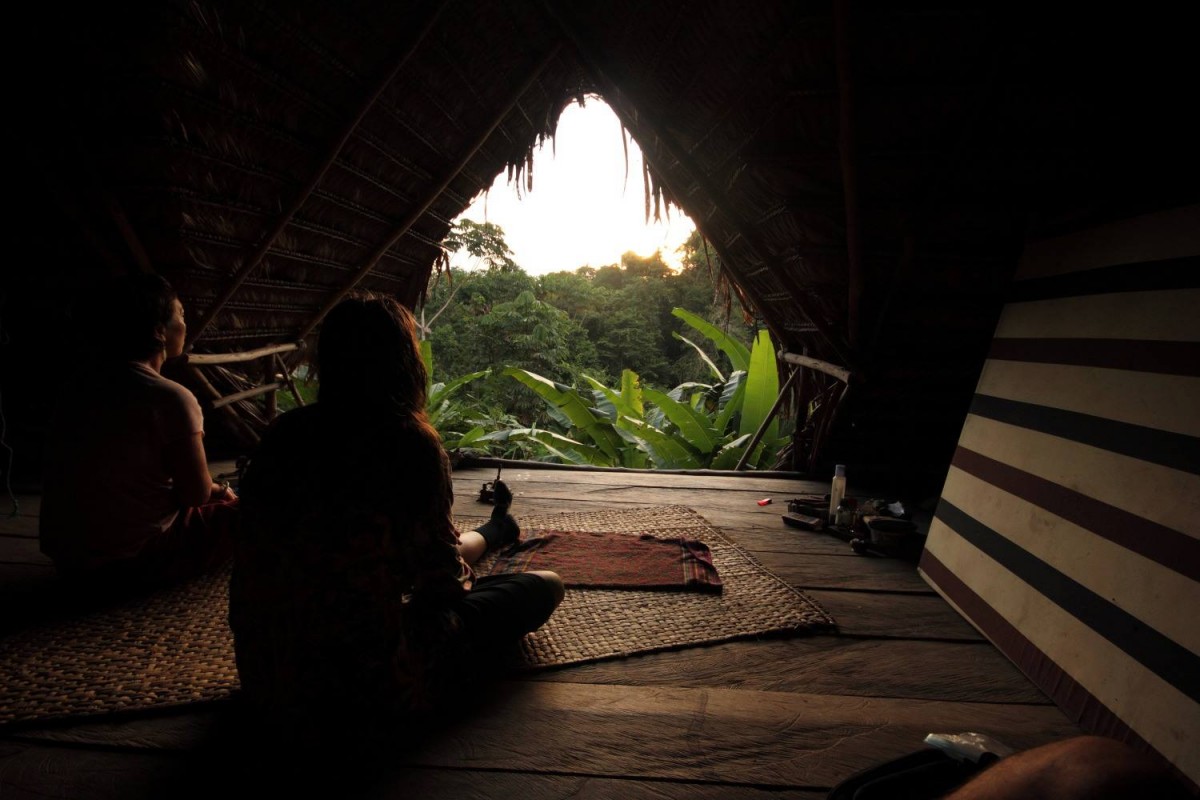
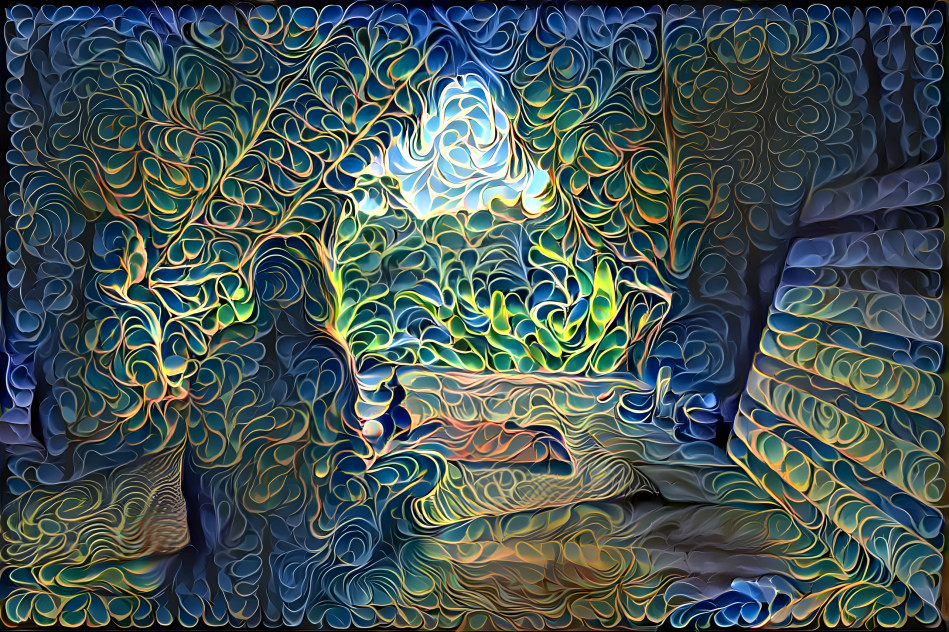
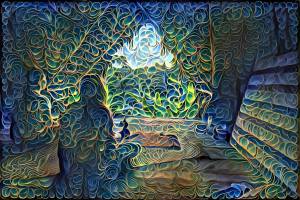
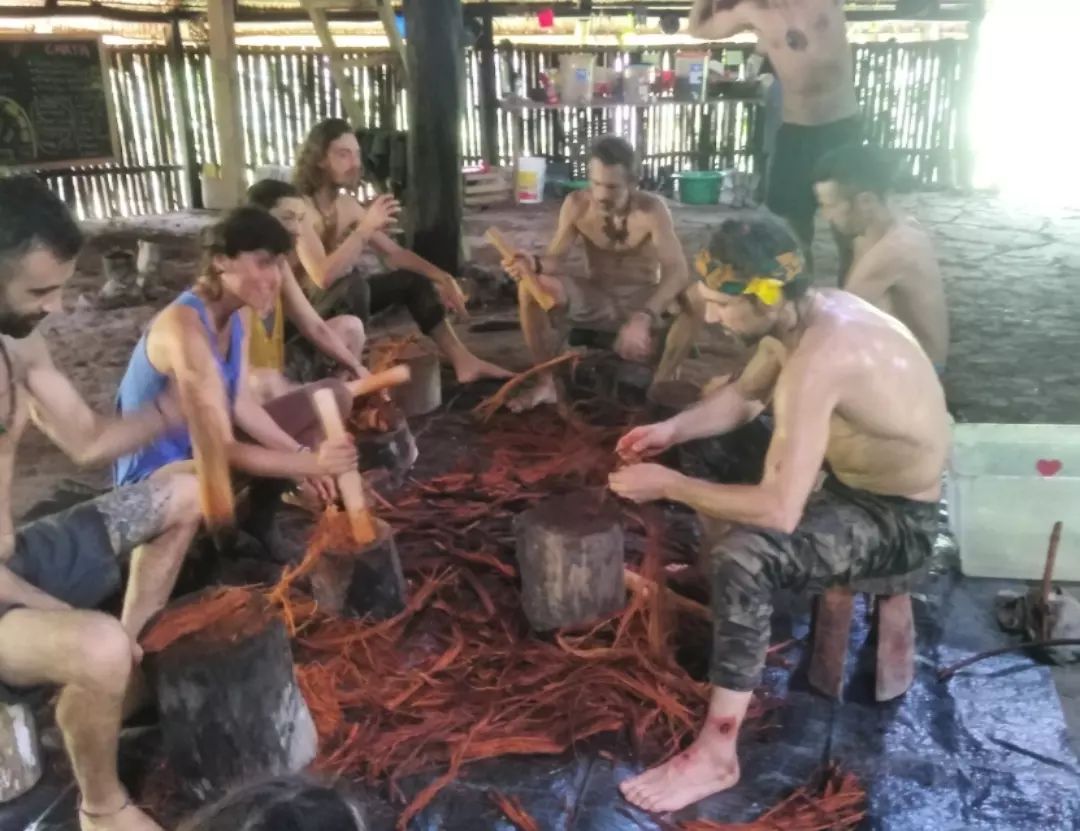
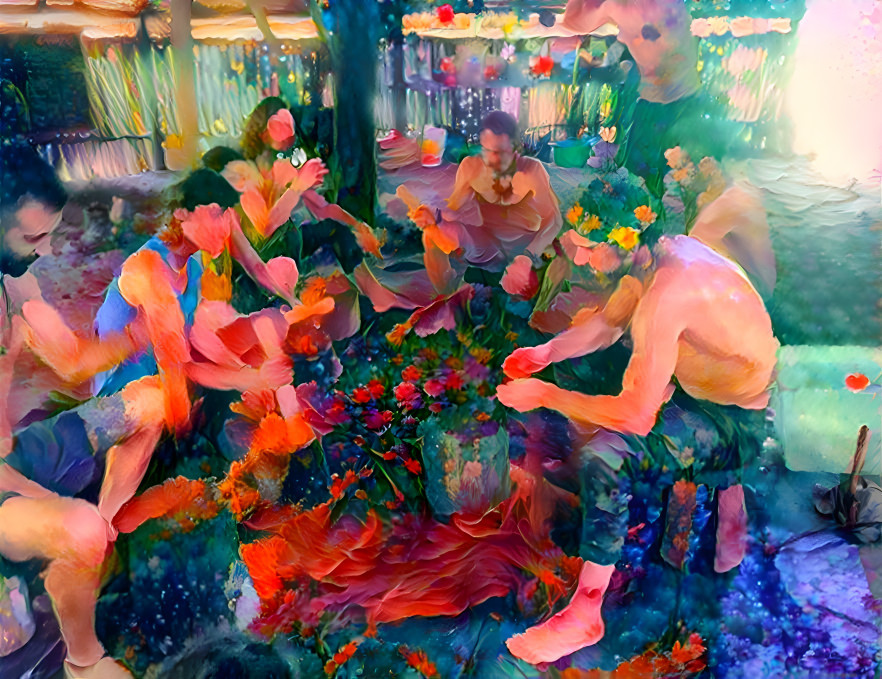
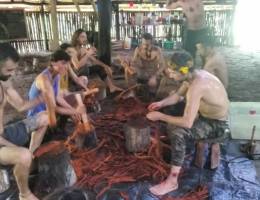
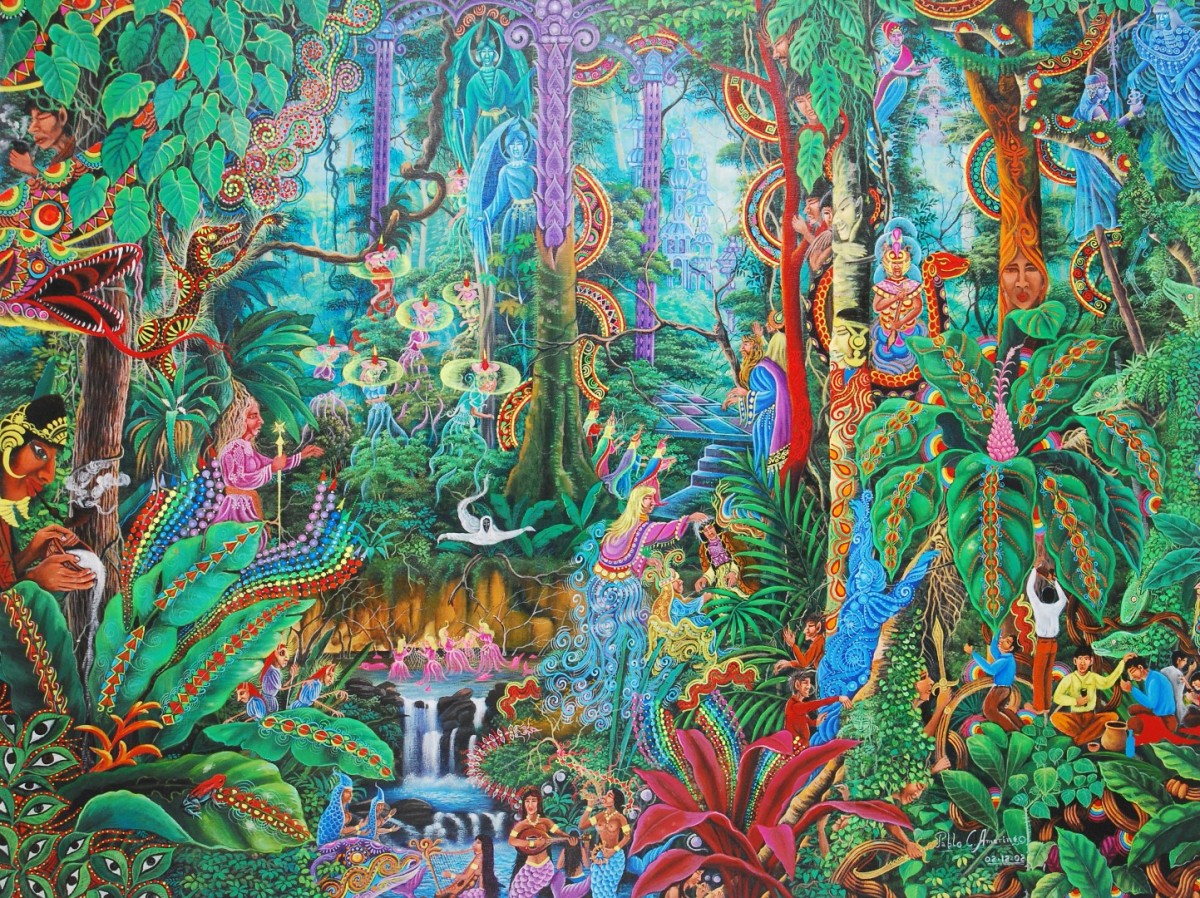

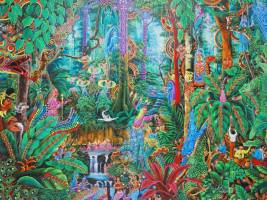
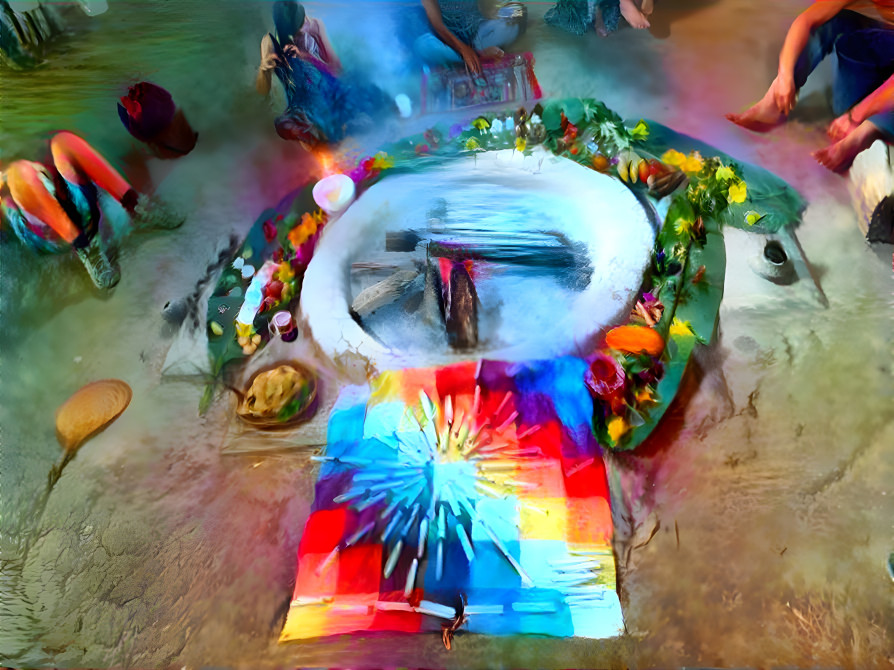
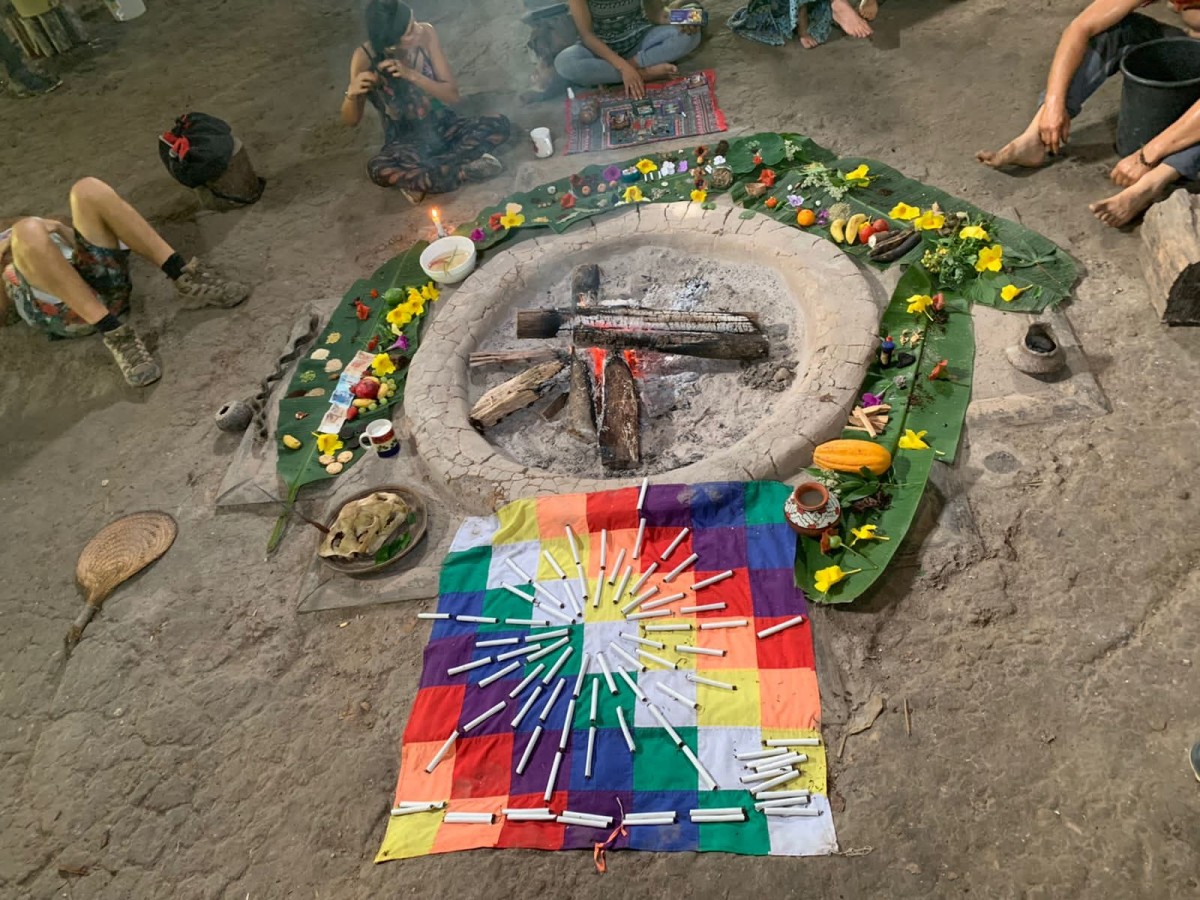
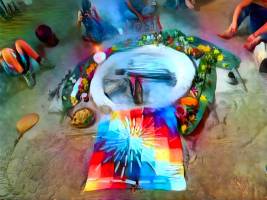
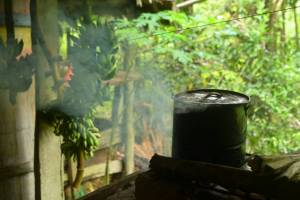
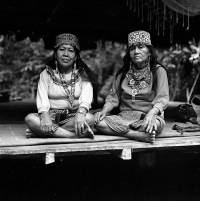
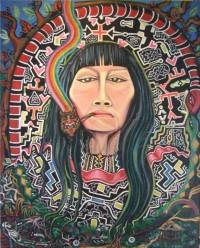
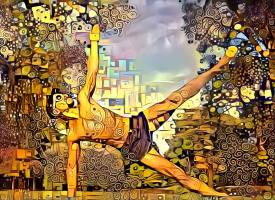
aka. Aguaje
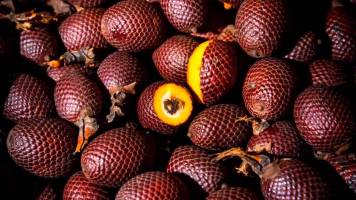
aka. Uña de Gato
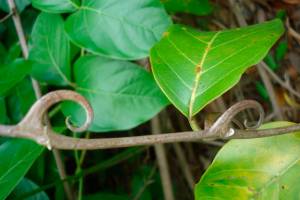
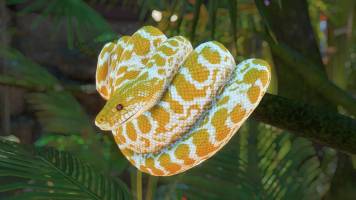
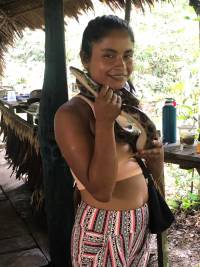
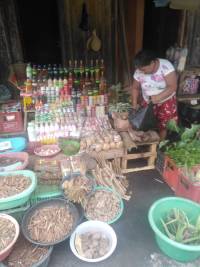
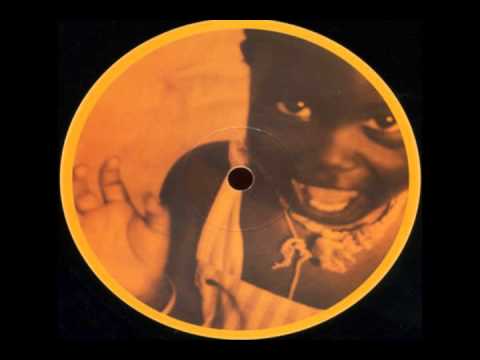
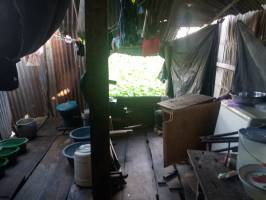
India 2016
In anthropology (and the social and behavioral sciences) the terms emic and etic refer to two different types of field research. Emic is a perspective from within the social group (from the perspective of the subject). Per contrast, etic refers to an outside-perspective (from the perspective of the observer). In my opinion both are complementary to each other (in the quantum-physical sense of complementarity; cf. bistable perception).
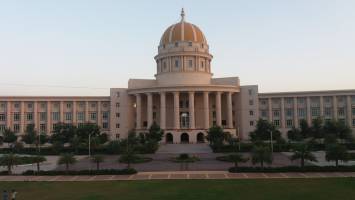
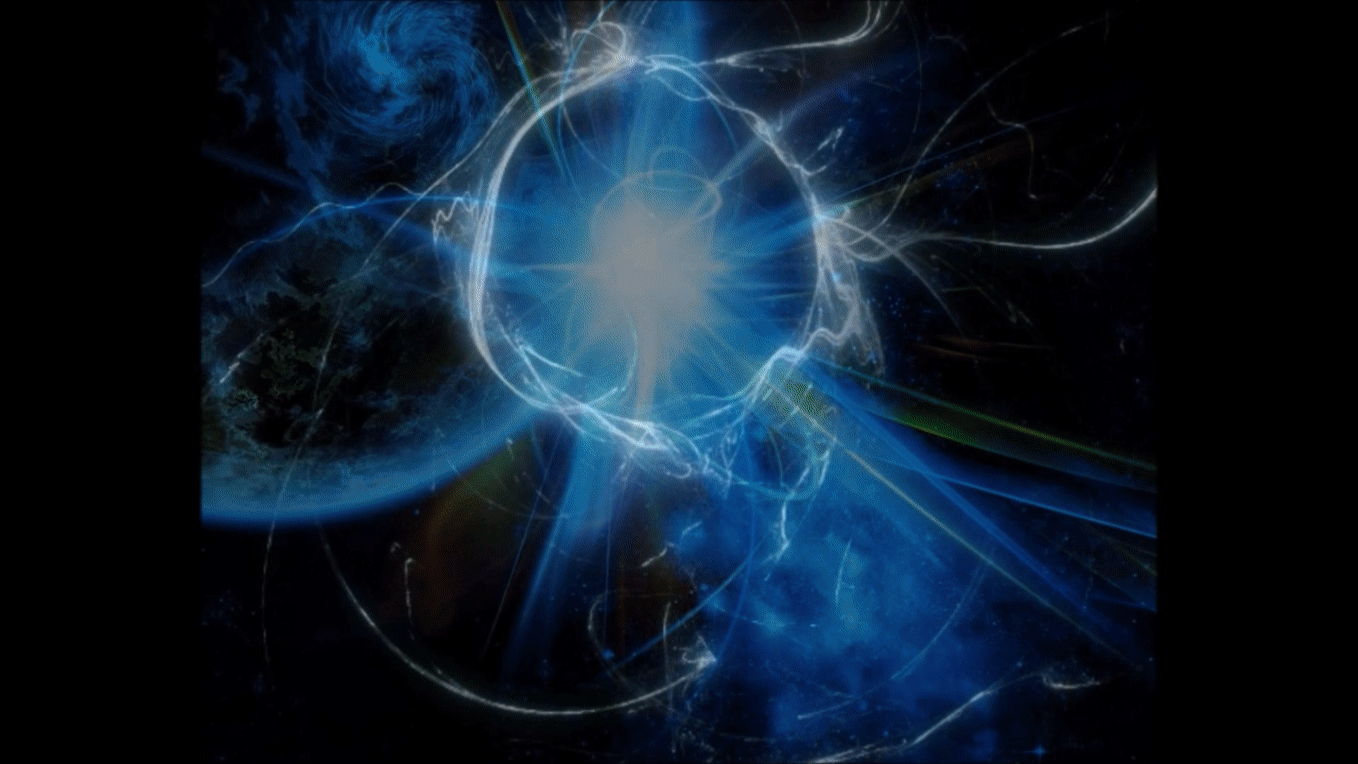
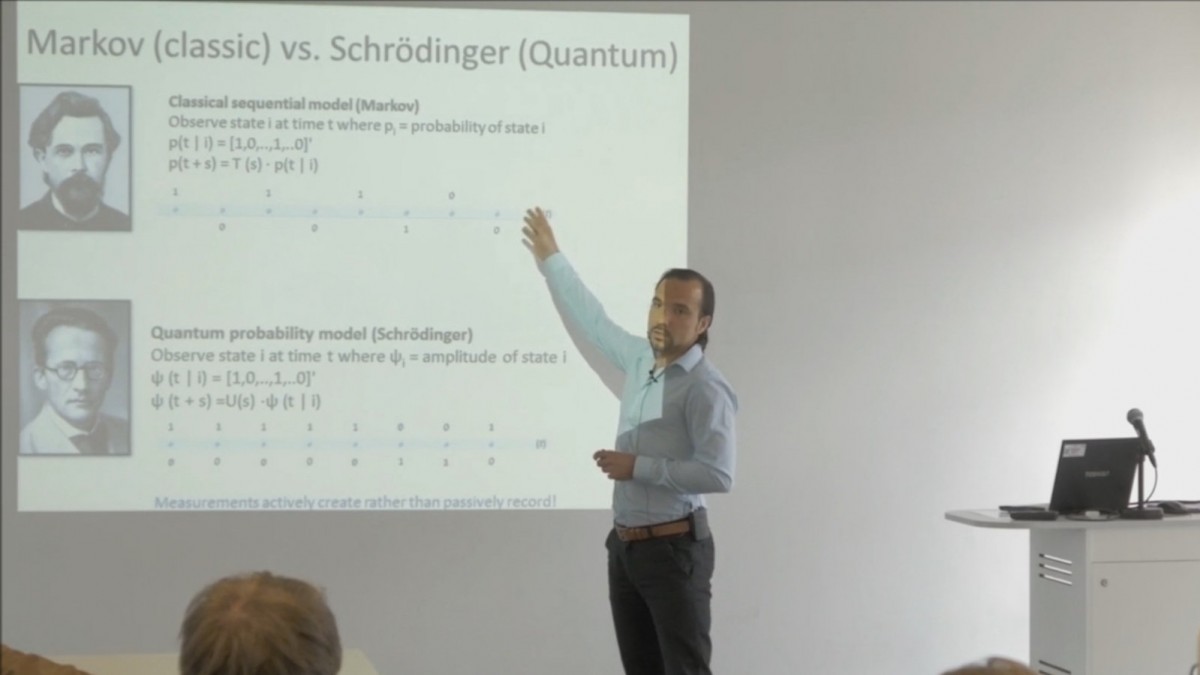
This presentation focuses on the role of noncommutativity in visual/perceptual decision-making.
First, some historical background information is provided.
Then, the interrelated concepts of complementarity and superposition are briefly delineated and two paradigmatic visual illusions are demonstrated.
Next, several pertinent empirical results are discussed in the theoretical framework of quantum cognition.
Finally, the interdisciplinary scope of the topic will be adumbrated in the context of "cognitive innovation" (CogNovo).
Switch to fullscreen-mode
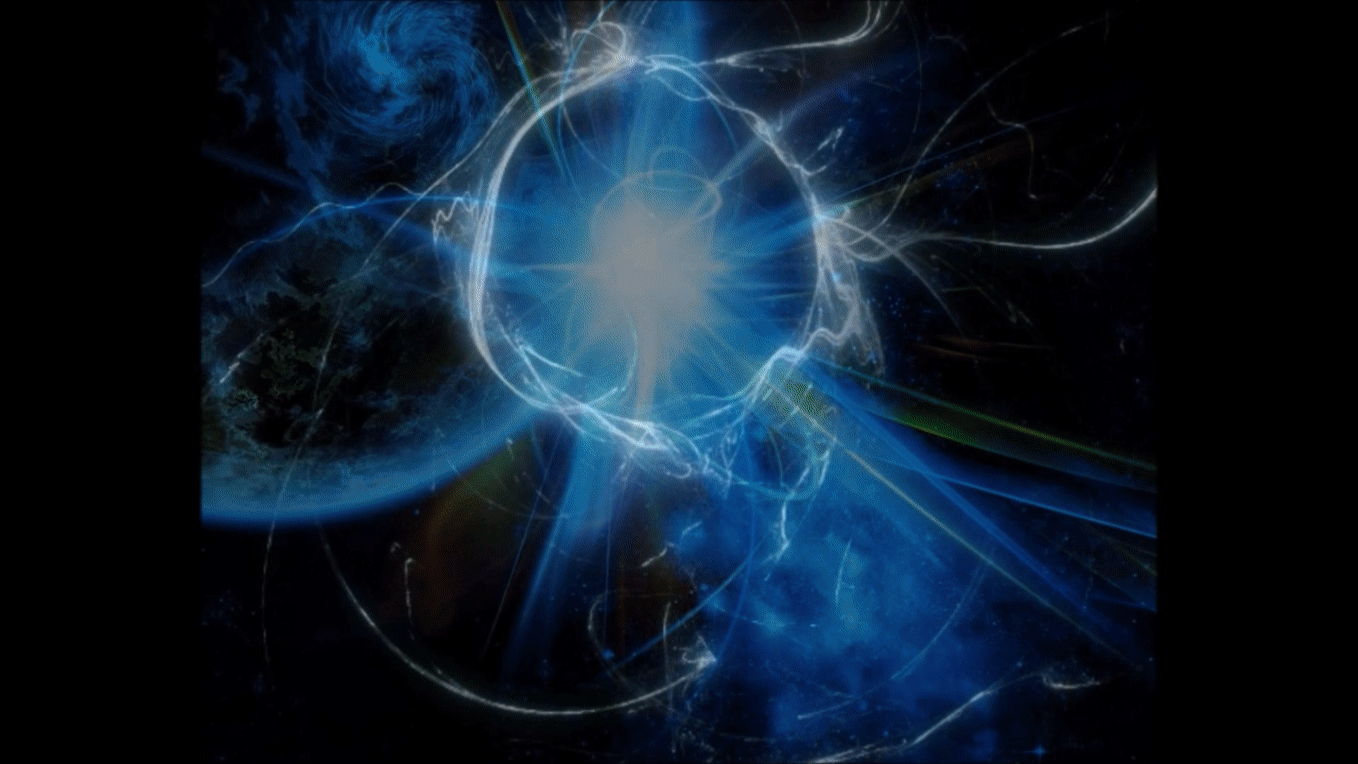
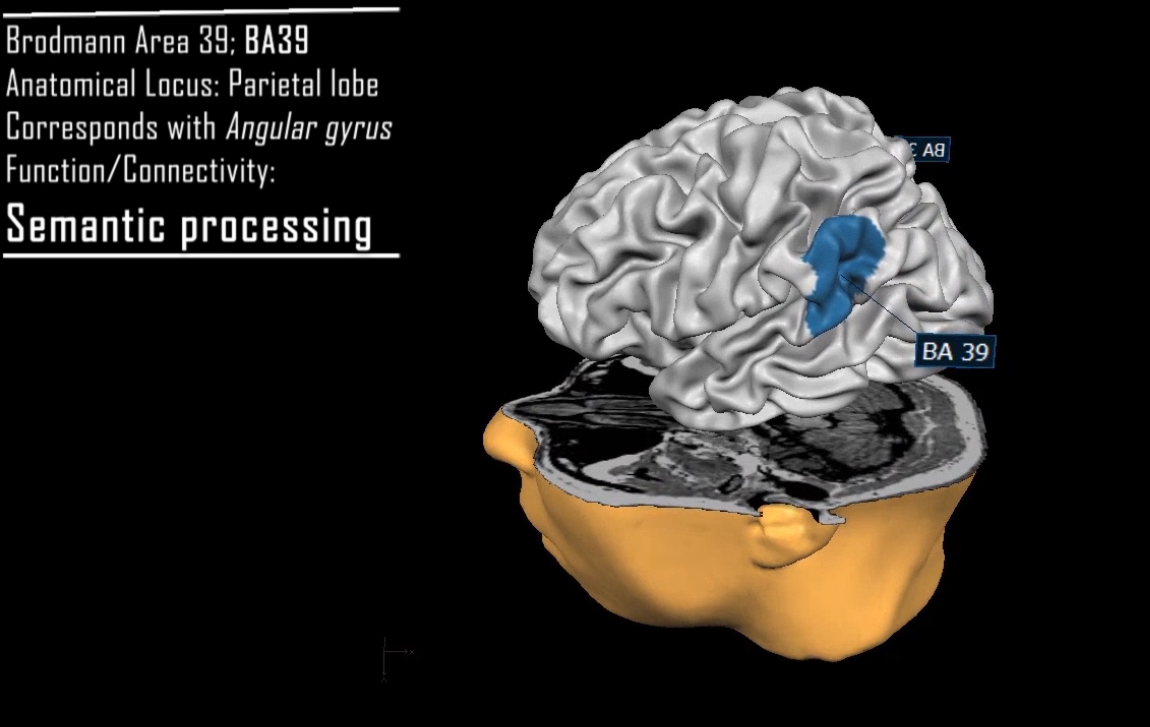
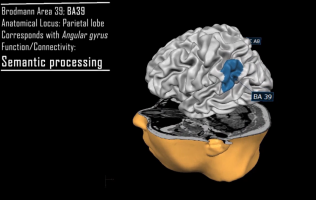
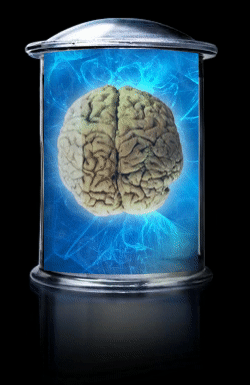
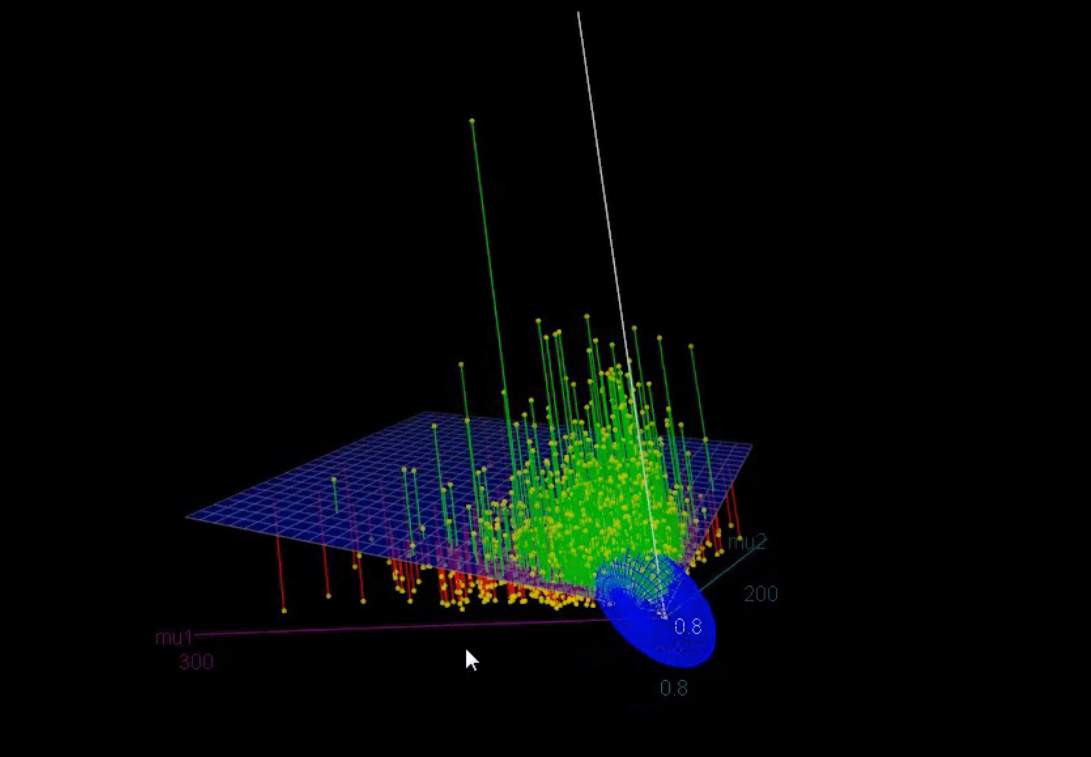
scatterplot3d(x, y=NULL, z=NULL, color=par("col"), pch=par("pch"), main=NULL, sub=NULL, xlim=NULL, ylim=NULL, zlim=NULL, xlab=NULL, ylab=NULL, zlab=NULL, scale.y=1, angle=40, axis=TRUE, tick.marks=TRUE, label.tick.marks=TRUE, x.ticklabs=NULL, y.ticklabs=NULL, z.ticklabs=NULL, y.margin.add=0, grid=TRUE, box=TRUE, lab=par("lab"), lab.z=mean(lab[1:2]), type="p", highlight.3d=FALSE, mar=c(5,3,4,3)+0.1, bg=par("bg"), col.axis=par("col.axis"), col.grid="grey", col.lab=par("col.lab"), cex.symbols=par("cex"), cex.axis=0.8 * par("cex.axis"), cex.lab=par("cex.lab"), font.axis=par("font.axis"), font.lab=par("font.lab"), lty.axis=par("lty"), lty.grid=par("lty"), lty.hide=NULL, lty.hplot=par("lty"), log="", asp=NA, ...)
Plain numerical DOI: 10.1214/12-STS402
DOI URL
directSciHub download
Show/hide publication abstract
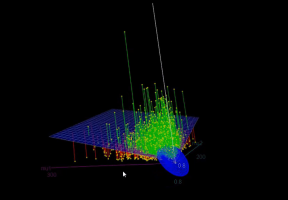
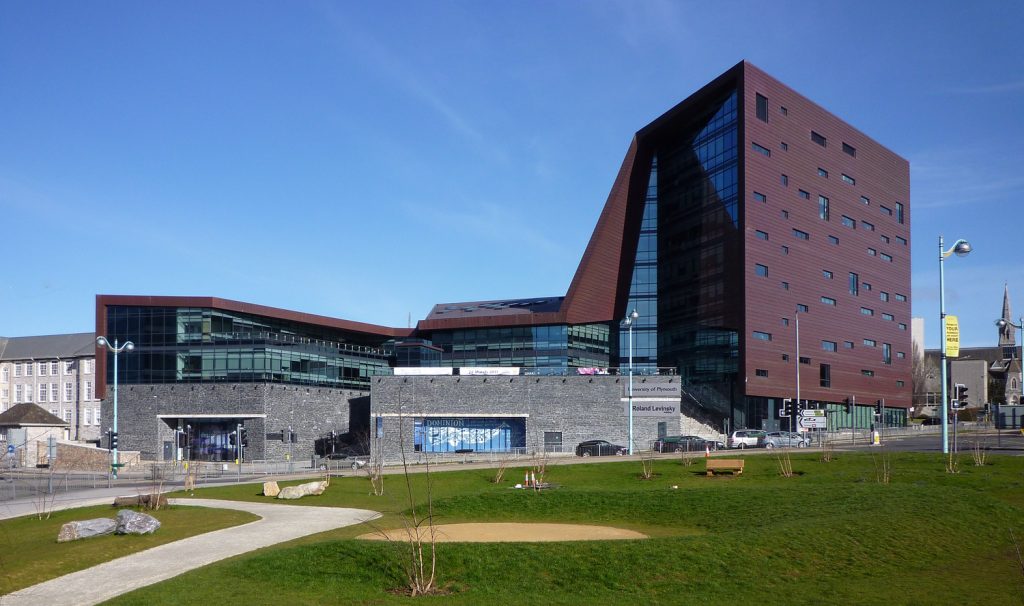
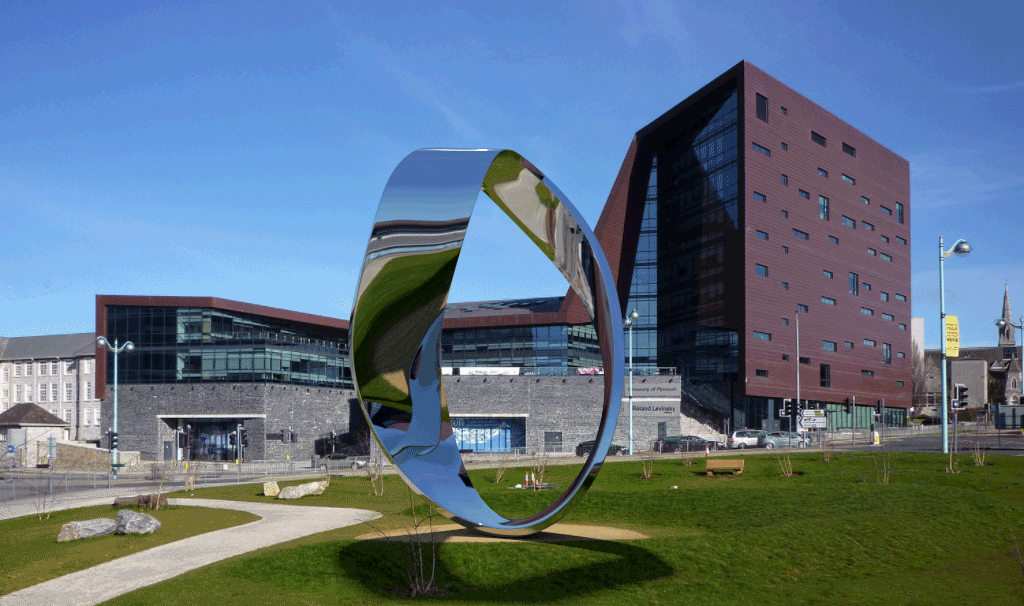
The Möbius band is an extraordinary geometrical figure. The band is eponymously named after the German mathematician August Ferdinand Möbius who described it in 1885, contemporaneously with another German mathematician named Johann Benedict Listing. It is a so called ruled surface with only one side and one boundary and it possesses the mathematical property of non-orientability (viz., a non-orientable manifold). In fact, the Möbius band is the simplest possible non-orientable surface. A Gedankenexperiment is helpful to understand this property intuitively: Imagine walking on the surface of a giant Möbius band. If you would travel long enough you would end up at the very starting point of the journey, only mirror-reversed. This journey can be repeated - ad infinitum. I argue that the Möbius band provides a reasily accessible metaphor for dual-aspect monism, a theory which challenges the predominant view that mind & matter (i.e., psyche & physis) are two fundamentally different substances. More information can be found on my eponymous websites.
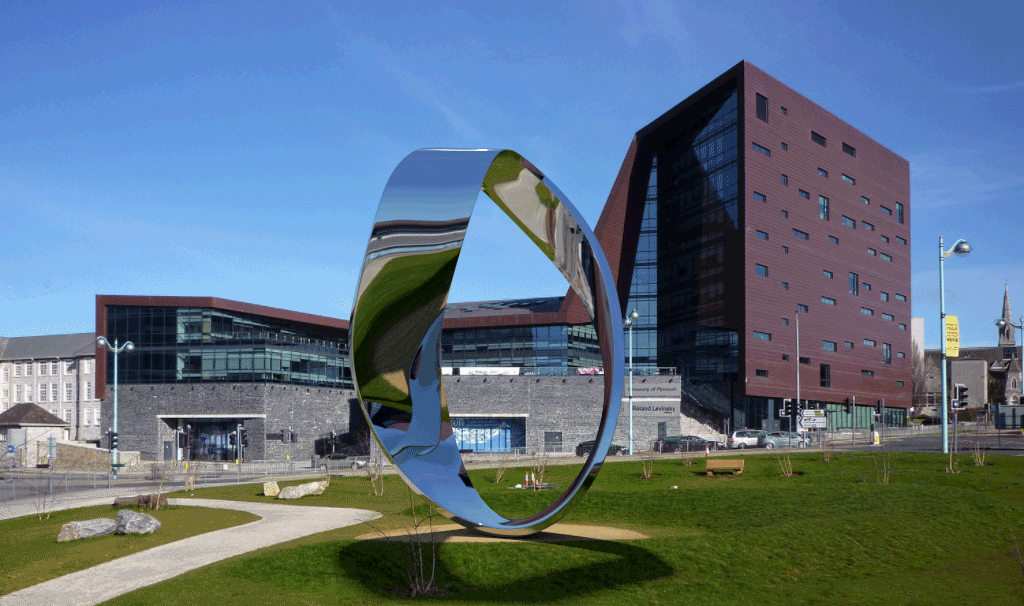
California, San Jose (Silicon Valley)
2016
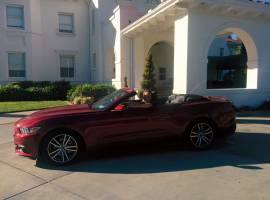
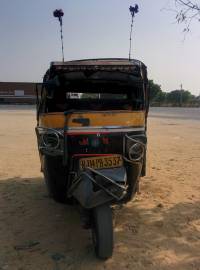
Saraswati Namastubhyam Varade Kama Rupini
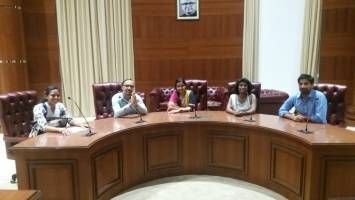
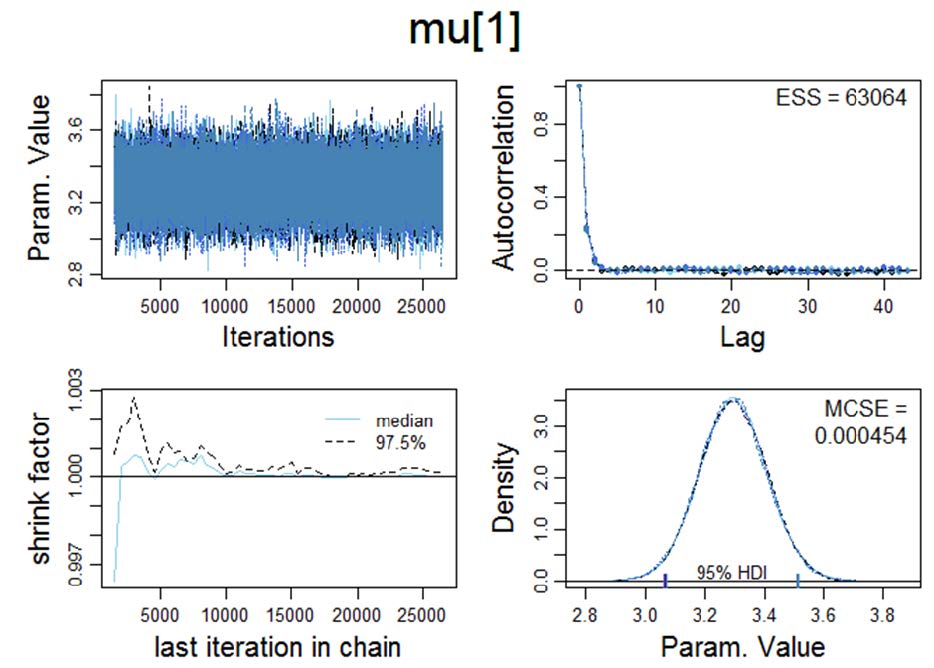
Trace plot: In order to examine the representativeness of the MCMC samples, we first visually examine the trajectory of the chains. The trace plot indicates convergence on θ, i.e., the trace plot appears to be stationary because its mean and variance are not changing as a function of time.
Shrink factor (Brooks-Gelman-Rubin statistic). Theoretically, the larger the number of iterations T, the closer 𝑅 should approximate 1 (as T → ∞, 𝑅→ 1).
Autocorrelation (effective sample size/EES)
The density plot entails the 95% HDI and it displays the numerical value of the Monte Carlo Standard Error (MCSE) of 0.000454. The Monte Carlo Error (MCSE) is the uncertainty which can be attributed to the fact that the number of simulation draws is always finite. In other words, it provides a quantitative index that represents the quality of parameter estimates. The MCSE package in R provides convenient tools for computing Monte Carlo standard errors and the effective sample size (Gelman et al., 2004).
#clears all of R's memory rm(list=ls()) # Get the functions loaded into R's working memory # The function can also be downloaded from the following URL: # http://irrational-decisions.com/?page_id=1996 source("BEST.R") #download data from server dataexp2 <- read.table("http://www.irrational-decisions.com/phd-thesis/dataexp2.txt", header=TRUE, sep="", na.strings="NA", dec=".", strip.white=TRUE) # Specify data as vectors y1 = c(dataexp2$v00) y2 = c(dataexp2$v01) # Run the Bayesian analysis using the default broad priors described by Kruschke (2013) mcmcChain = BESTmcmc( y1 , y2 , priorOnly=FALSE , numSavedSteps=12000 , thinSteps=1 , showMCMC=TRUE ) postInfo = BESTplot( y1 , y2 , mcmcChain , ROPEeff=c(-0.1,0.1) ) #The function “BEST.R” can be downloaded from the CRAN (Comprehensive R Archive Network) repository under https://cran.r-project.org/web/packages/BEST/index.html
Plain numerical DOI: 10.3758/s13423-016-1221-4
DOI URL
directSciHub download
Show/hide publication abstract
Plain numerical DOI: 10.1177/1745691611406925
DOI URL
directSciHub download
Show/hide publication abstract
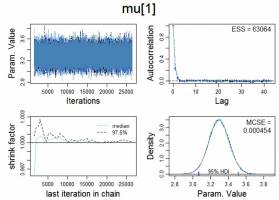
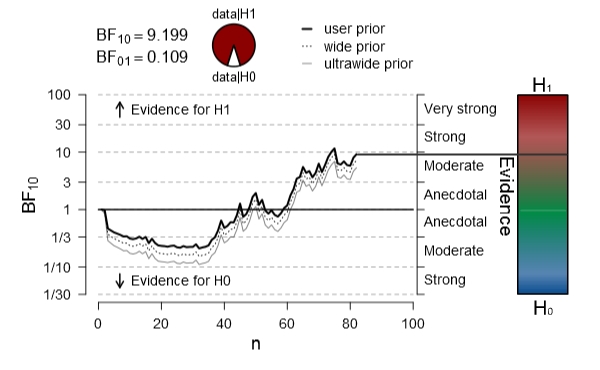
Plain numerical DOI: 10.3758/s13423-017-1323-7
DOI URL
directSciHub download
Show/hide publication abstract
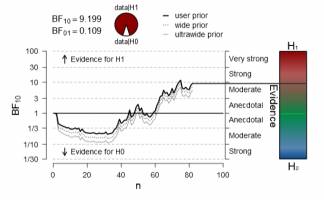
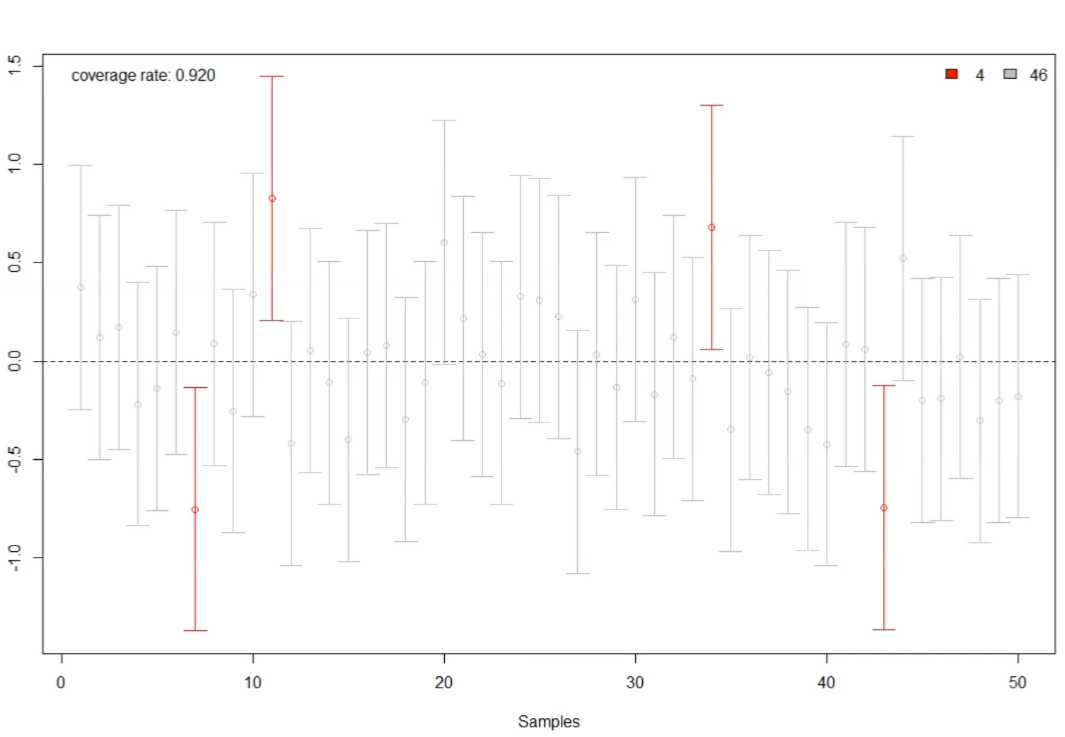
This animation which was created using R provides an intuitive explanation of what a 95% confidence interval really means: If we would repeat the exact same experiment 50 times, then 95 % of the time the 50 confidence intervals would contain the true mean. Visualisations are a powerful aid to understanding statistics. This makes sense from an evolutionary point of view as abstract symbolic cognition is phylogenetically much more recent than visual reasoning.
The American Psychological Association recommend confidence intervals as the "new statistics" in order to counteract the problems associuated with p-values. However, research clearly shows that confidence intervals are also widely misunderstood and they are therefore no real solution to the statistical crisis.
Out of 118 researcher only 3% were able to give the correct answer.
http://www.ejwagenmakers.com/inpress/HoekstraEtAlPBR.pdf
> library(animation) > conf.int function (level = 0.95, size = 50, cl = c("red", "gray"), ...) { n = ani.options("nmax") d = replicate(n, rnorm(size)) m = colMeans(d) ....
Plain numerical DOI: 10.3758/s13423-013-0572-3
DOI URL
directSciHub download
Show/hide publication abstract
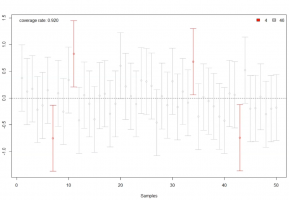
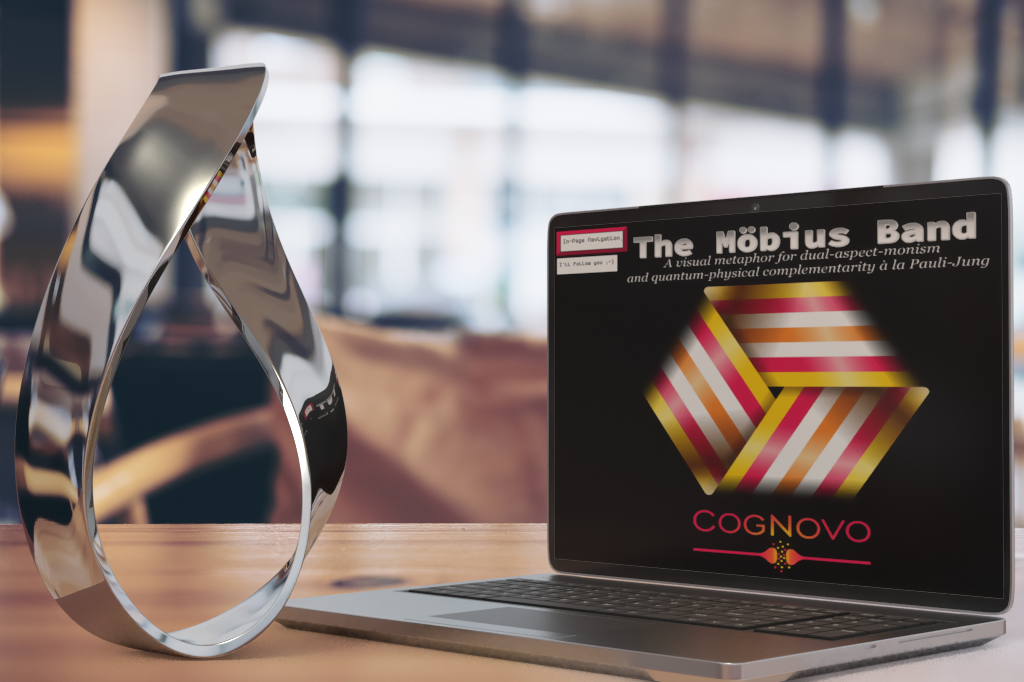
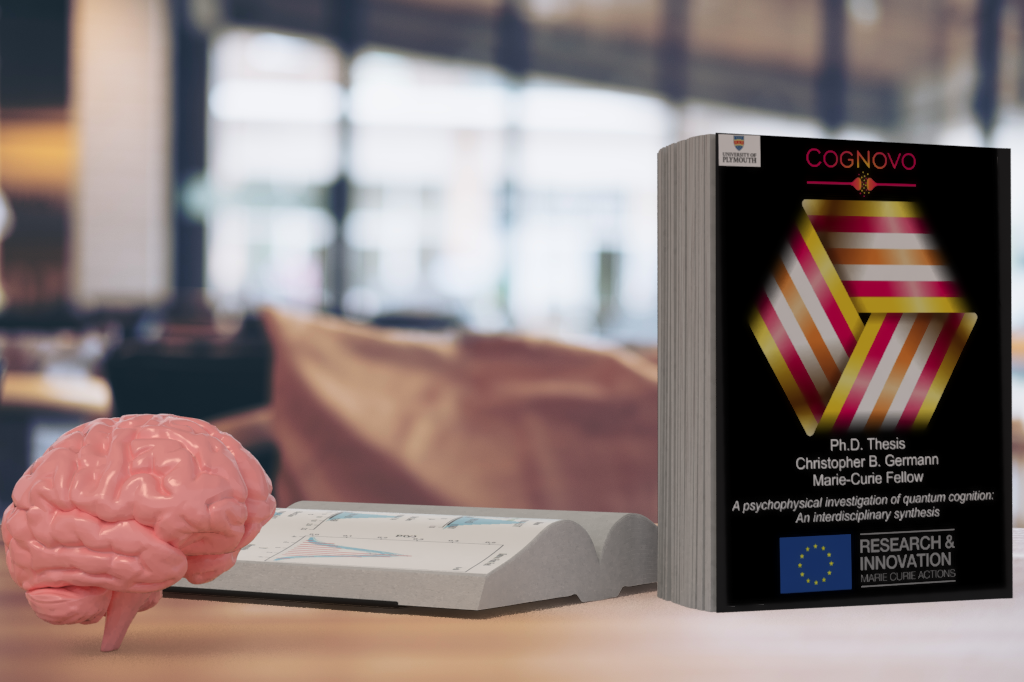
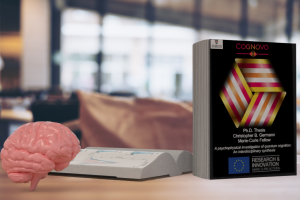
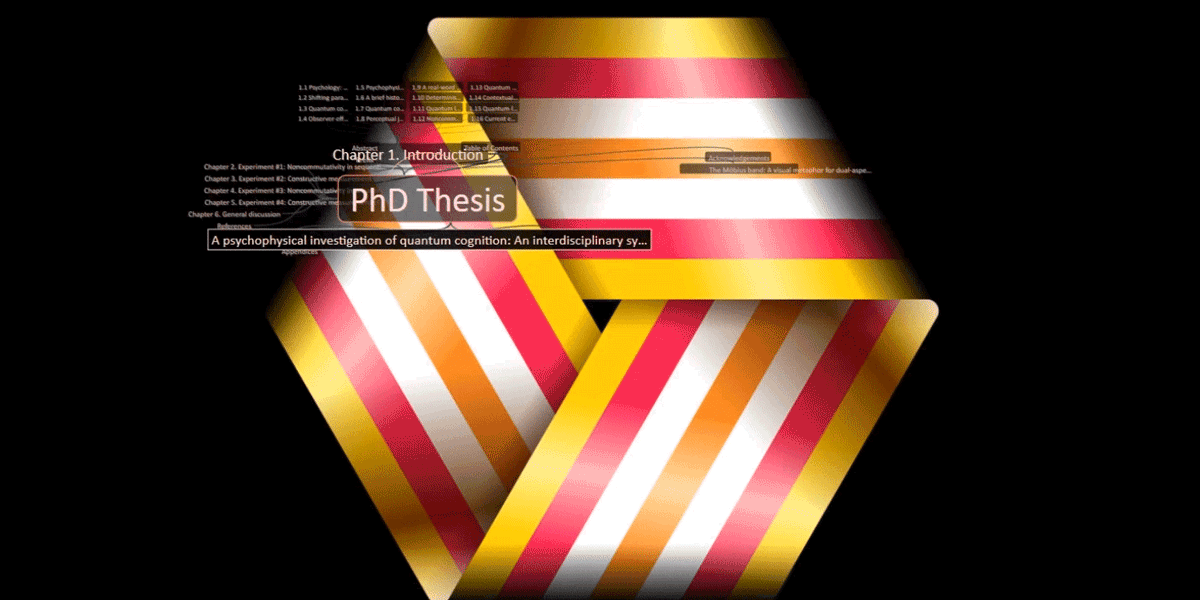
This is my beautiful "steel tongue drum" (a fantastic handmade percussion instrument). It creates amazing sounds (the harmonic scale is D-minor consisting of the pitches 𝄞 D, E, F, G, A, B♭). The tonality deeply penetrates the mind. Listen to it for yourself and enjoy the vibrations... 🎜
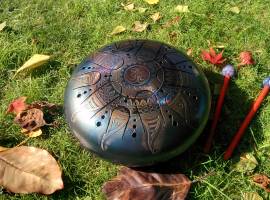
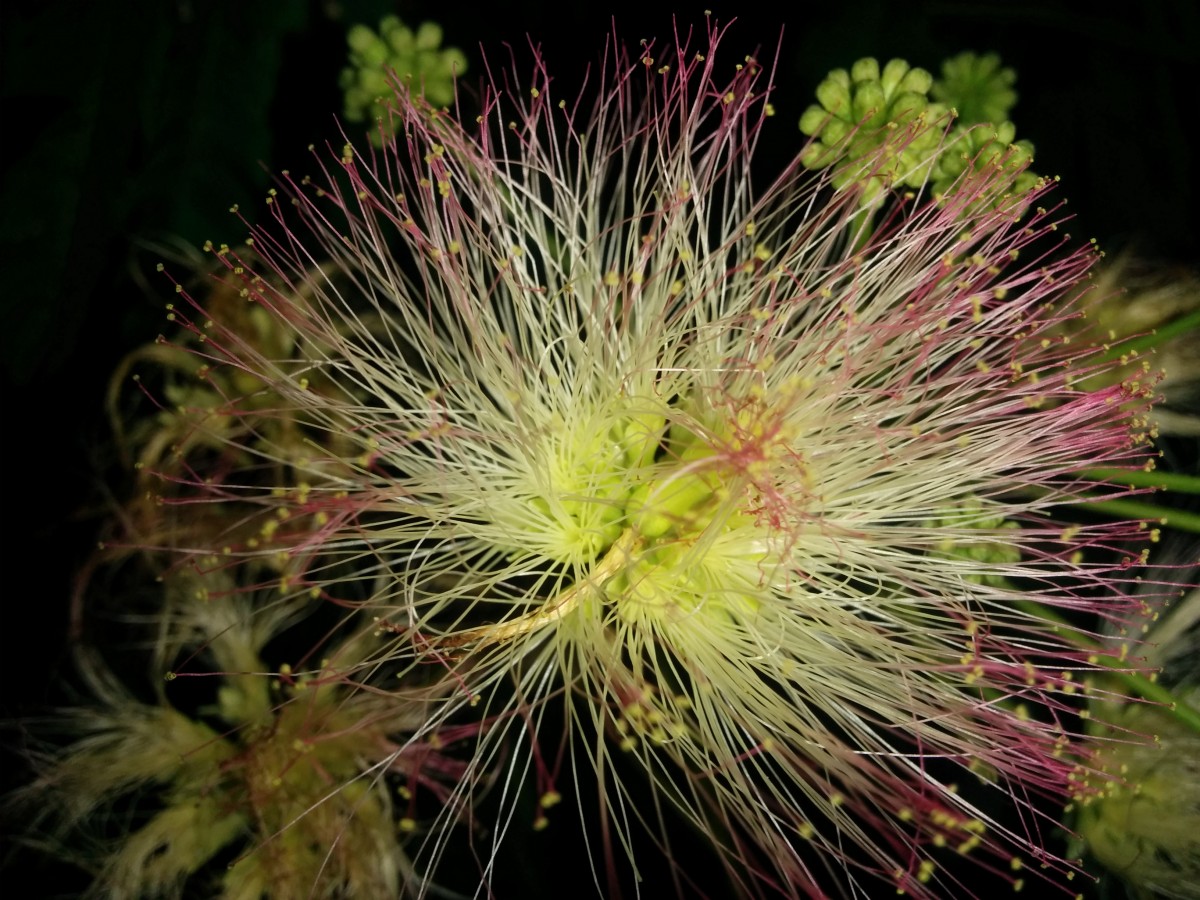
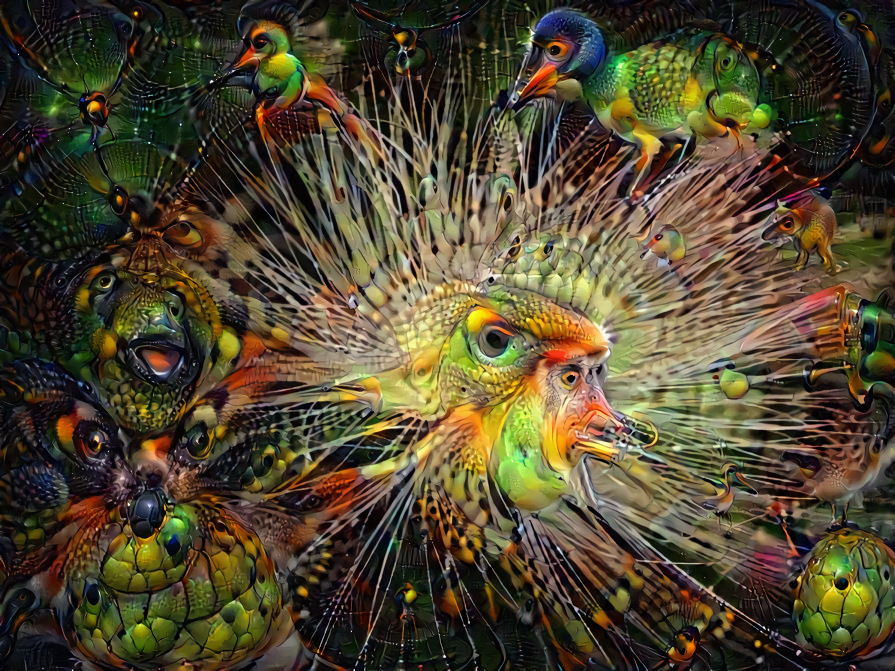
This is the flower of Albizia julibrissin, the Persian silk tree. I took this picture in 2016 in Plymouth, English Garden, Mount Edgcumbe. Not to be confused with Calliandra angustifolia aka. Bobinsana (a "healing plant" used in shamanism) which is in the Fabaceae family, too.
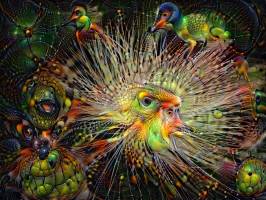
I created this website because cognitive liberty (freedom of thought) is a fundamental human right which is currently under heavy attack. The website focuses on neuropolitics, history, social psychology, and cognitive psychology, inter alia.
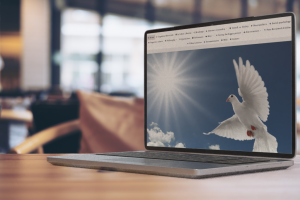
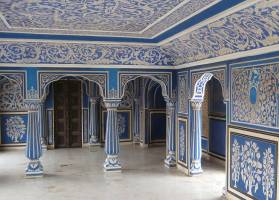
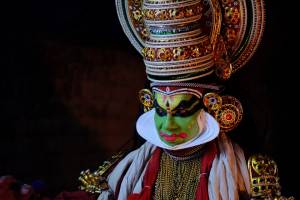
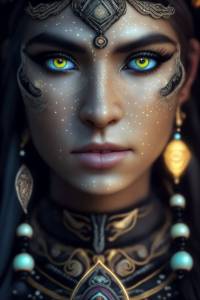
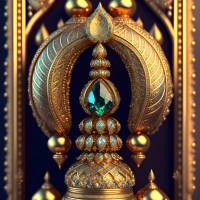
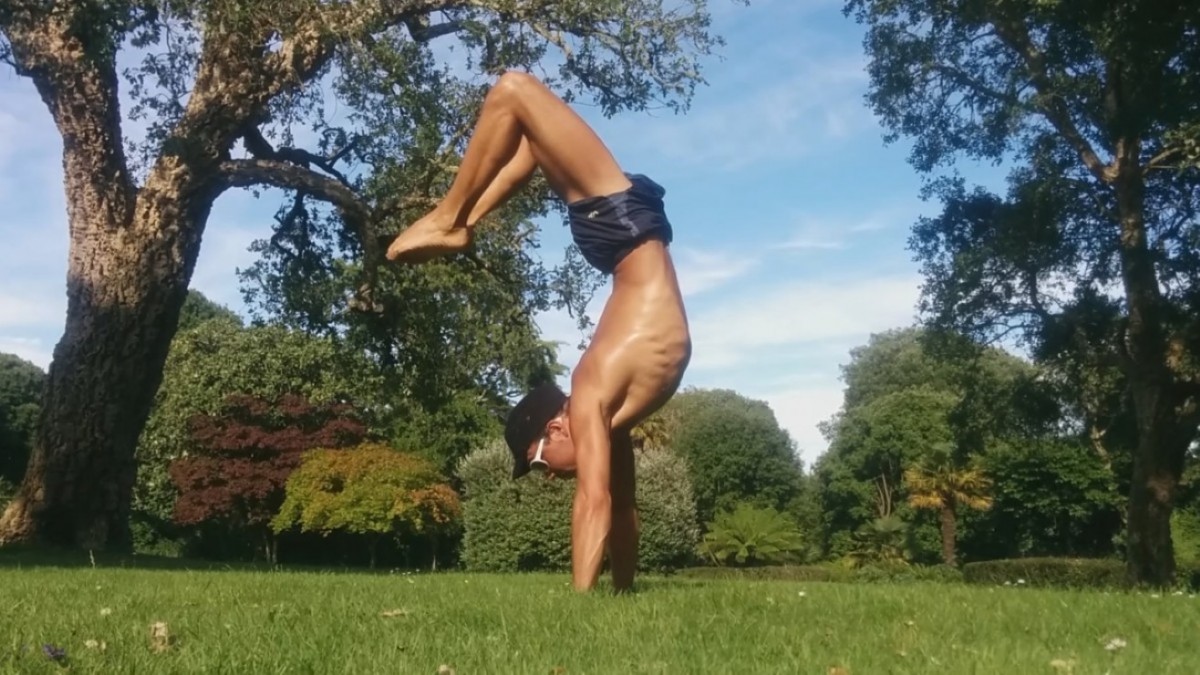
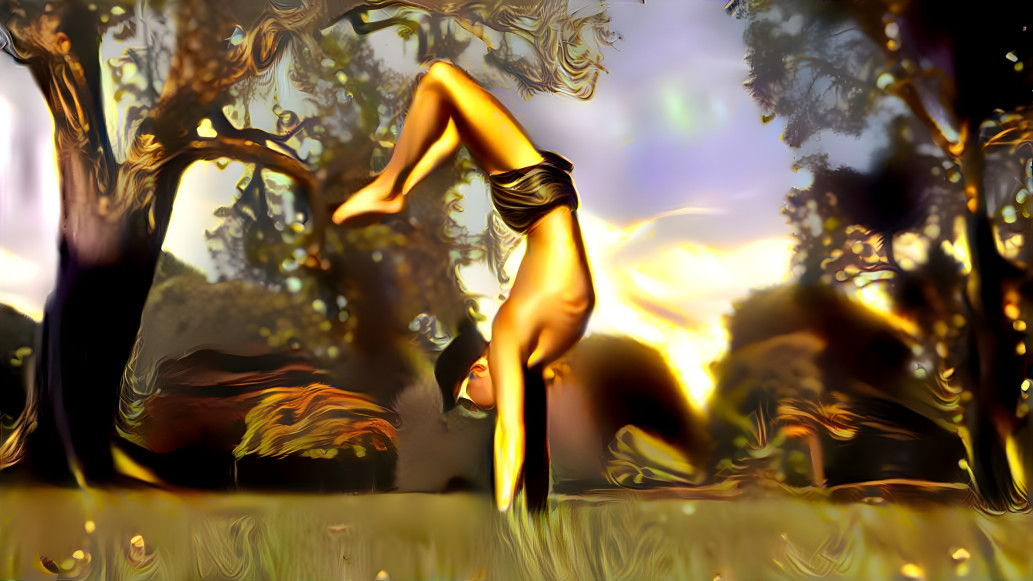
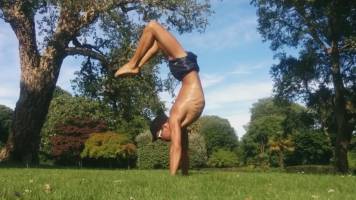
Sanskrit etymology:
nata = actor, dancer, mime
raja = king
asana = posture, seat
Nataraja is another name for Shiva and his dance symbolizes cosmic energy. It is a challenging balancing asana (cerebellum) which trains focus and concentration (self-control/prefrontal executive functions). It is an extroverted asana full of creative energy.
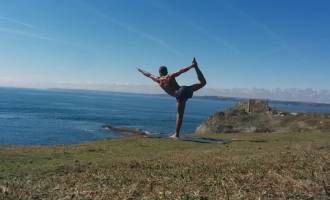
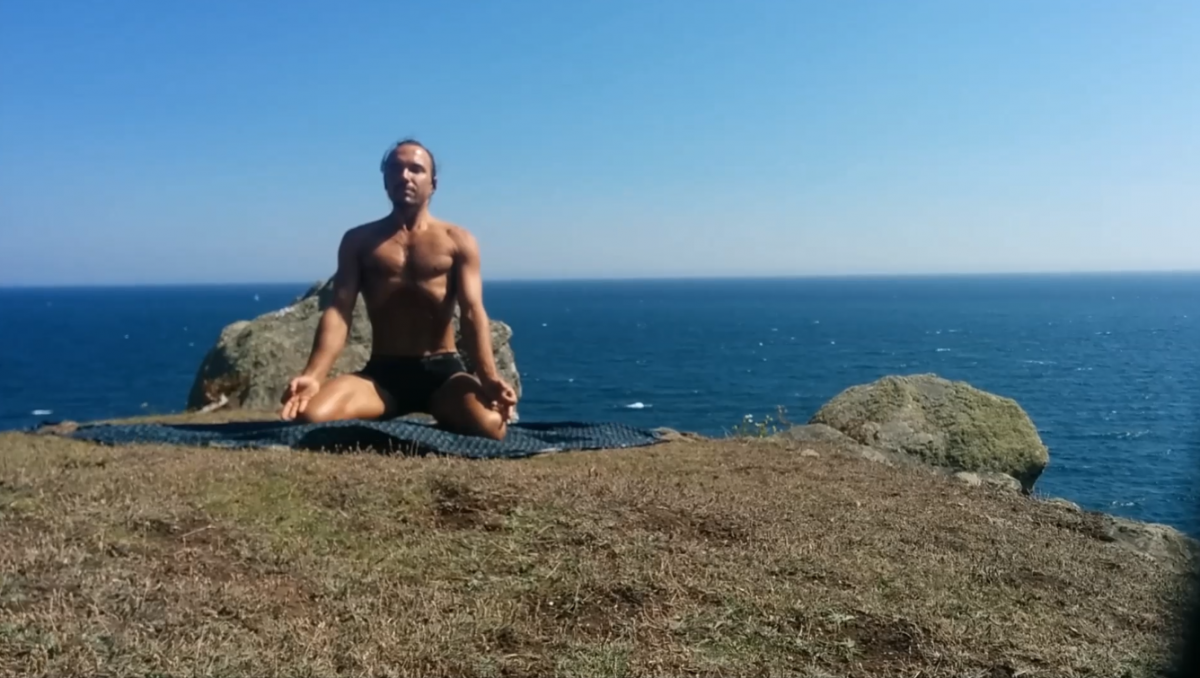
Click to close this text
The word psyche is etymologically derived from the ancient Greek ψυχή (psukhḗ, which translates into “mind/soul/spirit/breath”). The suffix "logia" (λογία) can be translated as "the study of" (cf. lógos/λόγος which can be be translated, inter alia, as "subject matter Hence, psychology literally means "the study of mind/soul/spirit/breath”. However, many psychologists are utterly nescient of this etymological definition and are not very comfortable with these profound philosophical concepts. There are some laudable exceptions, for instance, the Swiss depth-psychologist C.G. Jung who wrote extensively on Indian psychology and pranayama (viz., psycho-spiritual breathing-exercises). Jung also coined the terms introversion/extroversion which are today widely utilised in mainstream psychology (the 5-factor model of personality). It should be noted that there is no science without philosophy!The notion that science can be seperated from philosophy is a naïve positivistic illusion which completly neglects the history of science. Until quite recently science was philosophy. The terminological dichotomisation is a modern invention.Daniel Dennett formulated the following concise statement: “There is no such thing as philosophy-free science; there is only science whose philosophical baggage is taken on board without examination." — Daniel Dennett, Darwin's Dangerous Idea, 1995
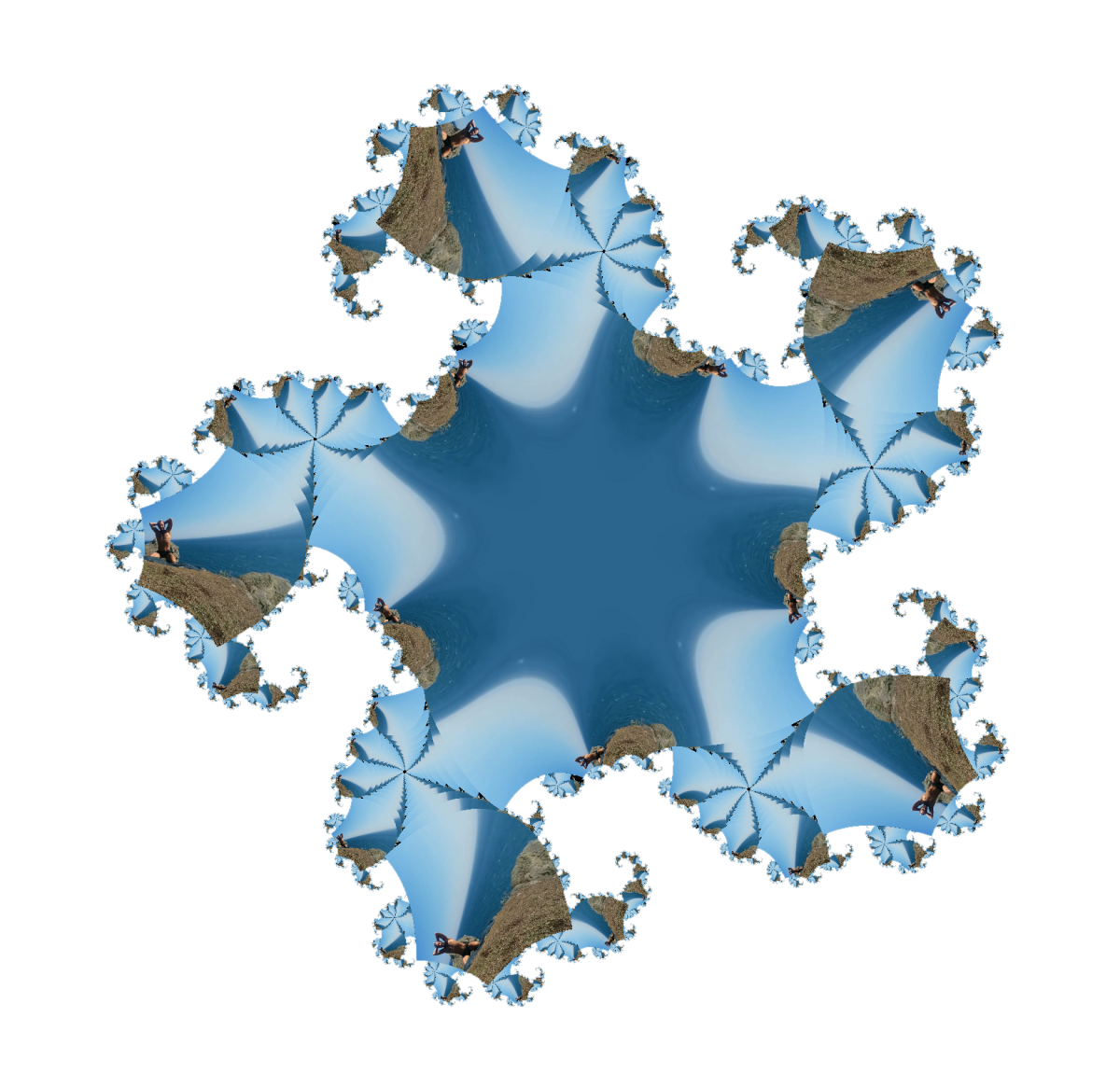
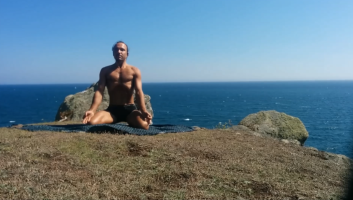
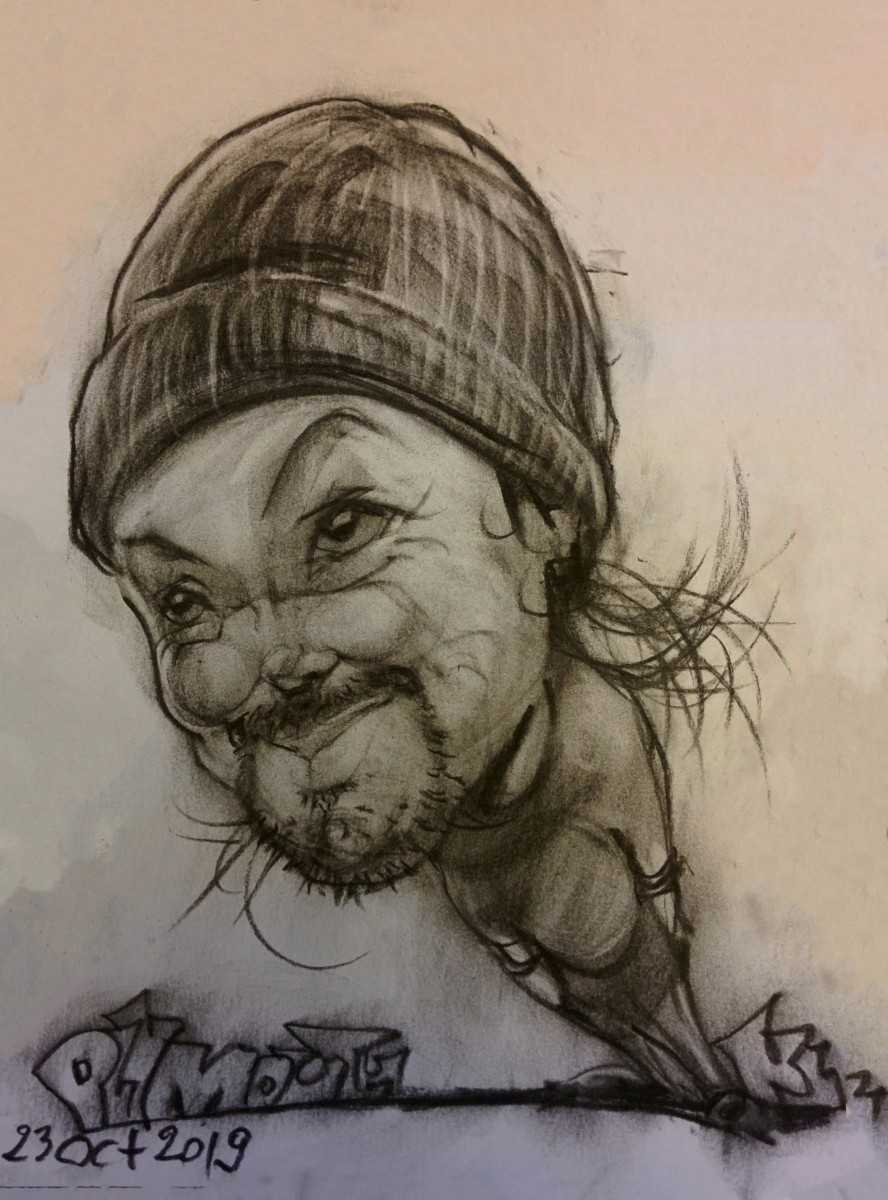
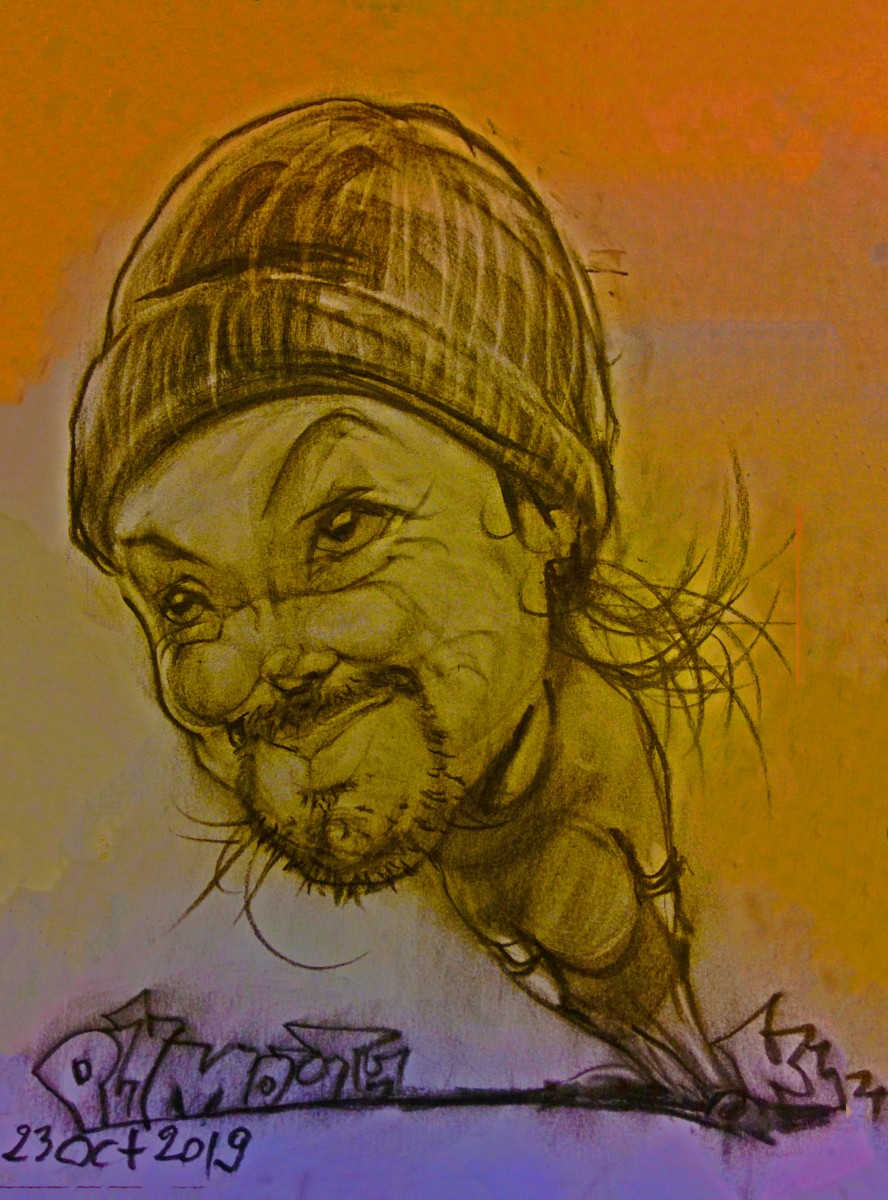
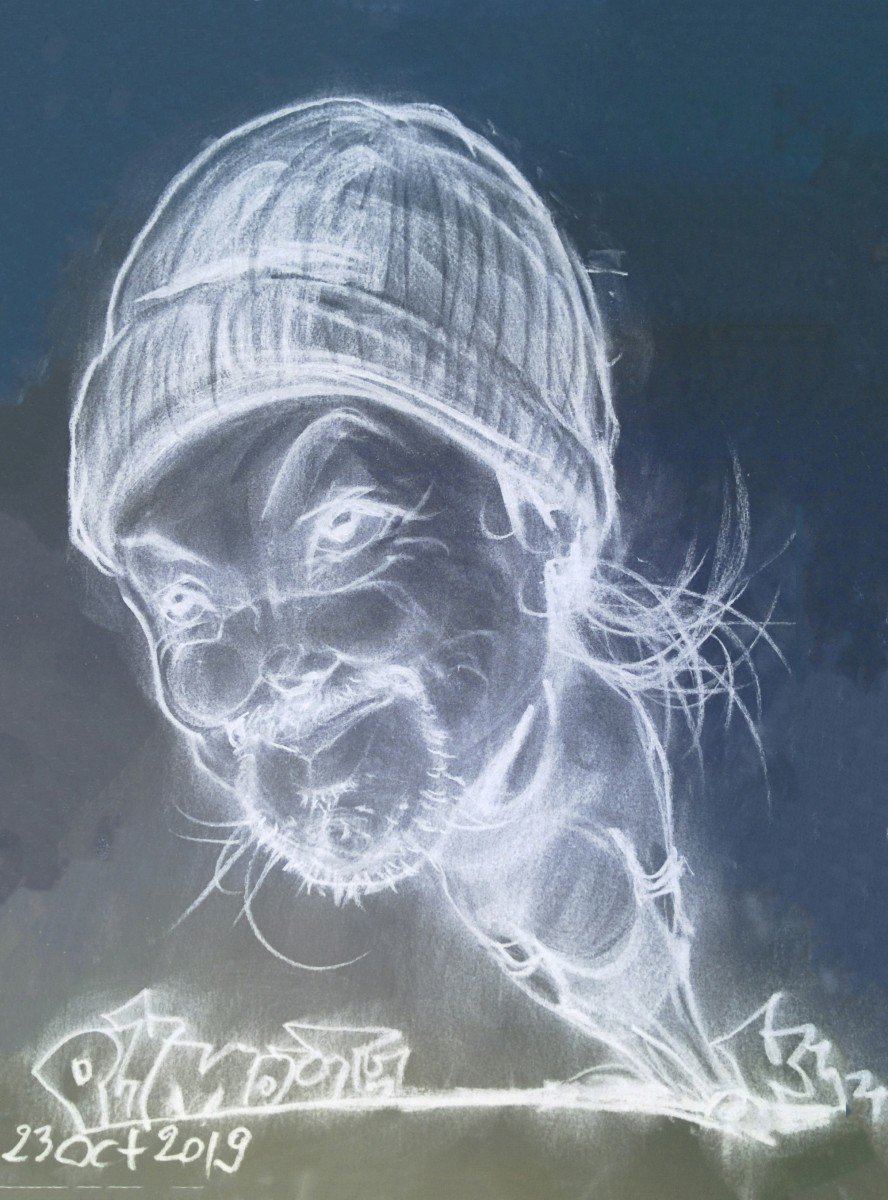
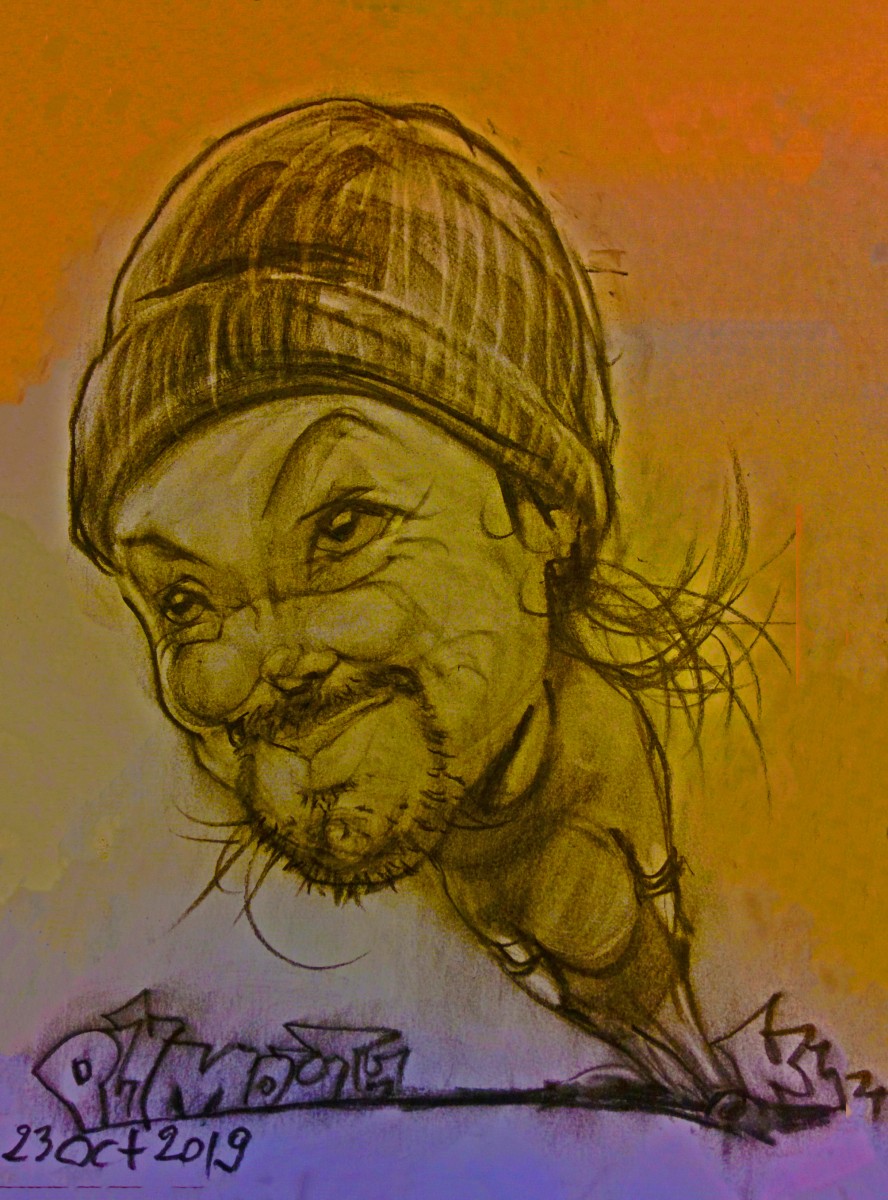
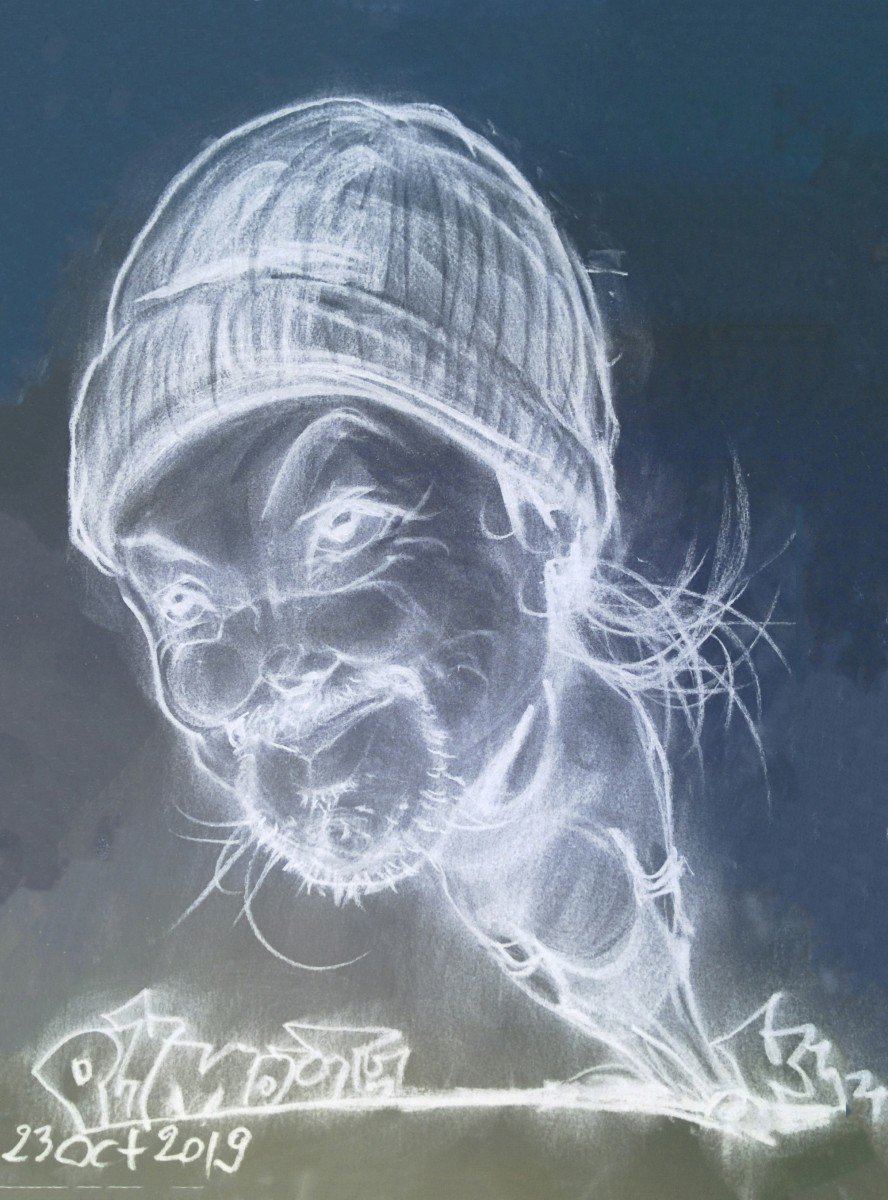
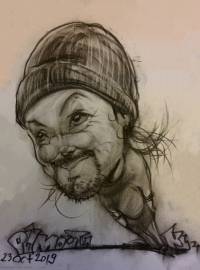
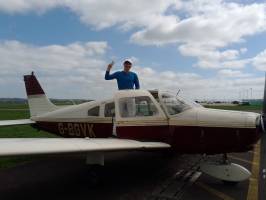
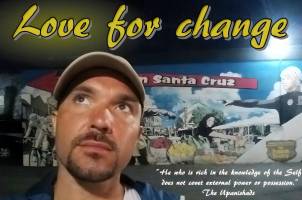
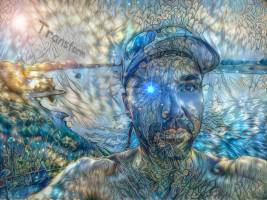
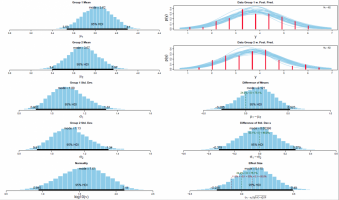
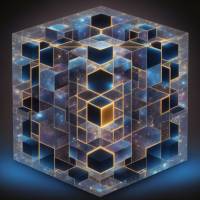
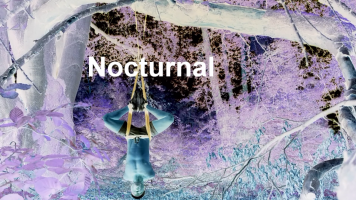
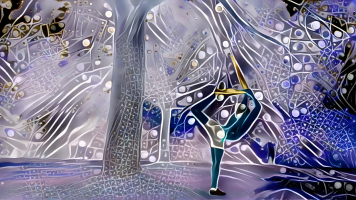
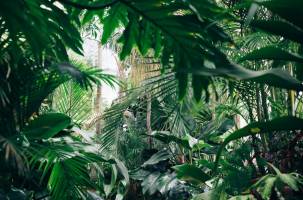
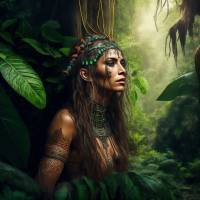
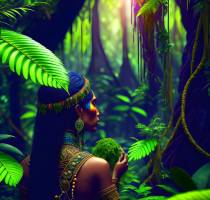
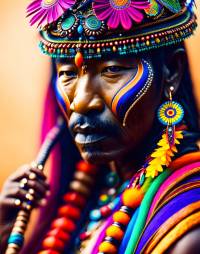
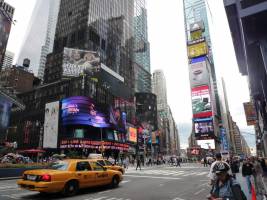
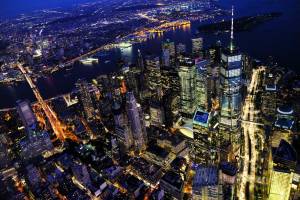
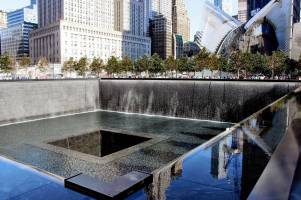
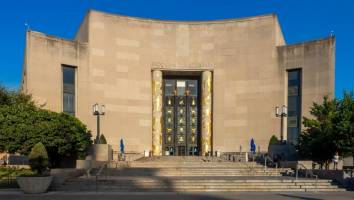
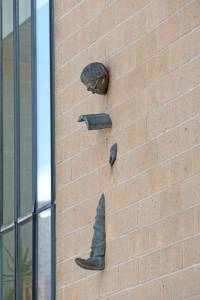
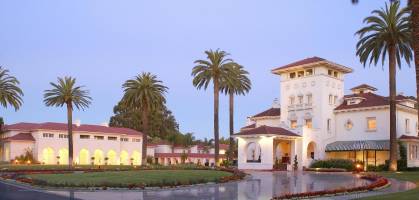
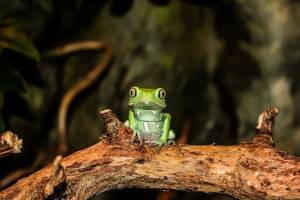
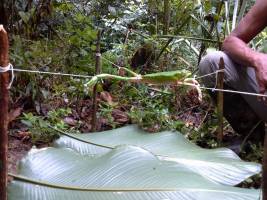
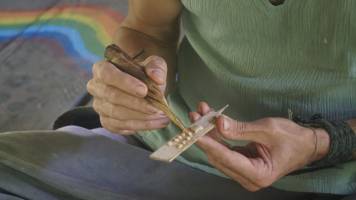
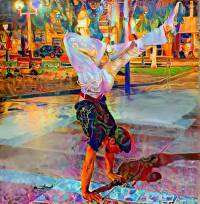
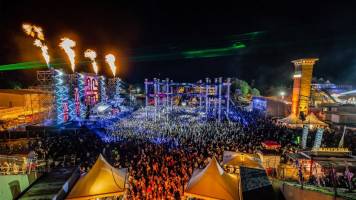
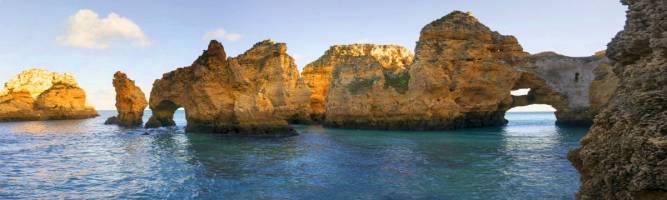

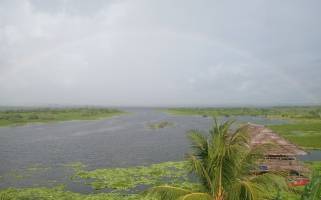
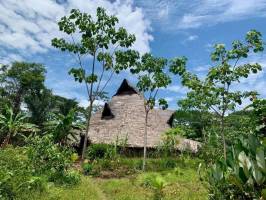
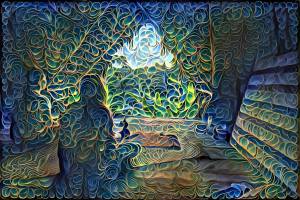
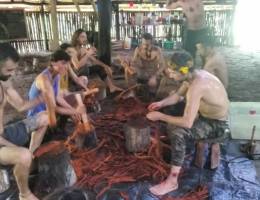
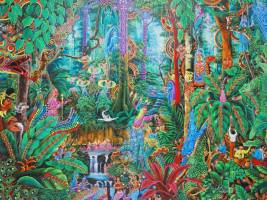
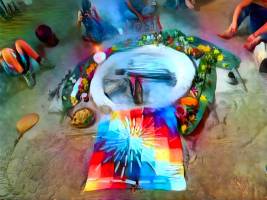
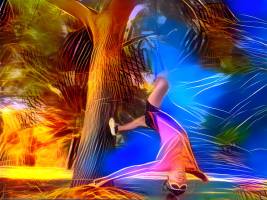
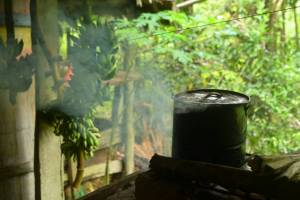
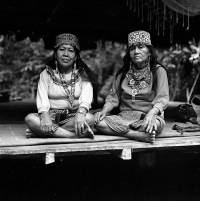
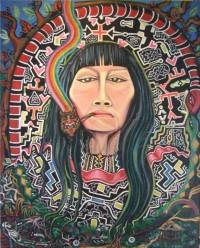
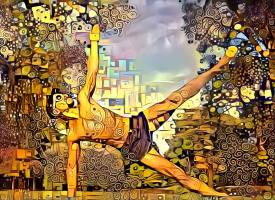
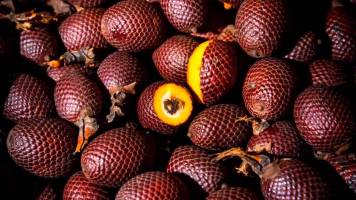
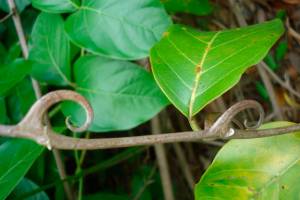
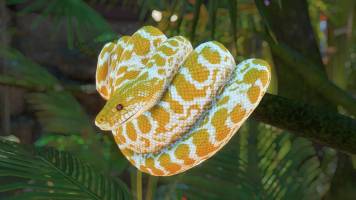
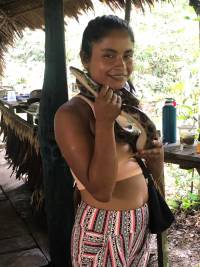
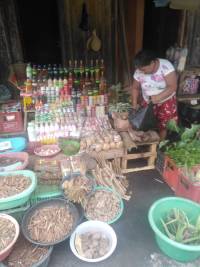
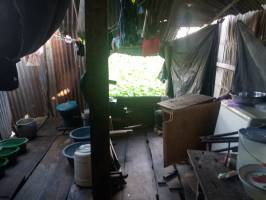
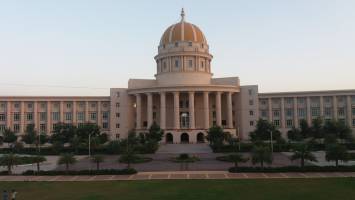
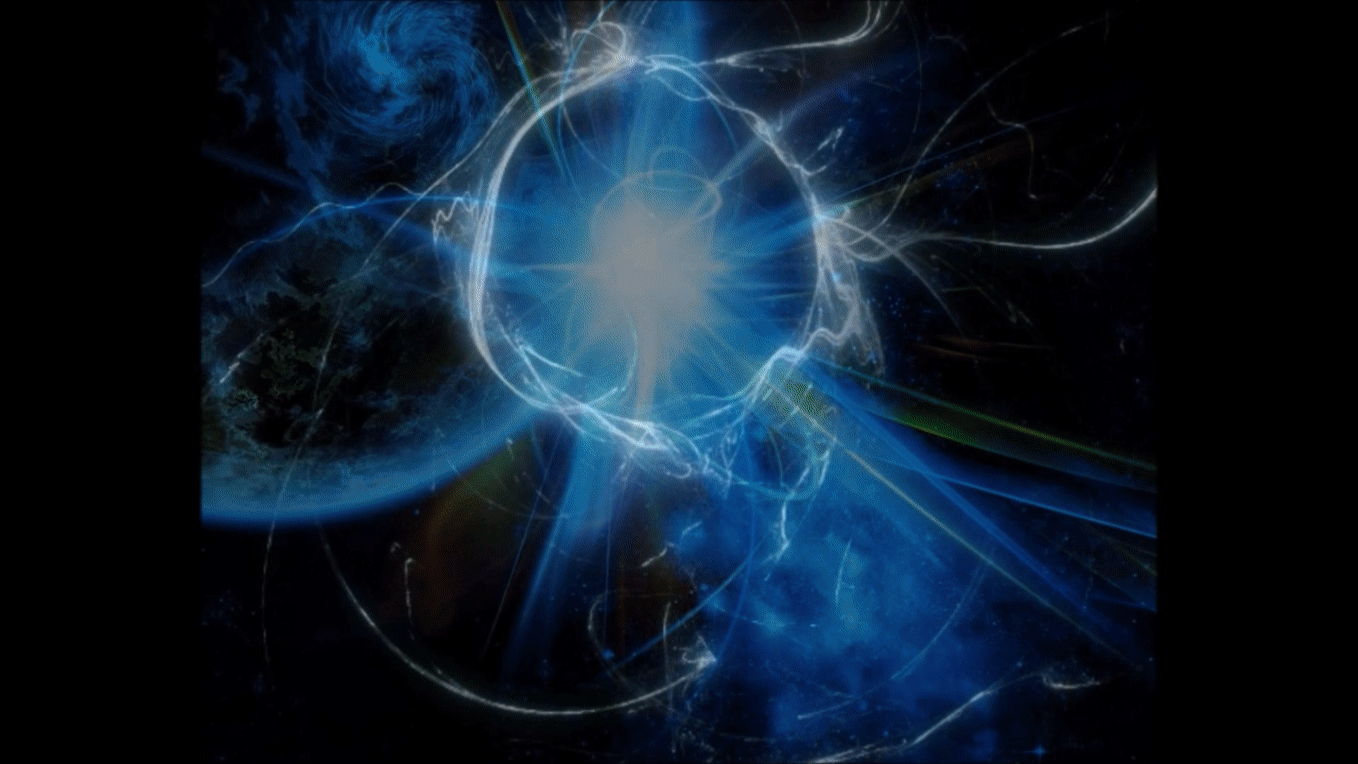
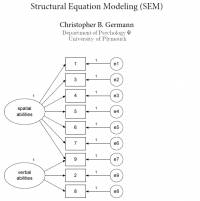
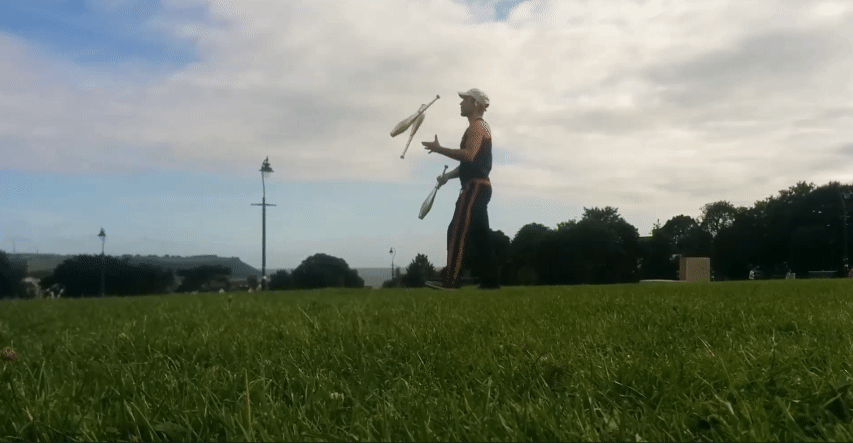
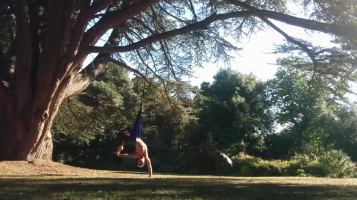
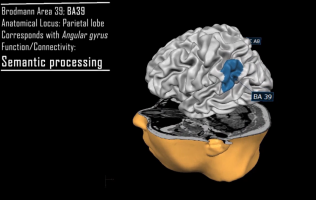
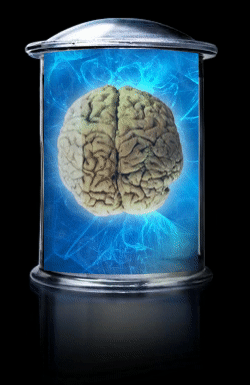
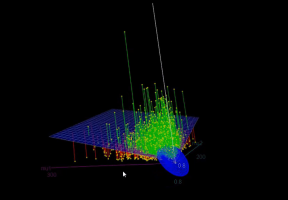
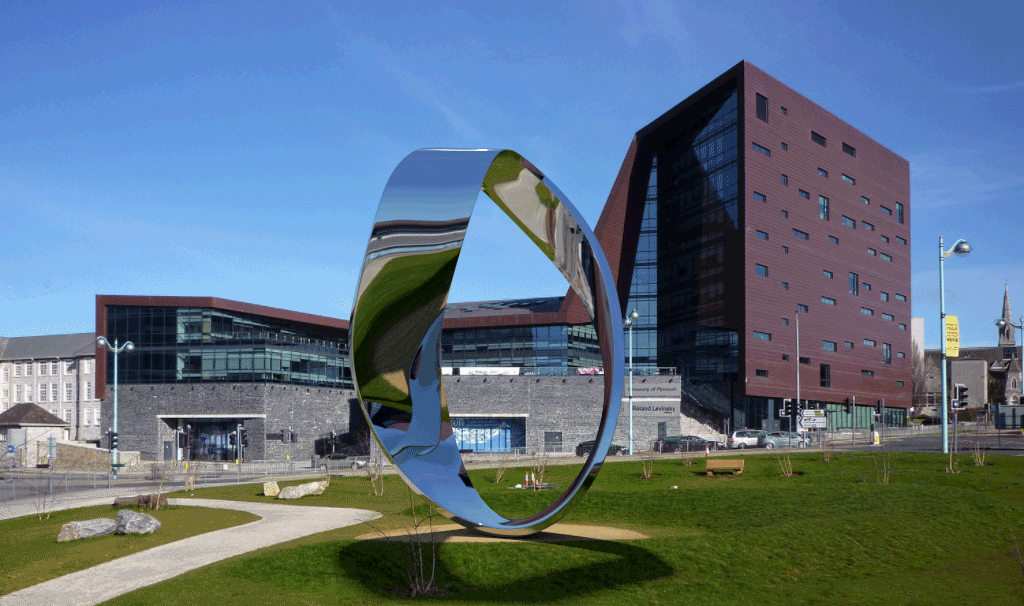
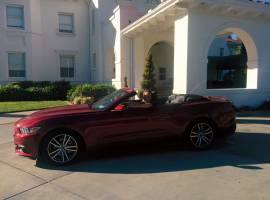
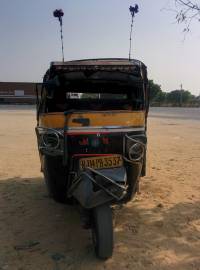
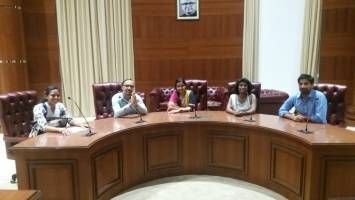
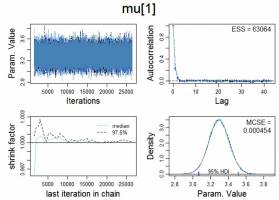
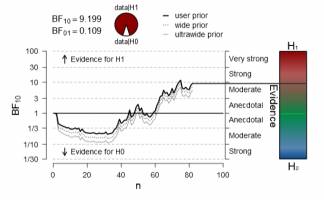
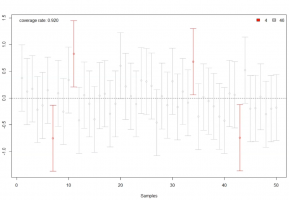
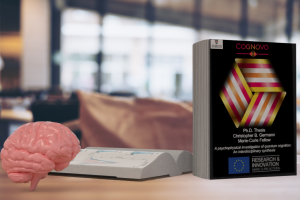
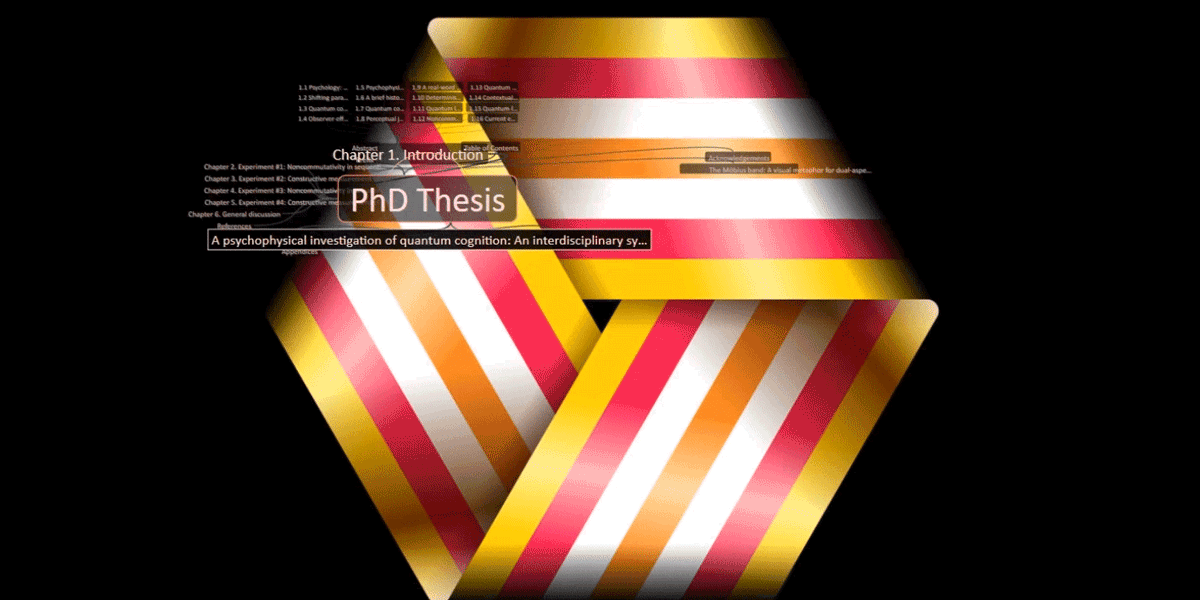
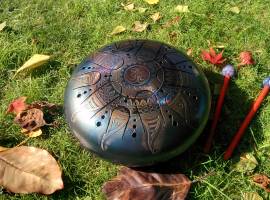
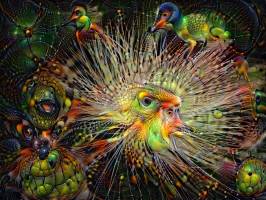
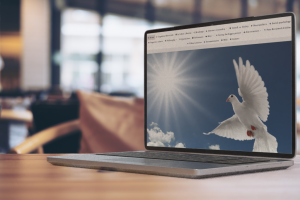
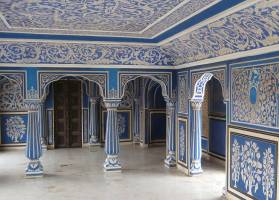
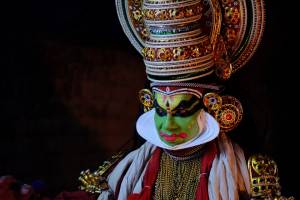
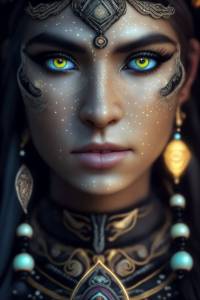
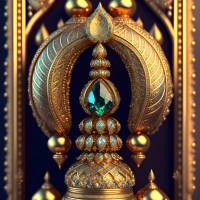
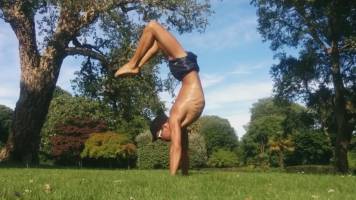
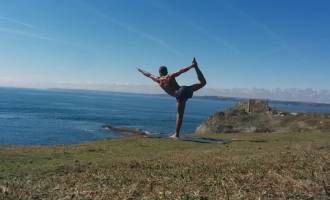
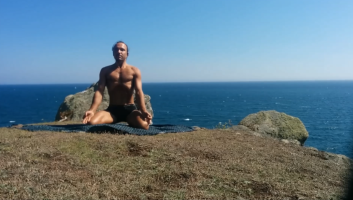
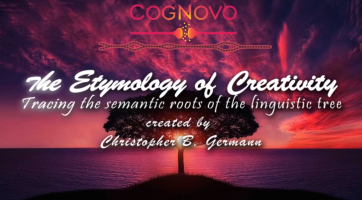
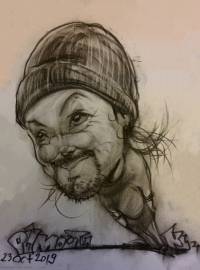

Posts & Pages
R code
Keywords: Statistical computingOpen-source softwareBayesian analysisMarkov chain Monte Carlo methodsData visualisationLogical inferenceHypothesis testingDeductive reasoningAbductionCredibility intervalsProbability theoryDecision algorithmsNew statisticsReplication crisisCreative statistics
data(ToothGrowth) ## Example plot from ?ToothGrowth coplot(len ~ dose | supp, data = ToothGrowth, panel = panel.smooth, xlab = "ToothGrowth data: length vs dose, given type of supplement") ## Treat dose as a factor ToothGrowth$dose = factor(ToothGrowth$dose) levels(ToothGrowth$dose) = c("Low", "Medium", "High") summary(aov(len ~ supp*dose, data=ToothGrowth)) #install.packages("xtable") library(xtable) xtable(x, caption = NULL, label = NULL, align = NULL, digits = NULL, display = NULL, auto = FALSE, ...) print(xtable(d), type="html") print(xtable(d), type="latex") # anova table to latex #https://cran.r-project.org/web/packages/xtable/index.html #https://rmarkdown.rstudio.com/
data(ToothGrowth) # model log2 of dose instead of dose directly ToothGrowth$dose = log2(ToothGrowth$dose) # Classical analysis for comparison lmToothGrowth <- lm(len ~ supp + dose + supp:dose, data=ToothGrowth) summary(lmToothGrowth)
x<- (1:5) y<- (1:111) pdf(file=file.choose()) hist(x) plot(x, type='o') dev.off()
Notes
file.choose()
is a very handy command which saves the work associated with defining absolute and relative paths which can be quite cumbersome.
list.files
for non-interactive selection.
choose.files
for selecting multiple files interactively.
More
https://www.rdocumentation.org/packages/base/versions/3.5.2/topics/file.choose
# http://wiki.math.yorku.ca/index.php/VR4:_Chapter_1_summary x <- seq( 1, 20, 0.5) # sequence from 1 to 20 by 0.5 x # print it w <- 1 + x/2 # a weight vector y <- x + w*rnorm(x) # generate y with standard deviations # equal to w dum <- data.frame( x, y, w ) # make a data frame of 3 columns dum # typing it's name shows the object rm( x, y, w ) # remove the original variables fm <- lm ( y ~ x, data = dum) # fit a simple linear regression (between x and y) summary( fm ) # and look at the analysis fm1 <- lm( y ~ x, data = dum, weight = 1/w^2 ) # a weighted regression summary(fm1) lrf <- loess( y ~ x, dum) # a smooth regression curve using a # modern local regression function attach( dum ) # make the columns in the data frame visible # as variables (Note: before this command, typing x and # y would yield errors) plot( x, y) # a standard scatterplot to which we will # fit regression curves lines( spline( x, fitted (lrf)), col = 2) # add in the local regression using spline # interpolation between calculated # points abline(0, 1, lty = 3, col = 3) # add in the true regression line (lty=3: read line type=dotted; # col=3: read colour=green; type "?par" for details) abline(fm, col = 4) # add in the unweighted regression line abline(fm1, lty = 4, col = 5) # add in the weighted regression line plot( fitted(fm), resid(fm), xlab = "Fitted Values", ylab = "Residuals") # a standard regression diagnostic plot # to check for heteroscedasticity. # The data were generated from a # heteroscedastic process. Can you see # it from this plot? qqnorm( resid(fm) ) # Normal scores to check for skewness, # kurtosis and outliers. How would you # expect heteroscedasticity to show up? search() # Have a look at the search path detach() # Remove the data frame from the search path search() # Have another look. How did it change? rm(fm, fm1, lrf, dum) # Clean up (this is not that important in R # for reasons we will understand later) library(rgl) rgl.open() rgl.bg(col='white') x <- sort(rnorm(1000)) y <- rnorm(1000) z1 <- atan2(x,y) z <- rnorm(1000) + atan2(x, y) plot3d(x, y, z1, col = rainbow(1000), size = 2) bbox3d()
skew(x, na.rm = TRUE) kurtosi(x, na.rm = TRUE) #x A data.frame or matrix #na.rm how to treat missing data ## The function is currently defined as function (x, na.rm = TRUE) { if (length(dim(x)) == 0) { if (na.rm) { x <- x[!is.na(x)] } mx <- mean(x) sdx <- sd(x,na.rm=na.rm) kurt <- sum((x - mx)^4)/(length(x) * sd(x)^4) -3 } else { kurt <- rep(NA,dim(x)[2]) mx <- colMeans(x,na.rm=na.rm) sdx <- sd(x,na.rm=na.rm) for (i in 1:dim(x)[2]) { kurt[i] <- sum((x[,i] - mx[i])^4, na.rm = na.rm)/((length(x[,i]) - sum(is.na(x[,i]))) * sdx[i]^4) -3 } } return(kurt) }
#https://richarddmorey.github.io/BayesFactor/ bf = ttestBF(x = diffScores) ## Equivalently: ## bf = ttestBF(x = sleep$extra[1:10],y=sleep$extra[11:20], paired=TRUE) bf
Comment
ttestBF function, which performs the “JZS” t test described by Rouder, Speckman, Sun, Morey, and Iverson (2009).
#https://richarddmorey.github.io/BayesFactor/ chains2 = recompute(chains, iterations = 10000) plot(chains2[,1:2])
Comment
The posterior function returns a object of type BFmcmc, which inherits the methods of the mcmc class from the coda package.
#https://richarddmorey.github.io/BayesFactor/ ## Compute Bayes factor bf = ttestBF(formula = weight ~ feed, data = chickwts) bf ### chains = posterior(bf, iterations = 10000) plot(chains[,2])
#https://richarddmorey.github.io/BayesFactor/ ## Bem's t statistics from four selected experiments t = c(-.15, 2.39, 2.42, 2.43) N = c(100, 150, 97, 99) ### ## Do analysis again, without nullInterval restriction bf = meta.ttestBF(t=t, n1=N, rscale=1) ## Obtain posterior samples chains = posterior(bf, iterations = 10000) plot(chains)
Comment
ttestBF function, which performs the “JZS” t test described by Rouder, Speckman, Sun, Morey, and Iverson (2009).
## This source code is licensed under the FreeBSD license ## (c) 2013 Felix Schönbrodt #' @title Plots a comparison of a sequence of priors for t test Bayes factors #' #' @details #' #' #' @param ts A vector of t values #' @param ns A vector of corresponding sample sizes #' @param rs The sequence of rs that should be tested. r should run up to 2 (higher values are implausible; E.-J. Wagenmakers, personal communication, Aug 22, 2013) #' @param labels Names for the studies (displayed in the facet headings) #' @param dots Values of r's which should be marked with a red dot #' @param plot If TRUE, a ggplot is returned. If false, a data frame with the computed Bayes factors is returned #' @param sides If set to "two" (default), a two-sided Bayes factor is computed. If set to "one", a one-sided Bayes factor is computed. In this case, it is assumed that positive t values correspond to results in the predicted direction and negative t values to results in the unpredicted direction. For details, see Wagenmakers, E. J., & Morey, R. D. (2013). Simple relation between one-sided and two-sided Bayesian point-null hypothesis tests. #' @param nrow Number of rows of the faceted plot. #' @param forH1 Defines the direction of the BF. If forH1 is TRUE, BF > 1 speak in favor of H1 (i.e., the quotient is defined as H1/H0). If forH1 is FALSE, it's the reverse direction. #' #' @references #' #' Rouder, J. N., Speckman, P. L., Sun, D., Morey, R. D., & Iverson, G. (2009). Bayesian t-tests for accepting and rejecting the null hypothesis. Psychonomic Bulletin and Review, 16, 225-237. #' Wagenmakers, E.-J., & Morey, R. D. (2013). Simple relation between one-sided and two-sided Bayesian point-null hypothesis tests. Manuscript submitted for publication #' Wagenmakers, E.-J., Wetzels, R., Borsboom, D., Kievit, R. & van der Maas, H. L. J. (2011). Yes, psychologists must change the way they analyze their data: Clarifications for Bem, Utts, & Johnson (2011) BFrobustplot <- function( ts, ns, rs=seq(0, 2, length.out=200), dots=1, plot=TRUE, labels=c(), sides="two", nrow=2, xticks=3, forH1=TRUE) { library(BayesFactor) # compute one-sided p-values from ts and ns ps <- pt(ts, df=ns-1, lower.tail = FALSE) # one-sided test # add the dots location to the sequences of r's rs <- c(rs, dots) res <- data.frame() for (r in rs) { # first: calculate two-sided BF B_e0 <- c() for (i in 1:length(ts)) B_e0 <- c(B_e0, exp(ttest.tstat(t = ts[i], n1 = ns[i], rscale=r)$bf)) # second: calculate one-sided BF B_r0 <- c() for (i in 1:length(ts)) { if (ts[i] > 0) { # correct direction B_r0 <- c(B_r0, (2 - 2*ps[i])*B_e0[i]) } else { # wrong direction B_r0 <- c(B_r0, (1 - ps[i])*2*B_e0[i]) } } res0 <- data.frame(t=ts, n=ns, BF_two=B_e0, BF_one=B_r0, r=r) if (length(labels) > 0) { res0$labels <- labels res0$heading <- factor(1:length(labels), labels=paste0(labels, "\n(t = ", ts, ", df = ", ns-1, ")"), ordered=TRUE) } else { res0$heading <- factor(1:length(ts), labels=paste0("t = ", ts, ", df = ", ns-1), ordered=TRUE) } res <- rbind(res, res0) } # define the measure to be plotted: one- or two-sided? res$BF <- res[, paste0("BF_", sides)] # Flip BF if requested if (forH1 == FALSE) { res$BF <- 1/res$BF } if (plot==TRUE) { library(ggplot2) p1 <- ggplot(res, aes(x=r, y=log(BF))) + geom_line() + facet_wrap(~heading, nrow=nrow) + theme_bw() + ylab("log(BF)") p1 <- p1 + geom_hline(yintercept=c(c(-log(c(30, 10, 3)), log(c(3, 10, 30)))), linetype="dotted", color="darkgrey") p1 <- p1 + geom_hline(yintercept=log(1), linetype="dashed", color="darkgreen") # add the dots p1 <- p1 + geom_point(data=res[res$r %in% dots,], aes(x=r, y=log(BF)), color="red", size=2) # add annotation p1 <- p1 + annotate("text", x=max(rs)*1.8, y=-2.85, label=paste0("Strong~H[", ifelse(forH1==TRUE,0,1), "]"), hjust=1, vjust=.5, size=3, color="black", parse=TRUE) p1 <- p1 + annotate("text", x=max(rs)*1.8, y=-1.7 , label=paste0("Moderate~H[", ifelse(forH1==TRUE,0,1), "]"), hjust=1, vjust=.5, size=3, color="black", parse=TRUE) p1 <- p1 + annotate("text", x=max(rs)*1.8, y=-.55 , label=paste0("Anectodal~H[", ifelse(forH1==TRUE,0,1), "]"), hjust=1, vjust=.5, size=3, color="black", parse=TRUE) p1 <- p1 + annotate("text", x=max(rs)*1.8, y=2.86 , label=paste0("Strong~H[", ifelse(forH1==TRUE,1,0), "]"), hjust=1, vjust=.5, size=3, color="black", parse=TRUE) p1 <- p1 + annotate("text", x=max(rs)*1.8, y=1.7 , label=paste0("Moderate~H[", ifelse(forH1==TRUE,1,0), "]"), hjust=1, vjust=.5, size=3, color="black", parse=TRUE) p1 <- p1 + annotate("text", x=max(rs)*1.8, y=.55 , label=paste0("Anectodal~H[", ifelse(forH1==TRUE,1,0), "]"), hjust=1, vjust=.5, vjust=.5, size=3, color="black", parse=TRUE) # set scale ticks p1 <- p1 + scale_y_continuous(breaks=c(c(-log(c(30, 10, 3)), 0, log(c(3, 10, 30)))), labels=c("-log(30)", "-log(10)", "-log(3)", "log(1)", "log(3)", "log(10)", "log(30)")) p1 <- p1 + scale_x_continuous(breaks=seq(min(rs), max(rs), length.out=xticks)) return(p1) } else { return(res) } }
References
Rouder, J. N., Speckman, P. L., Sun, D., Morey, R. D., & Iverson, G. (2009). Bayesian t-tests for accepting and rejecting the null hypothesis. Psychonomic Bulletin and Review, 16, 225-237
#https://jimgrange.wordpress.com/2015/11/27/animating-robustness-check-of-bayes-factor-priors/ ### plots of prior robustness check # gif created from PNG plots using http://gifmaker.me/ # clear R's memory rm(list = ls()) # load the Bayes factor package library(BayesFactor) # set working directory (will be used to save files here, so make sure # this is where you want to save your plots!) setwd <- "D:/Work//Blog_YouTube code//Blog/Prior Robust Visualisation/plots" #------------------------------------------------------------------------------ ### declare some variables for the analysis # what is the t-value for the data? tVal <- 3.098 # how many points in the prior should be explored? nPoints <- 1000 # what Cauchy rates should be explored? cauchyRates <- seq(from = 0.01, to = 1.5, length.out = nPoints) # what effect sizes should be plotted? effSize <- seq(from = -2, to = 2, length.out = nPoints) # get the Bayes factor for each prior value bayesFactors <- sapply(cauchyRates, function(x) exp(ttest.tstat(t = tVal, n1 = 76, rscale = x)[['bf']])) #------------------------------------------------------------------------------ #------------------------------------------------------------------------------ ### do the plotting # how many plots do we want to produce? nPlots <- 50 plotWidth <- round(seq(from = 1, to = nPoints, length.out = nPlots), 0) # loop over each plot for(i in plotWidth){ # set up the file currFile <- paste(getwd(), "/plot_", i, ".png", sep = "") # initiate the png file png(file = currFile, width = 1200, height = 1000, res = 200) # change the plotting window so plots appear side-by-side par(mfrow = c(1, 2)) #---- # do the prior density plot d <- dcauchy(effSize, scale = cauchyRates[i]) plot(effSize, d, type = "l", ylim = c(0, 5), xlim = c(-2, 2), ylab = "Density", xlab = "Effect Size (d)", lwd = 2, col = "gray48", main = paste("Rate (r) = ", round(cauchyRates[i], 3), sep = "")) #----- # do the Bayes factor plot plot(cauchyRates, bayesFactors, type = "l", lwd = 2, col = "gray48", ylim = c(0, max(bayesFactors)), xaxt = "n", xlab = "Cauchy Prior Width (r)", ylab = "Bayes Factor (10)") abline(h = 0, lwd = 1) abline(h = 6, col = "black", lty = 2, lwd = 2) axis(1, at = seq(0, 1.5, 0.25)) # add the BF at the default Cauchy point points(0.707, 9.97, col = "black", cex = 1.5, pch = 21, bg = "red") # add the BF for the Cauchy prior currently being plotted points(cauchyRates[i], bayesFactors[i], col = "black", pch = 21, cex = 1.3, bg = "cyan") # add legend legend(x = 0.25, y = 3, legend = c("r = 0.707", paste("r = ", round(cauchyRates[i], 3), sep = "")), pch = c(21, 21), lty = c(NA, NA), lwd = c(NA, NA), pt.cex = c(1, 1), col = c("black", "black"), pt.bg = c("red", "cyan"), bty = "n") # save the current plot dev.off() } #------------------------------------------------------------------------------
skew(x, na.rm = TRUE) kurtosi(x, na.rm = TRUE) #x A data.frame or matrix #na.rm how to treat missing data ## The function is currently defined as function (x, na.rm = TRUE) { if (length(dim(x)) == 0) { if (na.rm) { x <- x[!is.na(x)] } mx <- mean(x) sdx <- sd(x,na.rm=na.rm) kurt <- sum((x - mx)^4)/(length(x) * sd(x)^4) -3 } else { kurt <- rep(NA,dim(x)[2]) mx <- colMeans(x,na.rm=na.rm) sdx <- sd(x,na.rm=na.rm) for (i in 1:dim(x)[2]) { kurt[i] <- sum((x[,i] - mx[i])^4, na.rm = na.rm)/((length(x[,i]) - sum(is.na(x[,i]))) * sdx[i]^4) -3 } } return(kurt) }
#https://richarddmorey.github.io/BayesFactor/ data(ToothGrowth) ## Example plot from ?ToothGrowth coplot(len ~ dose | supp, data = ToothGrowth, panel = panel.smooth, xlab = "ToothGrowth data: length vs dose, given type of supplement") bf = anovaBF(len ~ supp*dose, data=ToothGrowth) bf plot(bf[3:4] / bf[2]) #reduce number of comparisons (alpha inflation) bf = anovaBF(len ~ supp*dose, data=ToothGrowth, whichModels="top") bf
bfMainEffects = lmBF(len ~ supp + dose, data = ToothGrowth) bfInteraction = lmBF(len ~ supp + dose + supp:dose, data = ToothGrowth) ## Compare the two models bf = bfInteraction / bfMainEffects bf ## newbf = recompute(bf, iterations = 500000) newbf # posterior function ## Sample from the posterior of the full model chains = posterior(bfInteraction, iterations = 10000) ## 1:13 are the only "interesting" parameters summary(chains[,1:13]) #lot posterior of selected effects plot(chains[,4:6])
Comment
Reduce number of comparisons by specifyig the xact model of interest a priori.
# MODIFIED FROM BEST.R FOR ONE GROUP INSTEAD OF TWO. # Version of Dec 02, 2015. # John K. Kruschke # johnkruschke@gmail.com # http://www.indiana.edu/~kruschke/BEST/ # # This program is believed to be free of errors, but it comes with no guarantee! # The user bears all responsibility for interpreting the results. # Please check the webpage above for updates or corrections. # ### *************************************************************** ### ******** SEE FILE BEST1Gexample.R FOR INSTRUCTIONS ************ ### *************************************************************** source("openGraphSaveGraph.R") # graphics functions for Windows, Mac OS, Linux BEST1Gmcmc = function( y , numSavedSteps=100000, thinSteps=1, showMCMC=FALSE) { # This function generates an MCMC sample from the posterior distribution. # Description of arguments: # showMCMC is a flag for displaying diagnostic graphs of the chains. # If F (the default), no chain graphs are displayed. If T, they are. require(rjags) #------------------------------------------------------------------------------ # THE MODEL. modelString = " model { for ( i in 1:Ntotal ) { y[i] ~ dt( mu , tau , nu ) } mu ~ dnorm( muM , muP ) tau <- 1/pow( sigma , 2 ) sigma ~ dunif( sigmaLow , sigmaHigh ) nu ~ dexp(1/30) } " # close quote for modelString # Write out modelString to a text file writeLines( modelString , con="BESTmodel.txt" ) #------------------------------------------------------------------------------ # THE DATA. # Load the data: Ntotal = length(y) # Specify the data in a list, for later shipment to JAGS: dataList = list( y = y , Ntotal = Ntotal , muM = mean(y) , muP = 0.000001 * 1/sd(y)^2 , sigmaLow = sd(y) / 1000 , sigmaHigh = sd(y) * 1000 ) #------------------------------------------------------------------------------ # INTIALIZE THE CHAINS. # Initial values of MCMC chains based on data: mu = mean(y) sigma = sd(y) # Regarding initial values in next line: (1) sigma will tend to be too big if # the data have outliers, and (2) nu starts at 5 as a moderate value. These # initial values keep the burn-in period moderate. initsList = list( mu = mu , sigma = sigma , nu = 5 ) #------------------------------------------------------------------------------ # RUN THE CHAINS parameters = c( "mu" , "sigma" , "nu" ) # The parameters to be monitored adaptSteps = 500 # Number of steps to "tune" the samplers burnInSteps = 1000 nChains = 3 nIter = ceiling( ( numSavedSteps * thinSteps ) / nChains ) # Create, initialize, and adapt the model: jagsModel = jags.model( "BESTmodel.txt" , data=dataList , inits=initsList , n.chains=nChains , n.adapt=adaptSteps ) # Burn-in: cat( "Burning in the MCMC chain...\n" ) update( jagsModel , n.iter=burnInSteps ) # The saved MCMC chain: cat( "Sampling final MCMC chain...\n" ) codaSamples = coda.samples( jagsModel , variable.names=parameters , n.iter=nIter , thin=thinSteps ) # resulting codaSamples object has these indices: # codaSamples[[ chainIdx ]][ stepIdx , paramIdx ] #------------------------------------------------------------------------------ # EXAMINE THE RESULTS if ( showMCMC ) { openGraph(width=7,height=7) autocorr.plot( codaSamples[[1]] , ask=FALSE ) show( gelman.diag( codaSamples ) ) effectiveChainLength = effectiveSize( codaSamples ) show( effectiveChainLength ) } # Convert coda-object codaSamples to matrix object for easier handling. # But note that this concatenates the different chains into one long chain. # Result is mcmcChain[ stepIdx , paramIdx ] mcmcChain = as.matrix( codaSamples ) return( mcmcChain ) } # end function BESTmcmc #============================================================================== BEST1Gplot = function( y , mcmcChain , compValm=0.0 , ROPEm=NULL , compValsd=NULL , ROPEsd=NULL , ROPEeff=NULL , showCurve=FALSE , pairsPlot=FALSE ) { # This function plots the posterior distribution (and data). # Description of arguments: # y is the data vector. # mcmcChain is a list of the type returned by function BEST1G. # compValm is hypothesized mean for comparing with estimated mean. # ROPEm is a two element vector, such as c(-1,1), specifying the limit # of the ROPE on the mean. # ROPEsd is a two element vector, such as c(14,16), specifying the limit # of the ROPE on the difference of standard deviations. # ROPEeff is a two element vector, such as c(-1,1), specifying the limit # of the ROPE on the effect size. # showCurve is TRUE or FALSE and indicates whether the posterior should # be displayed as a histogram (by default) or by an approximate curve. # pairsPlot is TRUE or FALSE and indicates whether scatterplots of pairs # of parameters should be displayed. mu = mcmcChain[,"mu"] sigma = mcmcChain[,"sigma"] nu = mcmcChain[,"nu"] if ( pairsPlot ) { # Plot the parameters pairwise, to see correlations: openGraph(width=7,height=7) nPtToPlot = 1000 plotIdx = floor(seq(1,length(mu),by=length(mu)/nPtToPlot)) panel.cor = function(x, y, digits=2, prefix="", cex.cor, ...) { usr = par("usr"); on.exit(par(usr)) par(usr = c(0, 1, 0, 1)) r = (cor(x, y)) txt = format(c(r, 0.123456789), digits=digits)[1] txt = paste(prefix, txt, sep="") if(missing(cex.cor)) cex.cor <- 0.8/strwidth(txt) text(0.5, 0.5, txt, cex=1.25 ) # was cex=cex.cor*r } pairs( cbind( mu , sigma , log10(nu) )[plotIdx,] , labels=c( expression(mu) , expression(sigma) , expression(log10(nu)) ) , lower.panel=panel.cor , col="skyblue" ) } # Define matrix for storing summary info: summaryInfo = NULL source("plotPost.R") # Set up window and layout: openGraph(width=6.0,height=5.0) layout( matrix( c(3,3,4,4,5,5, 1,1,1,1,2,2) , nrow=6, ncol=2 , byrow=FALSE ) ) par( mar=c(3.5,3.5,2.5,0.5) , mgp=c(2.25,0.7,0) ) # Select thinned steps in chain for plotting of posterior predictive curves: chainLength = NROW( mcmcChain ) nCurvesToPlot = 30 stepIdxVec = seq( 1 , chainLength , floor(chainLength/nCurvesToPlot) ) xRange = range( y ) xLim = c( xRange[1]-0.1*(xRange[2]-xRange[1]) , xRange[2]+0.1*(xRange[2]-xRange[1]) ) xVec = seq( xLim[1] , xLim[2] , length=200 ) maxY = max( dt( 0 , df=max(nu[stepIdxVec]) ) / min(sigma[stepIdxVec]) ) # Plot data y and smattering of posterior predictive curves: stepIdx = 1 plot( xVec , dt( (xVec-mu[stepIdxVec[stepIdx]])/sigma[stepIdxVec[stepIdx]] , df=nu[stepIdxVec[stepIdx]] )/sigma[stepIdxVec[stepIdx]] , ylim=c(0,maxY) , cex.lab=1.75 , type="l" , col="skyblue" , lwd=1 , xlab="y" , ylab="p(y)" , main="Data w. Post. Pred." ) for ( stepIdx in 2:length(stepIdxVec) ) { lines(xVec, dt( (xVec-mu[stepIdxVec[stepIdx]])/sigma[stepIdxVec[stepIdx]] , df=nu[stepIdxVec[stepIdx]] )/sigma[stepIdxVec[stepIdx]] , type="l" , col="skyblue" , lwd=1 ) } histBinWd = median(sigma)/2 histCenter = mean(mu) histBreaks = sort( c( seq( histCenter-histBinWd/2 , min(xVec)-histBinWd/2 , -histBinWd ), seq( histCenter+histBinWd/2 , max(xVec)+histBinWd/2 , histBinWd ) , xLim ) ) histInfo = hist( y , plot=FALSE , breaks=histBreaks ) yPlotVec = histInfo$density yPlotVec[ yPlotVec==0.0 ] = NA xPlotVec = histInfo$mids xPlotVec[ yPlotVec==0.0 ] = NA points( xPlotVec , yPlotVec , type="h" , lwd=3 , col="red" ) text( max(xVec) , maxY , bquote(N==.(length(y))) , adj=c(1.1,1.1) ) # Plot posterior distribution of parameter nu: paramInfo = plotPost( log10(nu) , col="skyblue" , # breaks=30 , showCurve=showCurve , xlab=bquote("log10("*nu*")") , cex.lab = 1.75 , showMode=TRUE , main="Normality" ) # (<0.7 suggests kurtosis) summaryInfo = rbind( summaryInfo , paramInfo ) rownames(summaryInfo)[NROW(summaryInfo)] = "log10(nu)" # Plot posterior distribution of parameters mu1, mu2, and their difference: xlim = range( c( mu , compValm ) ) paramInfo = plotPost( mu , xlim=xlim , cex.lab = 1.75 , ROPE=ROPEm , showCurve=showCurve , compVal = compValm , xlab=bquote(mu) , main=paste("Mean") , col="skyblue" ) summaryInfo = rbind( summaryInfo , paramInfo ) rownames(summaryInfo)[NROW(summaryInfo)] = "mu" # Plot posterior distribution of sigma: xlim=range( sigma ) paramInfo = plotPost( sigma , xlim=xlim , cex.lab = 1.75 , ROPE=ROPEsd , showCurve=showCurve , compVal=compValsd , xlab=bquote(sigma) , main=paste("Std. Dev.") , col="skyblue" , showMode=TRUE ) summaryInfo = rbind( summaryInfo , paramInfo ) rownames(summaryInfo)[NROW(summaryInfo)] = "sigma" # Plot of estimated effect size: effectSize = ( mu - compValm ) / sigma paramInfo = plotPost( effectSize , compVal=0 , ROPE=ROPEeff , showCurve=showCurve , xlab=bquote( (mu-.(compValm)) / sigma ), showMode=TRUE , cex.lab=1.75 , main="Effect Size" , col="skyblue" ) summaryInfo = rbind( summaryInfo , paramInfo ) rownames(summaryInfo)[NROW(summaryInfo)] = "effSz" return( summaryInfo ) } # end of function BEST1Gplot #==============================================================================
# MODIFIED 2015 DEC 02. # John K. Kruschke # johnkruschke@gmail.com # http://www.indiana.edu/~kruschke/BEST/ # # This program is believed to be free of errors, but it comes with no guarantee! # The user bears all responsibility for interpreting the results. # Please check the webpage above for updates or corrections. # ### *************************************************************** ### ******** SEE FILE BESTexample.R FOR INSTRUCTIONS ************** ### *************************************************************** # Load various essential functions: source("DBDA2E-utilities.R") BESTmcmc = function( y1 , y2 , priorOnly=FALSE , showMCMC=FALSE , numSavedSteps=20000 , thinSteps=1 , mu1PriorMean = mean(c(y1,y2)) , mu1PriorSD = sd(c(y1,y2))*5 , mu2PriorMean = mean(c(y1,y2)) , mu2PriorSD = sd(c(y1,y2))*5 , sigma1PriorMode = sd(c(y1,y2)) , sigma1PriorSD = sd(c(y1,y2))*5 , sigma2PriorMode = sd(c(y1,y2)) , sigma2PriorSD = sd(c(y1,y2))*5 , nuPriorMean = 30 , nuPriorSD = 30 , runjagsMethod=runjagsMethodDefault , nChains=nChainsDefault ) { # This function generates an MCMC sample from the posterior distribution. # Description of arguments: # showMCMC is a flag for displaying diagnostic graphs of the chains. # If F (the default), no chain graphs are displayed. If T, they are. #------------------------------------------------------------------------------ # THE DATA. # Load the data: y = c( y1 , y2 ) # combine data into one vector x = c( rep(1,length(y1)) , rep(2,length(y2)) ) # create group membership code Ntotal = length(y) # Specify the data and prior constants in a list, for later shipment to JAGS: if ( priorOnly ) { dataList = list( # y = y , x = x , Ntotal = Ntotal , mu1PriorMean = mu1PriorMean , mu1PriorSD = mu1PriorSD , mu2PriorMean = mu2PriorMean , mu2PriorSD = mu2PriorSD , Sh1 = gammaShRaFromModeSD( mode=sigma1PriorMode , sd=sigma1PriorSD )$shape , Ra1 = gammaShRaFromModeSD( mode=sigma1PriorMode , sd=sigma1PriorSD )$rate , Sh2 = gammaShRaFromModeSD( mode=sigma2PriorMode , sd=sigma2PriorSD )$shape , Ra2 = gammaShRaFromModeSD( mode=sigma2PriorMode , sd=sigma2PriorSD )$rate , ShNu = gammaShRaFromMeanSD( mean=nuPriorMean , sd=nuPriorSD )$shape , RaNu = gammaShRaFromMeanSD( mean=nuPriorMean , sd=nuPriorSD )$rate ) } else { dataList = list( y = y , x = x , Ntotal = Ntotal , mu1PriorMean = mu1PriorMean , mu1PriorSD = mu1PriorSD , mu2PriorMean = mu2PriorMean , mu2PriorSD = mu2PriorSD , Sh1 = gammaShRaFromModeSD( mode=sigma1PriorMode , sd=sigma1PriorSD )$shape , Ra1 = gammaShRaFromModeSD( mode=sigma1PriorMode , sd=sigma1PriorSD )$rate , Sh2 = gammaShRaFromModeSD( mode=sigma2PriorMode , sd=sigma2PriorSD )$shape , Ra2 = gammaShRaFromModeSD( mode=sigma2PriorMode , sd=sigma2PriorSD )$rate , ShNu = gammaShRaFromMeanSD( mean=nuPriorMean , sd=nuPriorSD )$shape , RaNu = gammaShRaFromMeanSD( mean=nuPriorMean , sd=nuPriorSD )$rate ) } #---------------------------------------------------------------------------- # THE MODEL. modelString = " model { for ( i in 1:Ntotal ) { y[i] ~ dt( mu[x[i]] , 1/sigma[x[i]]^2 , nu ) } mu[1] ~ dnorm( mu1PriorMean , 1/mu1PriorSD^2 ) # prior for mu[1] sigma[1] ~ dgamma( Sh1 , Ra1 ) # prior for sigma[1] mu[2] ~ dnorm( mu2PriorMean , 1/mu2PriorSD^2 ) # prior for mu[2] sigma[2] ~ dgamma( Sh2 , Ra2 ) # prior for sigma[2] nu ~ dgamma( ShNu , RaNu ) # prior for nu } " # close quote for modelString # Write out modelString to a text file writeLines( modelString , con="BESTmodel.txt" ) #------------------------------------------------------------------------------ # INTIALIZE THE CHAINS. # Initial values of MCMC chains based on data: mu = c( mean(y1) , mean(y2) ) sigma = c( sd(y1) , sd(y2) ) # Regarding initial values in next line: (1) sigma will tend to be too big if # the data have outliers, and (2) nu starts at 5 as a moderate value. These # initial values keep the burn-in period moderate. initsList = list( mu = mu , sigma = sigma , nu = 5 ) #------------------------------------------------------------------------------ # RUN THE CHAINS parameters = c( "mu" , "sigma" , "nu" ) # The parameters to be monitored adaptSteps = 500 # Number of steps to "tune" the samplers burnInSteps = 1000 runJagsOut <- run.jags( method=runjagsMethod , model="BESTmodel.txt" , monitor=parameters , data=dataList , inits=initsList , n.chains=nChains , adapt=adaptSteps , burnin=burnInSteps , sample=ceiling(numSavedSteps/nChains) , thin=thinSteps , summarise=FALSE , plots=FALSE ) codaSamples = as.mcmc.list( runJagsOut ) # resulting codaSamples object has these indices: # codaSamples[[ chainIdx ]][ stepIdx , paramIdx ] ## Original version, using rjags: # nChains = 3 # nIter = ceiling( ( numSavedSteps * thinSteps ) / nChains ) # # Create, initialize, and adapt the model: # jagsModel = jags.model( "BESTmodel.txt" , data=dataList , inits=initsList , # n.chains=nChains , n.adapt=adaptSteps ) # # Burn-in: # cat( "Burning in the MCMC chain...\n" ) # update( jagsModel , n.iter=burnInSteps ) # # The saved MCMC chain: # cat( "Sampling final MCMC chain...\n" ) # codaSamples = coda.samples( jagsModel , variable.names=parameters , # n.iter=nIter , thin=thinSteps ) #------------------------------------------------------------------------------ # EXAMINE THE RESULTS if ( showMCMC ) { for ( paramName in varnames(codaSamples) ) { diagMCMC( codaSamples , parName=paramName ) } } # Convert coda-object codaSamples to matrix object for easier handling. # But note that this concatenates the different chains into one long chain. # Result is mcmcChain[ stepIdx , paramIdx ] mcmcChain = as.matrix( codaSamples ) return( mcmcChain ) } # end function BESTmcmc #============================================================================== BESTsummary = function( y1 , y2 , mcmcChain ) { #source("HDIofMCMC.R") # in DBDA2E-utilities.R mcmcSummary = function( paramSampleVec , compVal=NULL ) { meanParam = mean( paramSampleVec ) medianParam = median( paramSampleVec ) dres = density( paramSampleVec ) modeParam = dres$x[which.max(dres$y)] hdiLim = HDIofMCMC( paramSampleVec ) if ( !is.null(compVal) ) { pcgtCompVal = ( 100 * sum( paramSampleVec > compVal ) / length( paramSampleVec ) ) } else { pcgtCompVal=NA } return( c( meanParam , medianParam , modeParam , hdiLim , pcgtCompVal ) ) } # Define matrix for storing summary info: summaryInfo = matrix( 0 , nrow=9 , ncol=6 , dimnames=list( PARAMETER=c( "mu1" , "mu2" , "muDiff" , "sigma1" , "sigma2" , "sigmaDiff" , "nu" , "nuLog10" , "effSz" ), SUMMARY.INFO=c( "mean" , "median" , "mode" , "HDIlow" , "HDIhigh" , "pcgtZero" ) ) ) summaryInfo[ "mu1" , ] = mcmcSummary( mcmcChain[,"mu[1]"] ) summaryInfo[ "mu2" , ] = mcmcSummary( mcmcChain[,"mu[2]"] ) summaryInfo[ "muDiff" , ] = mcmcSummary( mcmcChain[,"mu[1]"] - mcmcChain[,"mu[2]"] , compVal=0 ) summaryInfo[ "sigma1" , ] = mcmcSummary( mcmcChain[,"sigma[1]"] ) summaryInfo[ "sigma2" , ] = mcmcSummary( mcmcChain[,"sigma[2]"] ) summaryInfo[ "sigmaDiff" , ] = mcmcSummary( mcmcChain[,"sigma[1]"] - mcmcChain[,"sigma[2]"] , compVal=0 ) summaryInfo[ "nu" , ] = mcmcSummary( mcmcChain[,"nu"] ) summaryInfo[ "nuLog10" , ] = mcmcSummary( log10(mcmcChain[,"nu"]) ) N1 = length(y1) N2 = length(y2) effSzChain = ( ( mcmcChain[,"mu[1]"] - mcmcChain[,"mu[2]"] ) / sqrt( ( mcmcChain[,"sigma[1]"]^2 + mcmcChain[,"sigma[2]"]^2 ) / 2 ) ) summaryInfo[ "effSz" , ] = mcmcSummary( effSzChain , compVal=0 ) # Or, use sample-size weighted version: # effSz = ( mu1 - mu2 ) / sqrt( ( sigma1^2 *(N1-1) + sigma2^2 *(N2-1) ) # / (N1+N2-2) ) # Be sure also to change plot label in BESTplot function, below. return( summaryInfo ) } #============================================================================== BESTplot = function( y1 , y2 , mcmcChain , ROPEm=NULL , ROPEsd=NULL , ROPEeff=NULL , showCurve=FALSE , pairsPlot=FALSE ) { # This function plots the posterior distribution (and data). # Description of arguments: # y1 and y2 are the data vectors. # mcmcChain is a list of the type returned by function BTT. # ROPEm is a two element vector, such as c(-1,1), specifying the limit # of the ROPE on the difference of means. # ROPEsd is a two element vector, such as c(-1,1), specifying the limit # of the ROPE on the difference of standard deviations. # ROPEeff is a two element vector, such as c(-1,1), specifying the limit # of the ROPE on the effect size. # showCurve is TRUE or FALSE and indicates whether the posterior should # be displayed as a histogram (by default) or by an approximate curve. # pairsPlot is TRUE or FALSE and indicates whether scatterplots of pairs # of parameters should be displayed. mu1 = mcmcChain[,"mu[1]"] mu2 = mcmcChain[,"mu[2]"] sigma1 = mcmcChain[,"sigma[1]"] sigma2 = mcmcChain[,"sigma[2]"] nu = mcmcChain[,"nu"] if ( pairsPlot ) { # Plot the parameters pairwise, to see correlations: openGraph(width=7,height=7) nPtToPlot = 1000 plotIdx = floor(seq(1,length(mu1),by=length(mu1)/nPtToPlot)) panel.cor = function(x, y, digits=2, prefix="", cex.cor, ...) { usr = par("usr"); on.exit(par(usr)) par(usr = c(0, 1, 0, 1)) r = (cor(x, y)) txt = format(c(r, 0.123456789), digits=digits)[1] txt = paste(prefix, txt, sep="") if(missing(cex.cor)) cex.cor <- 0.8/strwidth(txt) text(0.5, 0.5, txt, cex=1.25 ) # was cex=cex.cor*r } pairs( cbind( mu1 , mu2 , sigma1 , sigma2 , log10(nu) )[plotIdx,] , labels=c( expression(mu[1]) , expression(mu[2]) , expression(sigma[1]) , expression(sigma[2]) , expression(log10(nu)) ) , lower.panel=panel.cor , col="skyblue" ) } # source("plotPost.R") # in DBDA2E-utilities.R # Set up window and layout: openGraph(width=6.0,height=8.0) layout( matrix( c(4,5,7,8,3,1,2,6,9,10) , nrow=5, byrow=FALSE ) ) par( mar=c(3.5,3.5,2.5,0.5) , mgp=c(2.25,0.7,0) ) # Select thinned steps in chain for plotting of posterior predictive curves: chainLength = NROW( mcmcChain ) nCurvesToPlot = 30 stepIdxVec = seq( 1 , chainLength , floor(chainLength/nCurvesToPlot) ) xRange = range( c(y1,y2) ) if ( isTRUE( all.equal( xRange[2] , xRange[1] ) ) ) { meanSigma = mean( c(sigma1,sigma2) ) xRange = xRange + c( -meanSigma , meanSigma ) } xLim = c( xRange[1]-0.1*(xRange[2]-xRange[1]) , xRange[2]+0.1*(xRange[2]-xRange[1]) ) xVec = seq( xLim[1] , xLim[2] , length=200 ) maxY = max( dt( 0 , df=max(nu[stepIdxVec]) ) / min(c(sigma1[stepIdxVec],sigma2[stepIdxVec])) ) # Plot data y1 and smattering of posterior predictive curves: stepIdx = 1 plot( xVec , dt( (xVec-mu1[stepIdxVec[stepIdx]])/sigma1[stepIdxVec[stepIdx]] , df=nu[stepIdxVec[stepIdx]] )/sigma1[stepIdxVec[stepIdx]] , ylim=c(0,maxY) , cex.lab=1.75 , type="l" , col="skyblue" , lwd=1 , xlab="y" , ylab="p(y)" , main="Data Group 1 w. Post. Pred." ) for ( stepIdx in 2:length(stepIdxVec) ) { lines(xVec, dt( (xVec-mu1[stepIdxVec[stepIdx]])/sigma1[stepIdxVec[stepIdx]] , df=nu[stepIdxVec[stepIdx]] )/sigma1[stepIdxVec[stepIdx]] , type="l" , col="skyblue" , lwd=1 ) } histBinWd = median(sigma1)/2 histCenter = mean(mu1) histBreaks = sort( c( seq( histCenter-histBinWd/2 , min(xVec)-histBinWd/2 , -histBinWd ), seq( histCenter+histBinWd/2 , max(xVec)+histBinWd/2 , histBinWd ) , xLim ) ) histInfo = hist( y1 , plot=FALSE , breaks=histBreaks ) yPlotVec = histInfo$density yPlotVec[ yPlotVec==0.0 ] = NA xPlotVec = histInfo$mids xPlotVec[ yPlotVec==0.0 ] = NA points( xPlotVec , yPlotVec , type="h" , lwd=3 , col="red" ) text( max(xVec) , maxY , bquote(N[1]==.(length(y1))) , adj=c(1.1,1.1) ) # Plot data y2 and smattering of posterior predictive curves: stepIdx = 1 plot( xVec , dt( (xVec-mu2[stepIdxVec[stepIdx]])/sigma2[stepIdxVec[stepIdx]] , df=nu[stepIdxVec[stepIdx]] )/sigma2[stepIdxVec[stepIdx]] , ylim=c(0,maxY) , cex.lab=1.75 , type="l" , col="skyblue" , lwd=1 , xlab="y" , ylab="p(y)" , main="Data Group 2 w. Post. Pred." ) for ( stepIdx in 2:length(stepIdxVec) ) { lines(xVec, dt( (xVec-mu2[stepIdxVec[stepIdx]])/sigma2[stepIdxVec[stepIdx]] , df=nu[stepIdxVec[stepIdx]] )/sigma2[stepIdxVec[stepIdx]] , type="l" , col="skyblue" , lwd=1 ) } histBinWd = median(sigma2)/2 histCenter = mean(mu2) histBreaks = sort( c( seq( histCenter-histBinWd/2 , min(xVec)-histBinWd/2 , -histBinWd ), seq( histCenter+histBinWd/2 , max(xVec)+histBinWd/2 , histBinWd ) , xLim ) ) histInfo = hist( y2 , plot=FALSE , breaks=histBreaks ) yPlotVec = histInfo$density yPlotVec[ yPlotVec==0.0 ] = NA xPlotVec = histInfo$mids xPlotVec[ yPlotVec==0.0 ] = NA points( xPlotVec , yPlotVec , type="h" , lwd=3 , col="red" ) text( max(xVec) , maxY , bquote(N[2]==.(length(y2))) , adj=c(1.1,1.1) ) # Plot posterior distribution of parameter nu: histInfo = plotPost( log10(nu) , col="skyblue" , # breaks=30 , showCurve=showCurve , xlab=bquote("log10("*nu*")") , cex.lab = 1.75 , cenTend=c("mode","median","mean")[1] , main="Normality" ) # (<0.7 suggests kurtosis) # Plot posterior distribution of parameters mu1, mu2, and their difference: xlim = range( c( mu1 , mu2 ) ) histInfo = plotPost( mu1 , xlim=xlim , cex.lab = 1.75 , showCurve=showCurve , xlab=bquote(mu[1]) , main=paste("Group",1,"Mean") , col="skyblue" ) histInfo = plotPost( mu2 , xlim=xlim , cex.lab = 1.75 , showCurve=showCurve , xlab=bquote(mu[2]) , main=paste("Group",2,"Mean") , col="skyblue" ) histInfo = plotPost( mu1-mu2 , compVal=0 , showCurve=showCurve , xlab=bquote(mu[1] - mu[2]) , cex.lab = 1.75 , ROPE=ROPEm , main="Difference of Means" , col="skyblue" ) # Plot posterior distribution of param's sigma1, sigma2, and their difference: xlim=range( c( sigma1 , sigma2 ) ) histInfo = plotPost( sigma1 , xlim=xlim , cex.lab = 1.75 , showCurve=showCurve , xlab=bquote(sigma[1]) , main=paste("Group",1,"Std. Dev.") , col="skyblue" , cenTend=c("mode","median","mean")[1] ) histInfo = plotPost( sigma2 , xlim=xlim , cex.lab = 1.75 , showCurve=showCurve , xlab=bquote(sigma[2]) , main=paste("Group",2,"Std. Dev.") , col="skyblue" , cenTend=c("mode","median","mean")[1] ) histInfo = plotPost( sigma1-sigma2 , compVal=0 , showCurve=showCurve , xlab=bquote(sigma[1] - sigma[2]) , cex.lab = 1.75 , ROPE=ROPEsd , main="Difference of Std. Dev.s" , col="skyblue" , cenTend=c("mode","median","mean")[1] ) # Plot of estimated effect size. Effect size is d-sub-a from # Macmillan & Creelman, 1991; Simpson & Fitter, 1973; Swets, 1986a, 1986b. effectSize = ( mu1 - mu2 ) / sqrt( ( sigma1^2 + sigma2^2 ) / 2 ) histInfo = plotPost( effectSize , compVal=0 , ROPE=ROPEeff , showCurve=showCurve , xlab=bquote( (mu[1]-mu[2]) /sqrt((sigma[1]^2 +sigma[2]^2 )/2 ) ), cenTend=c("mode","median","mean")[1] , cex.lab=1.0 , main="Effect Size" , col="skyblue" ) # Or use sample-size weighted version: # Hedges 1981; Wetzels, Raaijmakers, Jakab & Wagenmakers 2009. # N1 = length(y1) # N2 = length(y2) # effectSize = ( mu1 - mu2 ) / sqrt( ( sigma1^2 *(N1-1) + sigma2^2 *(N2-1) ) # / (N1+N2-2) ) # Be sure also to change BESTsummary function, above. # histInfo = plotPost( effectSize , compVal=0 , ROPE=ROPEeff , # showCurve=showCurve , # xlab=bquote( (mu[1]-mu[2]) # /sqrt((sigma[1]^2 *(N[1]-1)+sigma[2]^2 *(N[2]-1))/(N[1]+N[2]-2)) ), # cenTend=c("mode","median","mean")[1] , cex.lab=1.0 , main="Effect Size" , col="skyblue" ) return( BESTsummary( y1 , y2 , mcmcChain ) ) } # end of function BESTplot #============================================================================== BESTpower = function( mcmcChain , N1 , N2 , ROPEm , ROPEsd , ROPEeff , maxHDIWm , maxHDIWsd , maxHDIWeff , nRep=200 , mcmcLength=10000 , saveName="BESTpower.Rdata" , showFirstNrep=0 ) { # This function estimates power. # Description of arguments: # mcmcChain is a matrix of the type returned by function BESTmcmc. # N1 and N2 are sample sizes for the two groups. # ROPEm is a two element vector, such as c(-1,1), specifying the limit # of the ROPE on the difference of means. # ROPEsd is a two element vector, such as c(-1,1), specifying the limit # of the ROPE on the difference of standard deviations. # ROPEeff is a two element vector, such as c(-1,1), specifying the limit # of the ROPE on the effect size. # maxHDIWm is the maximum desired width of the 95% HDI on the difference # of means. # maxHDIWsd is the maximum desired width of the 95% HDI on the difference # of standard deviations. # maxHDIWeff is the maximum desired width of the 95% HDI on the effect size. # nRep is the number of simulated experiments used to estimate the power. #source("HDIofMCMC.R") # in DBDA2E-utilities.R #source("HDIofICDF.R") # in DBDA2E-utilities.R chainLength = NROW( mcmcChain ) # Select thinned steps in chain for posterior predictions: stepIdxVec = seq( 1 , chainLength , floor(chainLength/nRep) ) goalTally = list( HDIm.gt.ROPE = c(0) , HDIm.lt.ROPE = c(0) , HDIm.in.ROPE = c(0) , HDIm.wd.max = c(0) , HDIsd.gt.ROPE = c(0) , HDIsd.lt.ROPE = c(0) , HDIsd.in.ROPE = c(0) , HDIsd.wd.max = c(0) , HDIeff.gt.ROPE = c(0) , HDIeff.lt.ROPE = c(0) , HDIeff.in.ROPE = c(0) , HDIeff.wd.max = c(0) ) power = list( HDIm.gt.ROPE = c(0,0,0) , HDIm.lt.ROPE = c(0,0,0) , HDIm.in.ROPE = c(0,0,0) , HDIm.wd.max = c(0,0,0) , HDIsd.gt.ROPE = c(0,0,0) , HDIsd.lt.ROPE = c(0,0,0) , HDIsd.in.ROPE = c(0,0,0) , HDIsd.wd.max = c(0,0,0) , HDIeff.gt.ROPE = c(0,0,0) , HDIeff.lt.ROPE = c(0,0,0) , HDIeff.in.ROPE = c(0,0,0) , HDIeff.wd.max = c(0,0,0) ) nSim = 0 for ( stepIdx in stepIdxVec ) { nSim = nSim + 1 cat( "\n:::::::::::::::::::::::::::::::::::::::::::::::::::::::::::::::\n" ) cat( paste( "Power computation: Simulated Experiment" , nSim , "of" , length(stepIdxVec) , ":\n\n" ) ) # Get parameter values for this simulation: mu1Val = mcmcChain[stepIdx,"mu[1]"] mu2Val = mcmcChain[stepIdx,"mu[2]"] sigma1Val = mcmcChain[stepIdx,"sigma[1]"] sigma2Val = mcmcChain[stepIdx,"sigma[2]"] nuVal = mcmcChain[stepIdx,"nu"] # Generate simulated data: y1 = rt( N1 , df=nuVal ) * sigma1Val + mu1Val y2 = rt( N2 , df=nuVal ) * sigma2Val + mu2Val # Get posterior for simulated data: simChain = BESTmcmc( y1, y2, numSavedSteps=mcmcLength, thinSteps=1, showMCMC=FALSE ) if (nSim <= showFirstNrep ) { BESTplot( y1, y2, simChain, ROPEm=ROPEm, ROPEsd=ROPEsd, ROPEeff=ROPEeff ) } # Assess which goals were achieved and tally them: HDIm = HDIofMCMC( simChain[,"mu[1]"] - simChain[,"mu[2]"] ) if ( HDIm[1] > ROPEm[2] ) { goalTally$HDIm.gt.ROPE = goalTally$HDIm.gt.ROPE + 1 } if ( HDIm[2] < ROPEm[1] ) { goalTally$HDIm.lt.ROPE = goalTally$HDIm.lt.ROPE + 1 } if ( HDIm[1] > ROPEm[1] & HDIm[2] < ROPEm[2] ) { goalTally$HDIm.in.ROPE = goalTally$HDIm.in.ROPE + 1 } if ( HDIm[2] - HDIm[1] < maxHDIWm ) { goalTally$HDIm.wd.max = goalTally$HDIm.wd.max + 1 } HDIsd = HDIofMCMC( simChain[,"sigma[1]"] - simChain[,"sigma[2]"] ) if ( HDIsd[1] > ROPEsd[2] ) { goalTally$HDIsd.gt.ROPE = goalTally$HDIsd.gt.ROPE + 1 } if ( HDIsd[2] < ROPEsd[1] ) { goalTally$HDIsd.lt.ROPE = goalTally$HDIsd.lt.ROPE + 1 } if ( HDIsd[1] > ROPEsd[1] & HDIsd[2] < ROPEsd[2] ) { goalTally$HDIsd.in.ROPE = goalTally$HDIsd.in.ROPE + 1 } if ( HDIsd[2] - HDIsd[1] < maxHDIWsd ) { goalTally$HDIsd.wd.max = goalTally$HDIsd.wd.max + 1 } HDIeff = HDIofMCMC( ( simChain[,"mu[1]"] - simChain[,"mu[2]"] ) / sqrt( ( simChain[,"sigma[1]"]^2 + simChain[,"sigma[2]"]^2 ) / 2 ) ) if ( HDIeff[1] > ROPEeff[2] ) { goalTally$HDIeff.gt.ROPE = goalTally$HDIeff.gt.ROPE + 1 } if ( HDIeff[2] < ROPEeff[1] ) { goalTally$HDIeff.lt.ROPE = goalTally$HDIeff.lt.ROPE + 1 } if ( HDIeff[1] > ROPEeff[1] & HDIeff[2] < ROPEeff[2] ) { goalTally$HDIeff.in.ROPE = goalTally$HDIeff.in.ROPE + 1 } if ( HDIeff[2] - HDIeff[1] < maxHDIWeff ) { goalTally$HDIeff.wd.max = goalTally$HDIeff.wd.max + 1 } for ( i in 1:length(goalTally) ) { s1 = 1 + goalTally[[i]] s2 = 1 + ( nSim - goalTally[[i]] ) power[[i]][1] = s1/(s1+s2) power[[i]][2:3] = HDIofICDF( qbeta , shape1=s1 , shape2=s2 ) } cat( "\nAfter ", nSim , " Simulated Experiments, Power Is: \n( mean, HDI_low, HDI_high )\n" ) for ( i in 1:length(power) ) { cat( names(power[i]) , round(power[[i]],3) , "\n" ) } save( nSim , power , file=saveName ) } return( power ) } # end of function BESTpower #============================================================================== makeData = function( mu1 , sd1 , mu2 , sd2 , nPerGrp , pcntOut=0 , sdOutMult=2.0 , rnd.seed=NULL ) { # Auxilliary function for generating random values from a # mixture of normal distibutions. if(!is.null(rnd.seed)){set.seed(rnd.seed)} # Set seed for random values. nOut = ceiling(nPerGrp*pcntOut/100) # Number of outliers. nIn = nPerGrp - nOut # Number from main distribution. if ( pcntOut > 100 | pcntOut < 0 ) { stop("pcntOut must be between 0 and 100.") } if ( pcntOut > 0 & pcntOut < 1 ) { warning("pcntOut is specified as percentage 0-100, not proportion 0-1.") } if ( pcntOut > 50 ) { warning("pcntOut indicates more than 50% outliers; did you intend this?") } if ( nOut < 2 & pcntOut > 0 ) { stop("Combination of nPerGrp and pcntOut yields too few outliers.") } if ( nIn < 2 ) { stop("Too few non-outliers.") } sdN = function( x ) { sqrt( mean( (x-mean(x))^2 ) ) } # genDat = function( mu , sigma , Nin , Nout , sigmaOutMult=sdOutMult ) { y = rnorm(n=Nin) # Random values in main distribution. y = ((y-mean(y))/sdN(y))*sigma + mu # Translate to exactly realize desired. yOut = NULL if ( Nout > 0 ) { yOut = rnorm(n=Nout) # Random values for outliers yOut=((yOut-mean(yOut))/sdN(yOut))*(sigma*sdOutMult)+mu # Realize exactly. } y = c(y,yOut) # Concatenate main with outliers. return(y) } y1 = genDat( mu=mu1 , sigma=sd1 , Nin=nIn , Nout=nOut ) y2 = genDat( mu=mu2 , sigma=sd2 , Nin=nIn , Nout=nOut ) # # Set up window and layout: openGraph(width=7,height=7) # Plot the data. layout(matrix(1:2,nrow=2)) histInfo = hist( y1 , main="Simulated Data" , col="pink2" , border="white" , xlim=range(c(y1,y2)) , breaks=30 , prob=TRUE ) text( max(c(y1,y2)) , max(histInfo$density) , bquote(N==.(nPerGrp)) , adj=c(1,1) ) xlow=min(histInfo$breaks) xhi=max(histInfo$breaks) xcomb=seq(xlow,xhi,length=1001) lines( xcomb , dnorm(xcomb,mean=mu1,sd=sd1)*nIn/(nIn+nOut) + dnorm(xcomb,mean=mu1,sd=sd1*sdOutMult)*nOut/(nIn+nOut) , lwd=3 ) lines( xcomb , dnorm(xcomb,mean=mu1,sd=sd1)*nIn/(nIn+nOut) , lty="dashed" , col="green", lwd=3) lines( xcomb , dnorm(xcomb,mean=mu1,sd=sd1*sdOutMult)*nOut/(nIn+nOut) , lty="dashed" , col="red", lwd=3) histInfo = hist( y2 , main="" , col="pink2" , border="white" , xlim=range(c(y1,y2)) , breaks=30 , prob=TRUE ) text( max(c(y1,y2)) , max(histInfo$density) , bquote(N==.(nPerGrp)) , adj=c(1,1) ) xlow=min(histInfo$breaks) xhi=max(histInfo$breaks) xcomb=seq(xlow,xhi,length=1001) lines( xcomb , dnorm(xcomb,mean=mu2,sd=sd2)*nIn/(nIn+nOut) + dnorm(xcomb,mean=mu2,sd=sd2*sdOutMult)*nOut/(nIn+nOut) , lwd=3) lines( xcomb , dnorm(xcomb,mean=mu2,sd=sd2)*nIn/(nIn+nOut) , lty="dashed" , col="green", lwd=3) lines( xcomb , dnorm(xcomb,mean=mu2,sd=sd2*sdOutMult)*nOut/(nIn+nOut) , lty="dashed" , col="red", lwd=3) # return( list( y1=y1 , y2=y2 ) ) } #==============================================================================
# Version of May 26, 2012. Re-checked on 2015 May 08. # John K. Kruschke # johnkruschke@gmail.com # http://www.indiana.edu/~kruschke/BEST/ # # This program is believed to be free of errors, but it comes with no guarantee! # The user bears all responsibility for interpreting the results. # Please check the webpage above for updates or corrections. # ### *************************************************************** ### ******** SEE FILE BESTexample.R FOR INSTRUCTIONS ************** ### *************************************************************** # OPTIONAL: Clear R's memory and graphics: rm(list=ls()) # Careful! This clears all of R's memory! graphics.off() # This closes all of R's graphics windows. # Get the functions loaded into R's working memory: source("BEST.R") #------------------------------------------------------------------------------- # RETROSPECTIVE POWER ANALYSIS. # !! This section assumes you have already run BESTexample.R !! # Re-load the saved data and MCMC chain from the previously conducted # Bayesian analysis. This re-loads the variables y1, y2, mcmcChain, etc. load( "BESTexampleMCMC.Rdata" ) power = BESTpower( mcmcChain , N1=length(y1) , N2=length(y2) , ROPEm=c(-0.1,0.1) , ROPEsd=c(-0.1,0.1) , ROPEeff=c(-0.1,0.1) , maxHDIWm=2.0 , maxHDIWsd=2.0 , maxHDIWeff=0.2 , nRep=1000 , mcmcLength=10000 , saveName = "BESTexampleRetroPower.Rdata" ) #------------------------------------------------------------------------------- # PROSPECTIVE POWER ANALYSIS, using fictitious strong data. # Generate large fictitious data set that expresses hypothesis: prospectData = makeData( mu1=108, sd1=17, mu2=100, sd2=15, nPerGrp=1000, pcntOut=10, sdOutMult=2.0, rnd.seed=NULL ) y1pro = prospectData$y1 # Merely renames simulated data for convenience below. y2pro = prospectData$y2 # Merely renames simulated data for convenience below. # Generate Bayesian posterior distribution from fictitious data: # (uses fewer than usual MCMC steps because it only needs nRep credible # parameter combinations, not a high-resolution representation) mcmcChainPro = BESTmcmc( y1pro , y2pro , numSavedSteps=2000 ) postInfoPro = BESTplot( y1pro , y2pro , mcmcChainPro , pairsPlot=TRUE ) save( y1pro, y2pro, mcmcChainPro, postInfoPro, file="BESTexampleProPowerMCMC.Rdata" ) # Now compute the prospective power for planned sample sizes: N1plan = N2plan = 50 # specify planned sample size powerPro = BESTpower( mcmcChainPro , N1=N1plan , N2=N2plan , showFirstNrep=5 , ROPEm=c(-1.5,1.5) , ROPEsd=c(-0.0,0.0) , ROPEeff=c(-0.0,0.0) , maxHDIWm=15.0 , maxHDIWsd=10.0 , maxHDIWeff=1.0 , nRep=1000 , mcmcLength=10000 , saveName = "BESTexampleProPower.Rdata" ) #-------------------------------------------------------------------------------
#https://cran.r-project.org/web/packages/beanplot/beanplot.pdf par( mfrow = c( 1, 4 ) ) with(dataexp2, beanplot(v00, ylim = c(0,10), col="lightgray", main = "v00", kernel = "gaussian", cut = 3, cutmin = -Inf, cutmax = Inf, overallline = "mean", horizontal = FALSE, side = "no", jitter = NULL, beanlinewd = 2)) with(dataexp2, beanplot(v01, ylim = c(0,10), col="lightgray", main = "v01", kernel = "gaussian", cut = 3, cutmin = -Inf, cutmax = Inf, overallline = "mean", horizontal = FALSE, side = "no", jitter = NULL, beanlinewd = 2)) with(dataexp2, beanplot(v10, ylim = c(0,10), col="darkgray", main = "v10", kernel = "gaussian", cut = 3, cutmin = -Inf, cutmax = Inf, overallline = "mean", horizontal = FALSE, side = "no", jitter = NULL, beanlinewd = 2)) with(dataexp2, beanplot(v11, ylim = c(0,10), col="darkgray", main = "v11", kernel = "gaussian", cut = 3, cutmin = -Inf, cutmax = Inf, overallline = "mean", horizontal = FALSE, side = "no", jitter = NULL, beanlinewd = 2)) #with ylim and titles with(dataexp2, beanplot(v00, ylab = "VAS Brightness rating", xlab = "beanplot showing distribution and mean", ylim = c(0,10), col="darkgray", main = "v00", kernel = "gaussian", cut = 3, cutmin = -Inf, cutmax = Inf, overallline = "mean", horizontal = FALSE, side = "no", jitter = NULL, beanlinewd = 2)) install.packages ("beanplot") library("beanplot") par( mfrow = c( 1, 2 ) ) with(dataexp2, beanplot(v00, col="darkgray", main = "v00", kernel = "gaussian", cut = 3, cutmin = -Inf, cutmax = Inf, overallline = "mean", horizontal = FALSE, side = "no", jitter = NULL, beanlinewd = 2)) with(dataexp2, beanplot(v01, col="darkgray", main = "v01", kernel = "gaussian", cut = 3, cutmin = -Inf, cutmax = Inf, overallline = "mean", horizontal = FALSE, side = "no", jitter = NULL, beanlinewd = 2)) par( mfrow = c( 1, 2 ) ) with(dataexp2, beanplot(v10, col="darkgray", main = "v10", kernel = "gaussian", cut = 3, cutmin = -Inf, cutmax = Inf, overallline = "mean", horizontal = FALSE, side = "no", jitter = NULL, beanlinewd = 2)) with(dataexp2, beanplot(v11, col="darkgray", main = "v11", kernel = "gaussian", cut = 3, cutmin = -Inf, cutmax = Inf, overallline = "mean", horizontal = FALSE, side = "no", jitter = NULL, beanlinewd = 2)) stripchart(variable ~ factor, vertical=TRUE, method="stack", ylab="variable", data=StackedData) #two sided with(dataexp1, beanplot(v00, v10, col="darkgray", main = "v00 vs. v10", kernel = "gaussian", cut = 3, cutmin = -Inf, cutmax = Inf, overallline = "mean", horizontal = FALSE, side = "both", jitter = NULL, beanlinewd = 2)) #verticalpar( mfrow = c( 2, 1 ) ) install.packages ("beanplot") library("beanplot") par( mfrow = c( 2, 1 ) ) # layout matrix with(dataexp1, beanplot(v00, v10, main = "v00 vs. v10", kernel = "gaussian", cut = 3, col = list("black", c("grey", "white")), xlab="VAS", cutmin = -Inf, cutmax = Inf, overallline = "mean", horizontal = T, side = "both", jitter = NULL, beanlinewd = 2)) with(dataexp1, beanplot(v01, v11, main = "v01 vs. v11", kernel = "gaussian", cut = 3, col = list("black", c("grey", "white")), xlab="VAS", cutmin = -Inf, cutmax = Inf, overallline = "mean", horizontal = T, side = "both", jitter = NULL, beanlinewd = 2)) #exp1 dataexp1 <- read.table("http://www.irrational-decisions.com/phd-thesis/dataexp1.csv", header=TRUE, sep=",", na.strings="NA", dec=".", strip.white=TRUE) par( mfrow = c( 1, 2) ) with(dataexp1, beanplot(v00, ylim = c(0,7), col="lightgray", main = "v00", kernel = "gaussian", cut = 3, cutmin = -Inf, cutmax = Inf, overallline = "mean", horizontal = FALSE, side = "no", jitter = NULL, beanlinewd = 2)) with(dataexp1, beanplot(v10, ylim = c(0,7), col="lightgray", main = "v01", kernel = "gaussian", cut = 3, cutmin = -Inf, cutmax = Inf, overallline = "mean", horizontal = FALSE, side = "no", jitter = NULL, beanlinewd = 2)) par( mfrow = c( 1, 2) ) with(dataexp1, beanplot(v01, ylim = c(3,10), col="darkgray", main = "v10", kernel = "gaussian", cut = 3, cutmin = -Inf, cutmax = Inf, overallline = "mean", horizontal = FALSE, side = "no", jitter = NULL, beanlinewd = 2)) with(dataexp1, beanplot(v11, ylim = c(3,10), col="darkgray", main = "v11", kernel = "gaussian", cut = 3, cutmin = -Inf, cutmax = Inf, overallline = "mean", horizontal = FALSE, side = "no", jitter = NULL, beanlinewd = 2))
#histogram and rug plot plot(density(dataexp2$v00)) rug(dataexp2$v00, col=2, lwd=3.5) ############## library(MASS) plot(density(dataexp2$v00, bw=5)) rug(dataexp2$v00+ rnorm(length(dataexp2$v00), sd=5), col=2, lwd=3.5)
x = c(5) y = c(9) plot(x,y,type="h",xlim=c(0,10),ylim=c(0,10),lwd=2,col="blue",ylab="knowledge", xlab="diversity")
install.packages("gtrendsR") library(gtrendsR) gtrends(c("bayesian inference", "p-value"), time = "all") # since 2004 res <- gtrends(c("Bayesian inference", "Markov Chain Monte Carlo", "ANOVA"), geo = c("US", "GB", "DE", "CA",)) res <- gtrends("Markov Chain Monte Carlo", geo = c("US", "GB", "DE")) plot(res) #get vars length(res) str(res) res$interest_over_time ff<-res$interest_over_time scatterplot(ff$hits~ff$date| geo, reg.line=FALSE, smooth=FALSE, spread=FALSE, boxplots=FALSE, span=0.5, ellipse=FALSE, levels=c(.5, .9), by.groups=TRUE, data=ff) res2 <- gtrends(c("p-value", "bayes factor"))
library(plotrix) .likelihoodShiftedT <- function(par, data) { -sum(log(dt((data - par[1])/par[2], par[3])/par[2])) } .dposteriorShiftedT <- function(x, parameters, oneSided) { # function that returns the posterior density if (oneSided == FALSE) { dt((x - parameters[1])/parameters[2], parameters[3])/parameters[2] } else if (oneSided == "right") { ifelse(x >= 0, (dt((x - parameters[1])/parameters[2], parameters[3])/parameters[2])/pt((0 - parameters[1])/parameters[2], parameters[3], lower.tail = FALSE), 0) } else if (oneSided == "left") { ifelse(x <= 0, (dt((x - parameters[1])/parameters[2], parameters[3])/parameters[2])/pt((0 - parameters[1])/parameters[2], parameters[3], lower.tail = TRUE), 0) } } .dprior <- function(x, r, oneSided = oneSided) { # function that returns the prior density if (oneSided == "right") { y <- ifelse(x < 0, 0, 2/(pi * r * (1 + (x/r)^2))) return(y) } if (oneSided == "left") { y <- ifelse(x > 0, 0, 2/(pi * r * (1 + (x/r)^2))) return(y) } else { return(1/(pi * r * (1 + (x/r)^2))) } } .plotPosterior.ttest <- function(x = NULL, y = NULL, paired = FALSE, oneSided = FALSE, BF, BFH1H0 = TRUE, iterations = 10000, rscale = "medium", lwd = 2, cexPoints = 1.5, cexAxis = 1.2, cexYlab = 1.5, cexXlab = 1.5, cexTextBF = 1.4, cexCI = 1.1, cexLegend = 1.2, lwdAxis = 1.2, addInformation = TRUE, dontPlotData = FALSE) { if (addInformation) { par(mar = c(5.6, 5, 7, 4) + 0.1, las = 1) } else { par(mar = c(5.6, 5, 4, 4) + 0.1, las = 1) } if (dontPlotData) { plot(1, type = "n", xlim = 0:1, ylim = 0:1, bty = "n", axes = FALSE, xlab = "", ylab = "") axis(1, at = 0:1, labels = FALSE, cex.axis = cexAxis, lwd = lwdAxis, xlab = "") axis(2, at = 0:1, labels = FALSE, cex.axis = cexAxis, lwd = lwdAxis, ylab = "") mtext(text = "Density", side = 2, las = 0, cex = cexYlab, line = 3.25) mtext(expression(paste("Effect size", ~delta)), side = 1, cex = cexXlab, line = 2.5) return() } if (rscale == "medium") { r <- sqrt(2)/2 } if (rscale == "wide") { r <- 1 } if (rscale == "ultrawide") { r <- sqrt(2) } if (mode(rscale) == "numeric") { r <- rscale } if (oneSided == FALSE) { nullInterval <- NULL } if (oneSided == "right") { nullInterval <- c(0, Inf) } if (oneSided == "left") { nullInterval <- c(-Inf, 0) } # sample from delta posterior samples <- BayesFactor::ttestBF(x = x, y = y, paired = paired, posterior = TRUE, iterations = iterations, rscale = r) delta <- samples[, "delta"] # fit shifted t distribution if (is.null(y)) { deltaHat <- mean(x)/sd(x) N <- length(x) df <- N - 1 sigmaStart <- 1/N } else if (paired) { deltaHat <- mean(x - y)/sd(x - y) N <- length(x) df <- N - 1 sigmaStart <- 1/N } else if (!is.null(y) && !paired) { N1 <- length(x) N2 <- length(y) sdPooled <- sqrt(((N1 - 1) * var(x) + (N2 - 1) * var(y))/(N1 + N2)) deltaHat <- (mean(x) - mean(y))/sdPooled df <- N1 + N2 - 2 sigmaStart <- sqrt(N1 * N2/(N1 + N2)) } if (sigmaStart < 0.01) sigmaStart <- 0.01 parameters <- try(silent = TRUE, expr = optim(par = c(deltaHat, sigmaStart, df), fn = .likelihoodShiftedT, data = delta, method = "BFGS")$par) if (class(parameters) == "try-error") { parameters <- try(silent = TRUE, expr = optim(par = c(deltaHat, sigmaStart, df), fn = .likelihoodShiftedT, data = delta, method = "Nelder-Mead")$par) } if (BFH1H0) { BF10 <- BF BF01 <- 1/BF10 } else { BF01 <- BF BF10 <- 1/BF01 } # set limits plot xlim <- vector("numeric", 2) if (oneSided == FALSE) { xlim[1] <- min(-2, quantile(delta, probs = 0.01)[[1]]) xlim[2] <- max(2, quantile(delta, probs = 0.99)[[1]]) if (length(x) < 10) { if (addInformation) { stretch <- 1.52 } else { stretch <- 1.4 } } else { stretch <- 1.2 } } if (oneSided == "right") { # if (length(delta[delta >= 0]) < 10) return('Plotting is not # possible: To few posterior samples in tested interval') xlim[1] <- min(-2, quantile(delta[delta >= 0], probs = 0.01)[[1]]) xlim[2] <- max(2, quantile(delta[delta >= 0], probs = 0.99)[[1]]) if (any(is.na(xlim))) { xlim[1] <- min(-2, .qShiftedT(0.01, parameters, oneSided = "right")) xlim[2] <- max(2, .qShiftedT(0.99, parameters, oneSided = "right")) } stretch <- 1.32 } if (oneSided == "left") { # if (length(delta[delta <= 0]) < 10) return('Plotting is not # possible: To few posterior samples in tested interval') xlim[1] <- min(-2, quantile(delta[delta <= 0], probs = 0.01)[[1]]) xlim[2] <- max(2, quantile(delta[delta <= 0], probs = 0.99)[[1]]) if (any(is.na(xlim))) { xlim[1] <- min(-2, .qShiftedT(0.01, parameters, oneSided = "left")) xlim[2] <- max(2, .qShiftedT(0.99, parameters, oneSided = "left")) } stretch <- 1.32 } xticks <- pretty(xlim) ylim <- vector("numeric", 2) ylim[1] <- 0 dmax <- optimize(function(x) .dposteriorShiftedT(x, parameters = parameters, oneSided = oneSided), interval = range(xticks), maximum = TRUE)$objective ylim[2] <- max(stretch * .dprior(0, r, oneSided = oneSided), stretch * dmax) # get maximum density # calculate position of 'nice' tick marks and create labels yticks <- pretty(ylim) xlabels <- formatC(xticks, 1, format = "f") ylabels <- formatC(yticks, 1, format = "f") # compute 95% credible interval & median: if (oneSided == FALSE) { CIlow <- quantile(delta, probs = 0.025)[[1]] CIhigh <- quantile(delta, probs = 0.975)[[1]] medianPosterior <- median(delta) if (any(is.na(c(CIlow, CIhigh, medianPosterior)))) { CIlow <- .qShiftedT(0.025, parameters, oneSided = FALSE) CIhigh <- .qShiftedT(0.975, parameters, oneSided = FALSE) medianPosterior <- .qShiftedT(0.5, parameters, oneSided = FALSE) } } if (oneSided == "right") { CIlow <- quantile(delta[delta >= 0], probs = 0.025)[[1]] CIhigh <- quantile(delta[delta >= 0], probs = 0.975)[[1]] medianPosterior <- median(delta[delta >= 0]) if (any(is.na(c(CIlow, CIhigh, medianPosterior)))) { CIlow <- .qShiftedT(0.025, parameters, oneSided = "right") CIhigh <- .qShiftedT(0.975, parameters, oneSided = "right") medianPosterior <- .qShiftedT(0.5, parameters, oneSided = "right") } } if (oneSided == "left") { CIlow <- quantile(delta[delta <= 0], probs = 0.025)[[1]] CIhigh <- quantile(delta[delta <= 0], probs = 0.975)[[1]] medianPosterior <- median(delta[delta <= 0]) if (any(is.na(c(CIlow, CIhigh, medianPosterior)))) { CIlow <- .qShiftedT(0.025, parameters, oneSided = "left") CIhigh <- .qShiftedT(0.975, parameters, oneSided = "left") medianPosterior <- .qShiftedT(0.5, parameters, oneSided = "left") } } posteriorLine <- .dposteriorShiftedT(x = seq(min(xticks), max(xticks), length.out = 1000), parameters = parameters, oneSided = oneSided) xlim <- c(min(CIlow, range(xticks)[1]), max(range(xticks)[2], CIhigh)) plot(1, 1, xlim = xlim, ylim = range(yticks), ylab = "", xlab = "", type = "n", axes = FALSE) lines(seq(min(xticks), max(xticks), length.out = 1000), posteriorLine, lwd = lwd) lines(seq(min(xticks), max(xticks), length.out = 1000), .dprior(seq(min(xticks), max(xticks), length.out = 1000), r = r, oneSided = oneSided), lwd = lwd, lty = 3) axis(1, at = xticks, labels = xlabels, cex.axis = cexAxis, lwd = lwdAxis) axis(2, at = yticks, labels = ylabels, , cex.axis = cexAxis, lwd = lwdAxis) if (nchar(ylabels[length(ylabels)]) > 4) { mtext(text = "Density", side = 2, las = 0, cex = cexYlab, line = 4) } else if (nchar(ylabels[length(ylabels)]) == 4) { mtext(text = "Density", side = 2, las = 0, cex = cexYlab, line = 3.25) } else if (nchar(ylabels[length(ylabels)]) < 4) { mtext(text = "Density", side = 2, las = 0, cex = cexYlab, line = 2.85) } mtext(expression(paste("Effect size", ~delta)), side = 1, cex = cexXlab, line = 2.5) points(0, .dprior(0, r, oneSided = oneSided), col = "black", pch = 21, bg = "grey", cex = cexPoints) if (oneSided == FALSE) { heightPosteriorAtZero <- .dposteriorShiftedT(0, parameters = parameters, oneSided = oneSided) } else if (oneSided == "right") { posteriorLineLargerZero <- posteriorLine[posteriorLine > 0] heightPosteriorAtZero <- posteriorLineLargerZero[1] } else if (oneSided == "left") { posteriorLineLargerZero <- posteriorLine[posteriorLine > 0] heightPosteriorAtZero <- posteriorLineLargerZero[length(posteriorLineLargerZero)] } points(0, heightPosteriorAtZero, col = "black", pch = 21, bg = "grey", cex = cexPoints) ### 95% credible interval # enable plotting in margin par(xpd = TRUE) yCI <- grconvertY(dmax, "user", "ndc") + 0.04 yCI <- grconvertY(yCI, "ndc", "user") arrows(CIlow, yCI, CIhigh, yCI, angle = 90, code = 3, length = 0.1, lwd = lwd) medianText <- formatC(medianPosterior, digits = 3, format = "f") if (addInformation) { # display BF10 value offsetTopPart <- 0.06 yy <- grconvertY(0.75 + offsetTopPart, "ndc", "user") yy2 <- grconvertY(0.806 + offsetTopPart, "ndc", "user") xx <- min(xticks) if (BF10 >= 1000000 | BF01 >= 1000000) { BF10t <- formatC(BF10, 3, format = "e") BF01t <- formatC(BF01, 3, format = "e") } if (BF10 < 1000000 & BF01 < 1000000) { BF10t <- formatC(BF10, 3, format = "f") BF01t <- formatC(BF01, 3, format = "f") } if (oneSided == FALSE) { text(xx, yy2, bquote(BF[10] == .(BF10t)), cex = cexTextBF, pos = 4) text(xx, yy, bquote(BF[0][1] == .(BF01t)), cex = cexTextBF, pos = 4) } if (oneSided == "right") { text(xx, yy2, bquote(BF["+"][0] == .(BF10t)), cex = cexTextBF, pos = 4) text(xx, yy, bquote(BF[0]["+"] == .(BF01t)), cex = cexTextBF, pos = 4) } if (oneSided == "left") { text(xx, yy2, bquote(BF["-"][0] == .(BF10t)), cex = cexTextBF, pos = 4) text(xx, yy, bquote(BF[0]["-"] == .(BF01t)), cex = cexTextBF, pos = 4) } yy <- grconvertY(0.756 + offsetTopPart, "ndc", "user") yy2 <- grconvertY(0.812 + offsetTopPart, "ndc", "user") CIText <- paste("95% CI: [", bquote(.(formatC(CIlow, 3, format = "f"))), ", ", bquote(.(formatC(CIhigh, 3, format = "f"))), "]", sep = "") medianLegendText <- paste("median =", medianText) text(max(xticks), yy2, medianLegendText, cex = 1.1, pos = 2) text(max(xticks), yy, CIText, cex = 1.1, pos = 2) # probability wheel if (max(nchar(BF10t), nchar(BF01t)) <= 4) { xx <- grconvertX(0.44, "ndc", "user") } if (max(nchar(BF10t), nchar(BF01t)) == 5) { xx <- grconvertX(0.44 + 0.001 * 5, "ndc", "user") } if (max(nchar(BF10t), nchar(BF01t)) == 6) { xx <- grconvertX(0.44 + 0.001 * 6, "ndc", "user") } if (max(nchar(BF10t), nchar(BF01t)) == 7) { xx <- grconvertX(0.44 + 0.002 * max(nchar(BF10t), nchar(BF01t)), "ndc", "user") } if (max(nchar(BF10t), nchar(BF01t)) == 8) { xx <- grconvertX(0.44 + 0.003 * max(nchar(BF10t), nchar(BF01t)), "ndc", "user") } if (max(nchar(BF10t), nchar(BF01t)) > 8) { xx <- grconvertX(0.44 + 0.005 * max(nchar(BF10t), nchar(BF01t)), "ndc", "user") } yy <- grconvertY(0.788 + offsetTopPart, "ndc", "user") # make sure that colored area is centered radius <- 0.06 * diff(range(xticks)) A <- radius^2 * pi alpha <- 2/(BF01 + 1) * A/radius^2 startpos <- pi/2 - alpha/2 # draw probability wheel plotrix::floating.pie(xx, yy, c(BF10, 1), radius = radius, col = c("darkred", "white"), lwd = 2, startpos = startpos) yy <- grconvertY(0.865 + offsetTopPart, "ndc", "user") yy2 <- grconvertY(0.708 + offsetTopPart, "ndc", "user") if (oneSided == FALSE) { text(xx, yy, "data|H1", cex = cexCI) text(xx, yy2, "data|H0", cex = cexCI) } if (oneSided == "right") { text(xx, yy, "data|H+", cex = cexCI) text(xx, yy2, "data|H0", cex = cexCI) } if (oneSided == "left") { text(xx, yy, "data|H-", cex = cexCI) text(xx, yy2, "data|H0", cex = cexCI) } # add legend CIText <- paste("95% CI: [", bquote(.(formatC(CIlow, 3, format = "f"))), " ; ", bquote(.(formatC(CIhigh, 3, format = "f"))), "]", sep = "") medianLegendText <- paste("median =", medianText) } mostPosterior <- mean(delta > mean(range(xticks))) if (mostPosterior >= 0.5) { legendPosition <- min(xticks) legend(legendPosition, max(yticks), legend = c("Posterior", "Prior"), lty = c(1, 3), bty = "n", lwd = c(lwd, lwd), cex = cexLegend, xjust = 0, yjust = 1, x.intersp = 0.6, seg.len = 1.2) } else { legendPosition <- max(xticks) legend(legendPosition, max(yticks), legend = c("Posterior", "Prior"), lty = c(1, 3), bty = "n", lwd = c(lwd, lwd), cex = cexLegend, xjust = 1, yjust = 1, x.intersp = 0.6, seg.len = 1.2) } } ### generate data ### set.seed(1) x <- rnorm(30, 0.15) ### calculate Bayes factor ### library(BayesFactor) BF <- extractBF(ttestBF(x, rscale = "medium"), onlybf = TRUE) ### plot ### .plotPosterior.ttest(x = x, rscale = "medium", BF = BF)
#monte carlo t test simulation #install.packages("MonteCarlo") library(MonteCarlo) # Define function that generates data and applies the method of interest ttest<-function(n,loc,scale){ # generate sample: sample<-rnorm(n, loc, scale) # calculate test statistic: stat<-sqrt(n)*mean(sample)/sd(sample) # get test decision: decision<-abs(stat)>1.96 # return result: return(list("decision"=decision)) } # define parameter grid: n_grid<-c(50,100,250,500) loc_grid<-seq(0,1,0.2) scale_grid<-c(1,2) # collect parameter grids in list: param_list=list("n"=n_grid, "loc"=loc_grid, "scale"=scale_grid) # run simulation: MC_result<-MonteCarlo(func=ttest, nrep=1000, param_list=param_list) summary(MC_result)
#install.packages("RWordPress", repos="http://www.omegahat.org/R", build=TRUE) library(RWordPress) options(WordPressLogin=c(user="password"), WordPressURL="http://your_wp_installation.org/xmlrpc.php") #[code lang='r'] #... #[/code] knit_hooks$set(output=function(x, options) paste("\\[code\\]\n", x, "\\[/code\\]\n", sep="")) knit_hooks$set(source=function(x, options) paste("\\[code lang='r'\\]\n", x, "\\[/code\\]\n", sep="")) knit2wp <- function(file) { require(XML) content <- readLines(file) content <- htmlTreeParse(content, trim=FALSE) ## WP will add the h1 header later based on the title, so delete here content$children$html$children$body$children$h1 <- NULL content <- paste(capture.output(print(content$children$html$children$body, indent=FALSE, tagSeparator="")), collapse="\n") content <- gsub("<?.body>", "", content) # remove body tag ## enclose code snippets in SyntaxHighlighter format content <- gsub("<?pre><code class=\"r\">", "\\[code lang='r'\\]\\\n", content) content <- gsub("<?pre><code class=\"no-highlight\">", "\\[code\\]\\\n", content) content <- gsub("<?pre><code>", "\\[code\\]\\\n", content) content <- gsub("<?/code></pre>", "\\[/code\\]\\\n", content) return(content) } newPost(content=list(description=knit2wp('rerWorkflow.html'), title='Workflow: Post R markdown to WordPress', categories=c('R')), publish=FALSE) postID <- 99 # post id returned by newPost() editPost(postID, content=list(description=knit2wp('rerWorkflow.html'), title='Workflow: Post R markdown to WordPress', categories=c('R')), publish=FALSE)